Horizon auto-picking with quantitative uncertainty evaluation by using a modified VQ-VAE framework
JOURNAL OF GEOPHYSICS AND ENGINEERING(2022)
摘要
In this paper, we propose a novel approach of deep-learning-based seismic horizon auto-picking that introduces a modified vector quantized variational autoencoder (VQ-VAE) framework to improve the accuracy of seismic horizon interpretation and, for the first time, quantitatively evaluate the uncertainty of the auto-picked horizon by exploiting the concept of entropy. Compared with the conventional VQ-VAE approach, the proposed method not only modifies the VQ-VAE model with more deep-learning channels at each layer of the network to enhance the performance of horizon auto-picking within the VQ-VAE framework, but also extends the 1D seismic labels with more continuous samplings within a single trace to boost the stability of auto-picked horizon in geologically complex settings and also significantly suppress the resulting uncertainty. To further improve the resulting accuracy in geologically complex settings, we introduce the directional structure tensor to extract a more reliable initial horizon and, moreover, a dilated horizon searching strategy to extend the capacity of the proposed method in dealing with the large fault displacement and reducing the computational cost simultaneously. Additionally, the resulting uncertainty quantitatively measured by entropy can also serve as an effective indicator to enable a further refinement of the auto-picked result accordingly. Both 2D example and 3D field applications are carried out to validate the effectiveness of the proposed method.
更多查看译文
关键词
horizon auto-picking, quantitative uncertainty evaluation, VQ-VAE framework, directional structure tensor, dilated horizon searching strategy
AI 理解论文
溯源树
样例
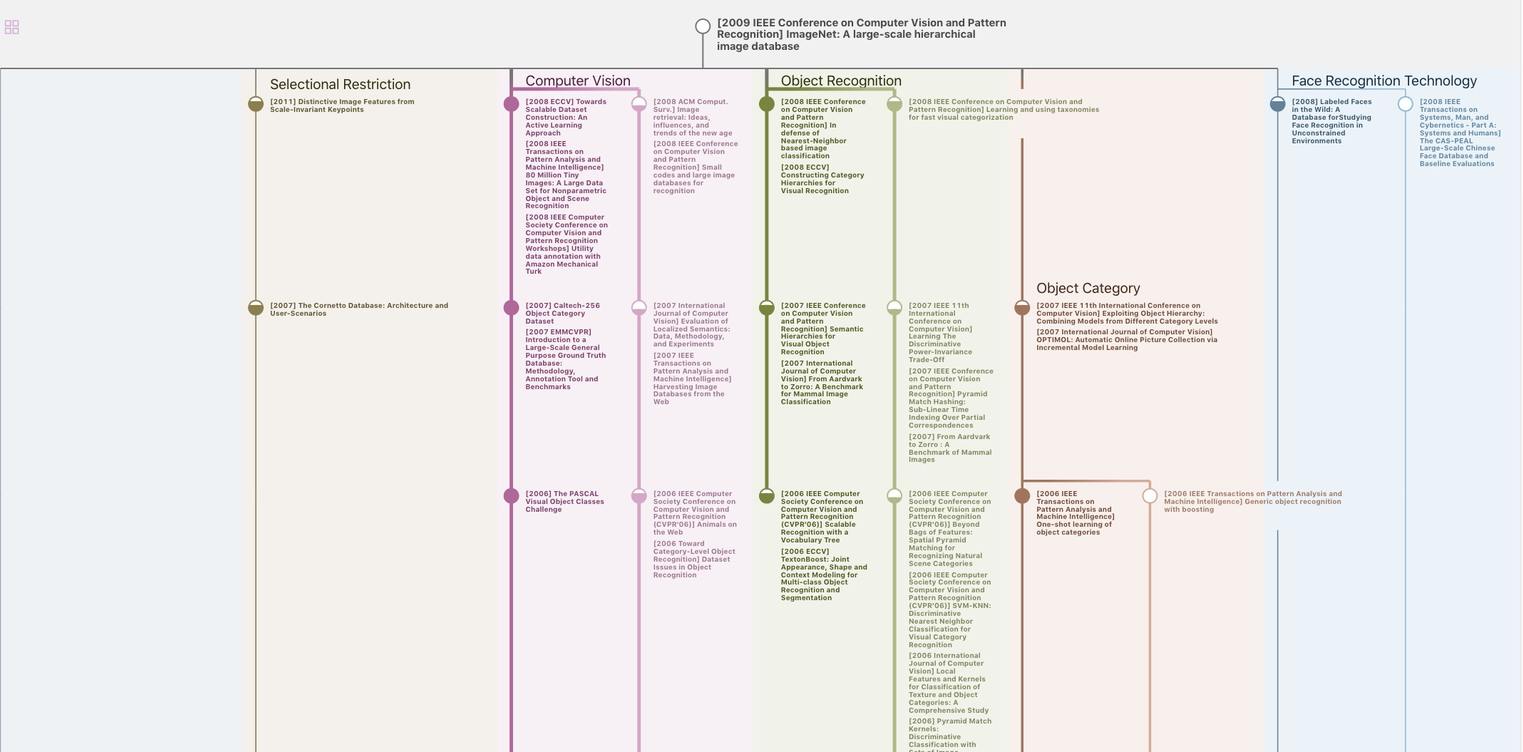
生成溯源树,研究论文发展脉络
Chat Paper
正在生成论文摘要