SUPER-Rec: SUrrounding Position-Enhanced Representation for Recommendation
arXiv (Cornell University)(2022)
摘要
Collaborative filtering problems are commonly solved based on matrix completion techniques which recover the missing values of user-item interaction matrices. In a matrix, the rating position specifically represents the user given and the item rated. Previous matrix completion techniques tend to neglect the position of each element (user, item and ratings) in the matrix but mainly focus on semantic similarity between users and items to predict the missing value in a matrix. This paper proposes a novel position-enhanced user/item representation training model for recommendation, SUPER-Rec. We first capture the rating position in the matrix using the relative positional rating encoding and store the position-enhanced rating information and its user-item relationship to the fixed dimension of embedding that is not affected by the matrix size. Then, we apply the trained position-enhanced user and item representations to the simplest traditional machine learning models to highlight the pure novelty of our representation learning model. We contribute the first formal introduction and quantitative analysis of position-enhanced item representation in the recommendation domain and produce a principled discussion about our SUPER-Rec to the outperformed performance of typical collaborative filtering recommendation tasks with both explicit and implicit feedback.
更多查看译文
关键词
representation,super-rec,position-enhanced
AI 理解论文
溯源树
样例
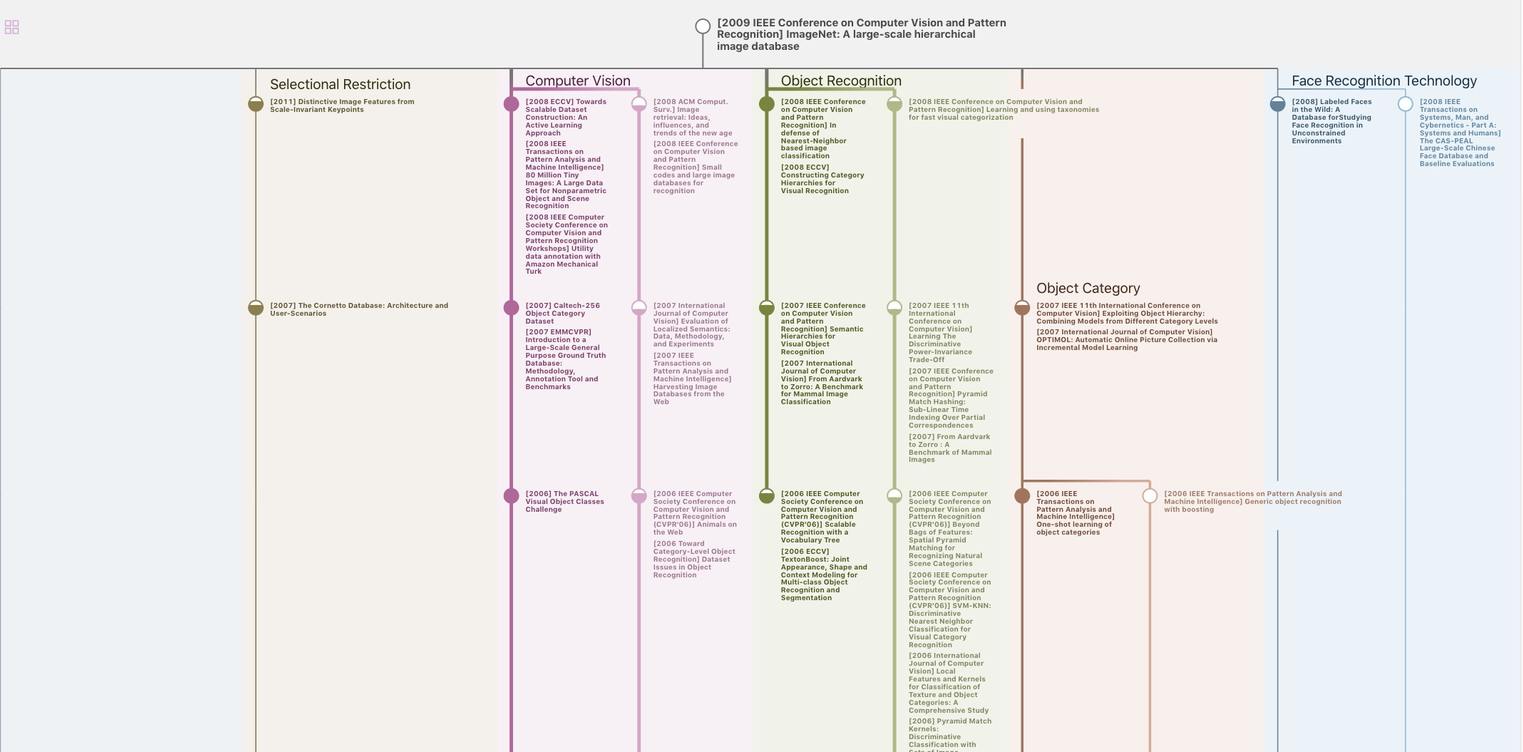
生成溯源树,研究论文发展脉络
Chat Paper
正在生成论文摘要