Heart Sound Classification based on Residual Shrinkage Networks.
Annual International Conference of the IEEE Engineering in Medicine and Biology Society (EMBC)(2022)
摘要
Heart sound classification is one of the non-invasive methods for early detection of the cardiovascular diseases (CVDs), the leading cause for deaths. In recent years, Computer Audition (CA) technology has become increasingly sophisticated, auxiliary diagnosis technology of heart disease based on CA has become a popular research area. This paper proposes a deep Convolutional Neural Network (CNN) model for heart sound classification. To improve the classification accuracy of heart sound, we design a classification algorithm combining classical Residual Network (ResNet) and Long Short-Term Memory (LSTM). The model performance is evaluated in the PhysioNet/CinC Challenges 2016 datasets using a 2D time-frequency feature. We extract the four features from different filter-bank coefficients, including Filterbank (Fbank), Mel-Frequency Spectral Coefficients (MFSCs), and Mel-Frequency Cepstral Coefficients (MFCCs). The experimental results show the MFSCs feature outperforms the other features in the proposed CNN model. The proposed model performs well on the test set, particularly the F1 score of 84.3 % - the accuracy of 84.4 %, the sensitivity of 84.3 %, and the specificity of 85.6 %. Compared with the classical ResNet model, an accuracy of 4.9 % improvement is observed in the proposed model.
更多查看译文
关键词
Algorithms,Disease Progression,Hearing,Heart Sounds,Humans,Neural Networks, Computer
AI 理解论文
溯源树
样例
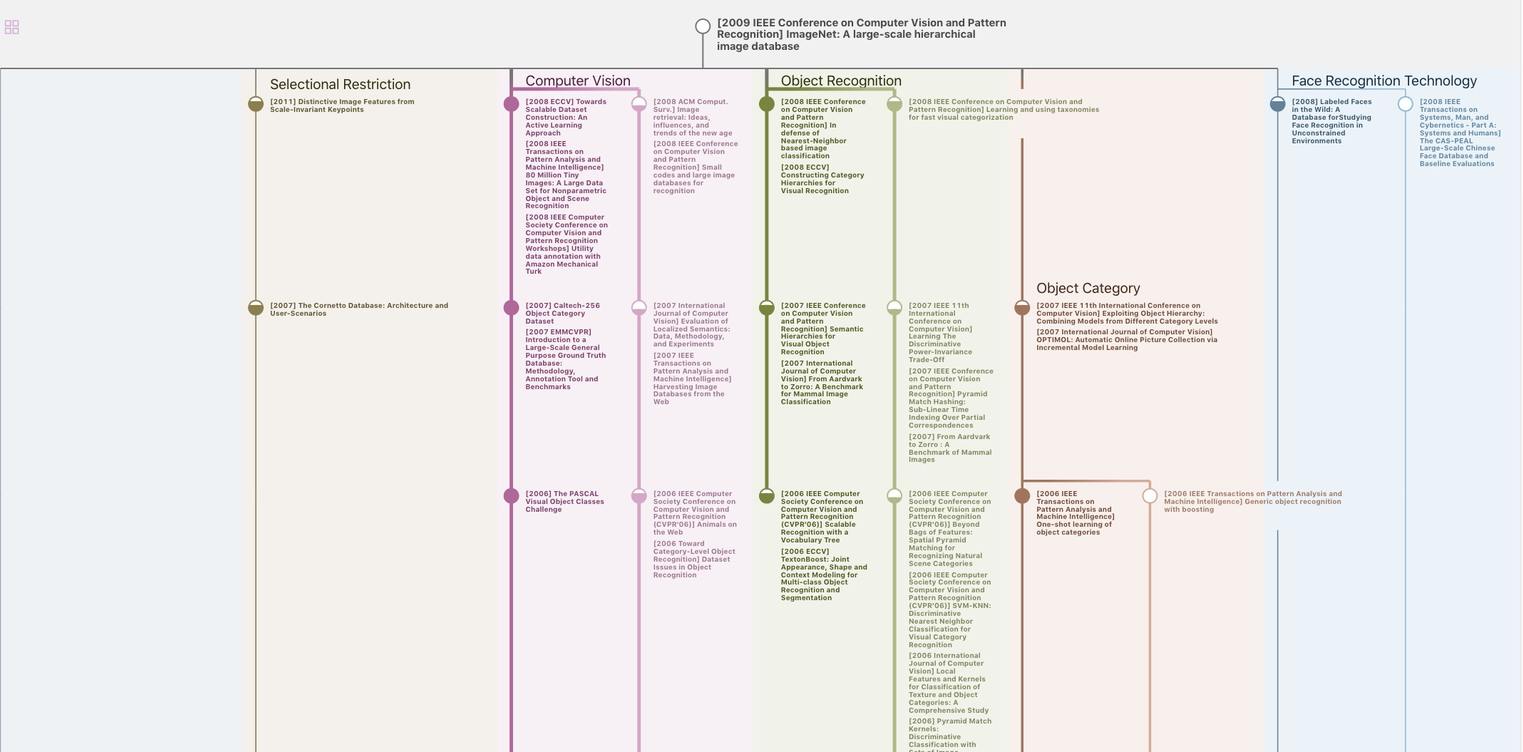
生成溯源树,研究论文发展脉络
Chat Paper
正在生成论文摘要