SiSL-Net: Saliency-guided Self-Supervised Learning Network for Image Classification
Neurocomputing(2022)
摘要
The emergent studies and success on contrastive self-supervised learning have been well verified in the pretext task of instance discrimination, which learns visual representations by maximizing agreement between different augmented views of the same image sample (positive pairs). However, randomly crop-ping on original images may cause that the augmented view contains interference from a large propor-tion of the backgrounds, referred to as noisy data. Aiming to optimize the data augmentation and improve positive pairs, a Saliency-Augmented Module is proposed to obtain the augmented views, which only contain the "latent" object area, referred to as clean data. Furthermore, a Saliency-Guided Self -Supervised Learning Network (SiSL-Net) is constructed as a new pattern of contrastive learning. A sym-metric structure of trunk net and branch net is trained to learn a feature mapping from the clean data space and the noisy data space. Besides, a novel loss function is designed, including the embedding con-trastive loss and distribution consistency loss, to optimize the feature representations during network training. The linear classification performance of our SiSL-Net is evaluated on the miniImageNet dataset with ResNet-50. Experiments show that our method achieves the top-1 accuracy from 64.67% to 69.02%, outperforming the state-of-the-art performance. (c) 2022 Published by Elsevier B.V.
更多查看译文
关键词
Positive pairs,Contrastive self-supervised learning,Saliency detection
AI 理解论文
溯源树
样例
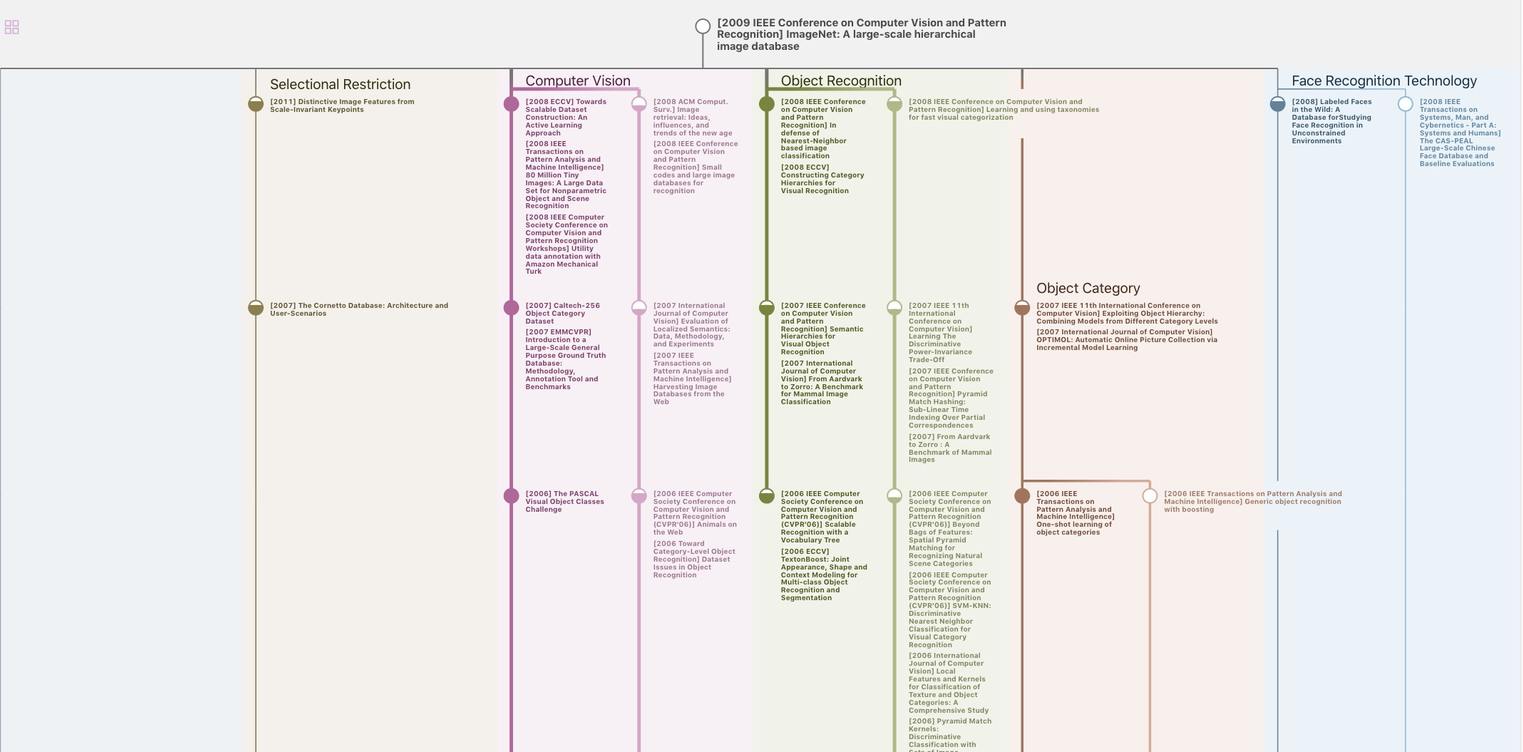
生成溯源树,研究论文发展脉络
Chat Paper
正在生成论文摘要