Randomized Privacy Budget Differential Privacy
arxiv(2022)
摘要
While pursuing better utility by discovering knowledge from the data, individual's privacy may be compromised during an analysis. To that end, differential privacy has been widely recognized as the state-of-the-art privacy notion. By requiring the presence of any individual's data in the input to only marginally affect the distribution over the output, differential privacy provides strong protection against adversaries in possession of arbitrary background. However, the privacy constraints (e.g., the degree of randomization) imposed by differential privacy may render the released data less useful for analysis, the fundamental trade-off between privacy and utility (i.e., analysis accuracy) has attracted significant attention in various settings. In this report we present DP mechanisms with randomized parameters, i.e., randomized privacy budget, and formally analyze its privacy and utility and demonstrate that randomizing privacy budget in DP mechanisms will boost the accuracy in a humongous scale.
更多查看译文
关键词
privacy
AI 理解论文
溯源树
样例
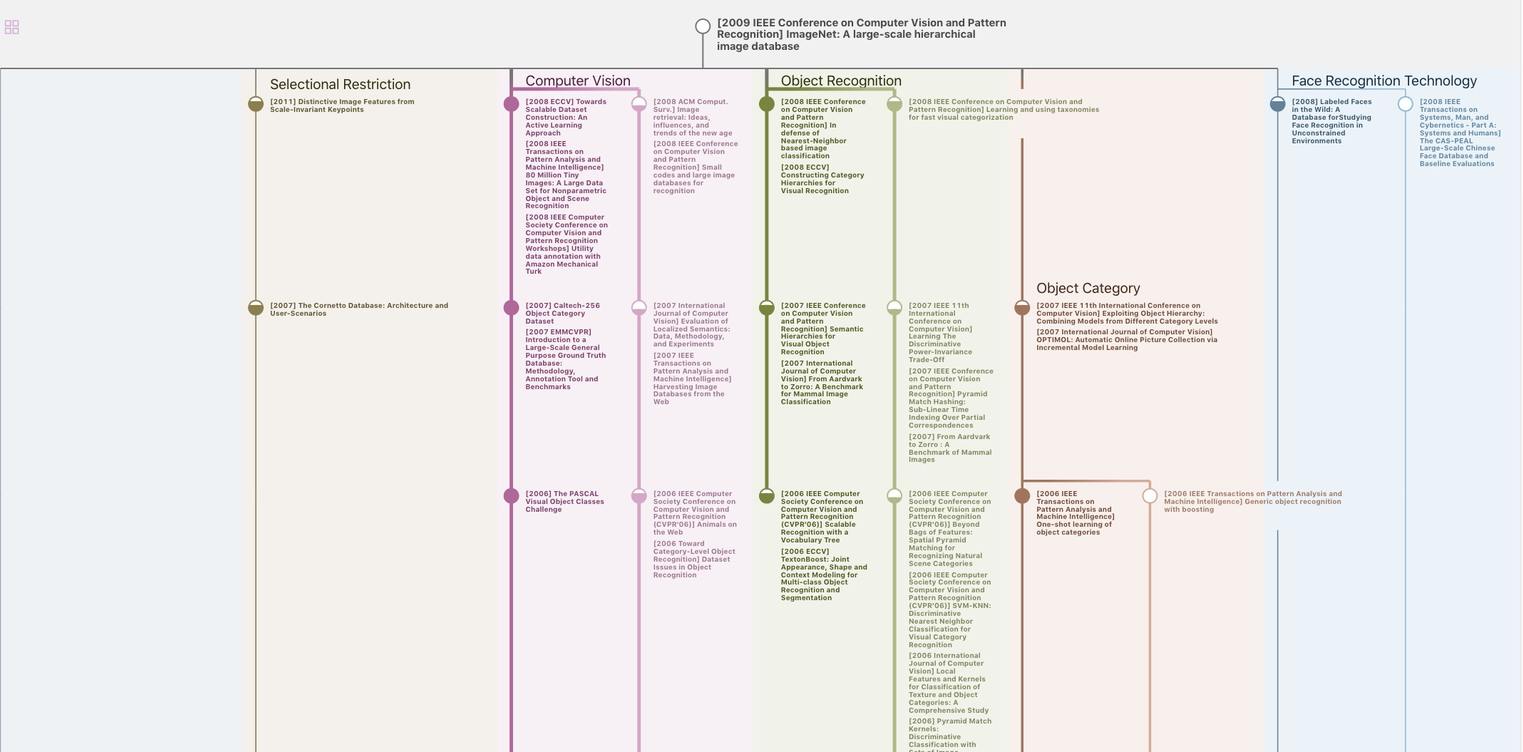
生成溯源树,研究论文发展脉络
Chat Paper
正在生成论文摘要