Voluntary Activation of the Diaphragm after Inspiratory Pressure Threshold Loading
Physiological Reports(2023)
Univ British Columbia | St Pauls Hosp
Abstract
Abstract After a bout of isolated inspiratory work, such as inspiratory pressure threshold loading (IPTL), the human diaphragm can exhibit a reversible loss in contractile function, as evidenced by a decrease in transdiaphragmatic twitch pressure (PDI,TW). Whether or not diaphragm fatigability after IPTL is affected by neural mechanisms, measured through voluntary activation of the diaphragm (D‐VA) in addition to contractile mechanisms, is unknown. It is also unknown if changes in D‐VA are similar between sexes given observed differences in diaphragm fatigability between males and females. We sought to determine whether D‐VA decreases after IPTL and whether this was different between sexes. Healthy females (n = 11) and males (n = 10) completed an IPTL task with an inspired duty cycle of 0.7 and targeting an intensity of 60% maximal transdiaphragmatic pressure until task failure. PDI,TW and D‐VA were measured using cervical magnetic stimulation of the phrenic nerves in combination with maximal inspiratory pressure maneuvers. At task failure, PDI,TW decreased to a lesser degree in females vs. males (87 ± 15 vs. 73 ± 12% baseline, respectively, p = 0.016). D‐VA decreased after IPTL but was not different between females and males (91 ± 8 vs. 88 ± 10% baseline, respectively, p = 0.432). When all participants were pooled together, the decrease in PDI,TW correlated with both the total cumulative diaphragm pressure generation (R2 = 0.43; p = 0.021) and the time to task failure (TTF, R2 = 0.40; p = 0.30) whereas the decrease in D‐VA correlated only with TTF (R2 = 0.24; p = 0.041). Our results suggest that neural mechanisms can contribute to diaphragm fatigability, and this contribution is similar between females and males following IPTL.
MoreTranslated text
Key words
Positive End-Expiratory Pressure
求助PDF
上传PDF
View via Publisher
AI Read Science
AI Summary
AI Summary is the key point extracted automatically understanding the full text of the paper, including the background, methods, results, conclusions, icons and other key content, so that you can get the outline of the paper at a glance.
Example
Background
Key content
Introduction
Methods
Results
Related work
Fund
Key content
- Pretraining has recently greatly promoted the development of natural language processing (NLP)
- We show that M6 outperforms the baselines in multimodal downstream tasks, and the large M6 with 10 parameters can reach a better performance
- We propose a method called M6 that is able to process information of multiple modalities and perform both single-modal and cross-modal understanding and generation
- The model is scaled to large model with 10 billion parameters with sophisticated deployment, and the 10 -parameter M6-large is the largest pretrained model in Chinese
- Experimental results show that our proposed M6 outperforms the baseline in a number of downstream tasks concerning both single modality and multiple modalities We will continue the pretraining of extremely large models by increasing data to explore the limit of its performance
Upload PDF to Generate Summary
Must-Reading Tree
Example
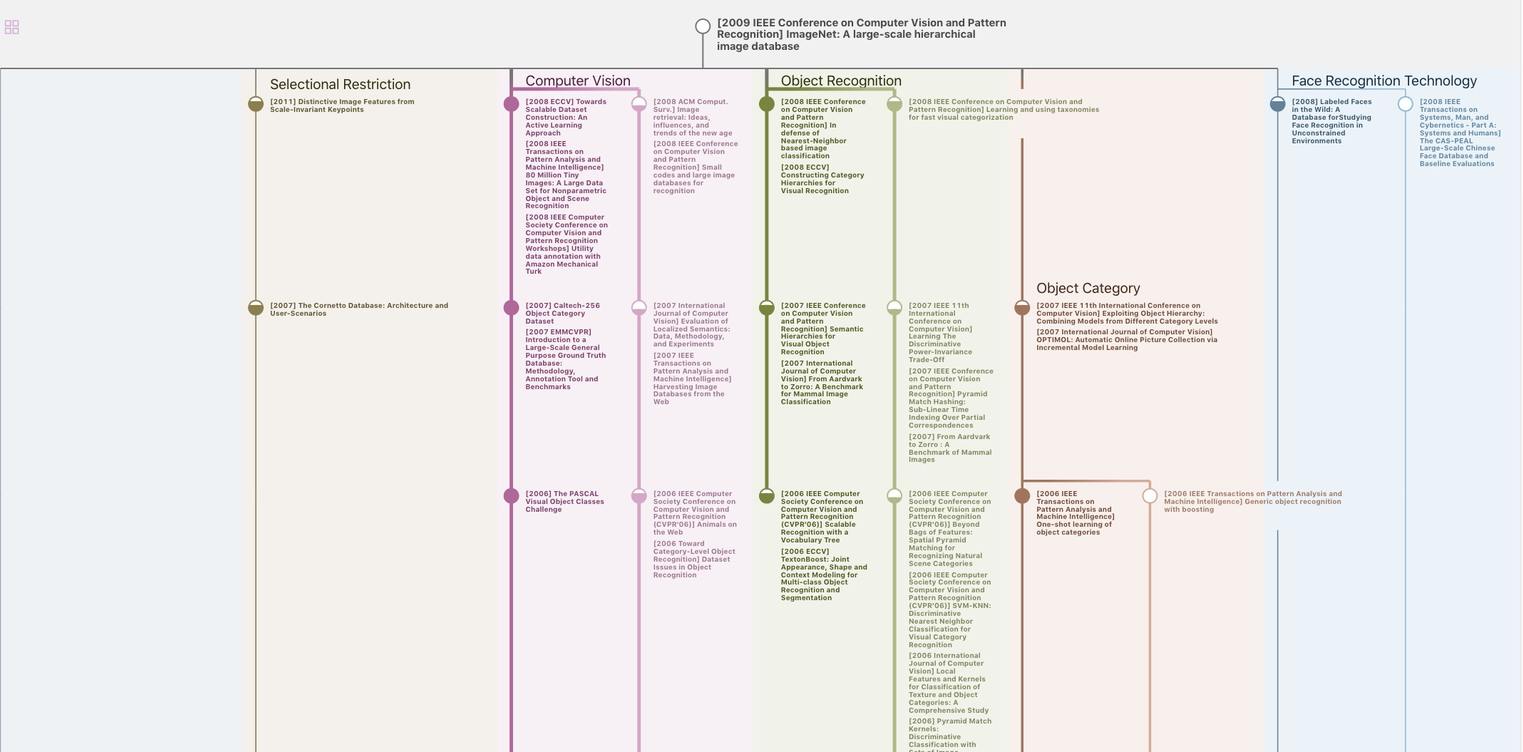
Generate MRT to find the research sequence of this paper
Related Papers
2007
被引用162 | 浏览
2018
被引用37 | 浏览
2018
被引用61 | 浏览
2018
被引用85 | 浏览
2016
被引用49 | 浏览
2019
被引用117 | 浏览
2020
被引用4 | 浏览
2020
被引用16 | 浏览
Data Disclaimer
The page data are from open Internet sources, cooperative publishers and automatic analysis results through AI technology. We do not make any commitments and guarantees for the validity, accuracy, correctness, reliability, completeness and timeliness of the page data. If you have any questions, please contact us by email: report@aminer.cn
Chat Paper