A Class-Aware Representation Refinement Framework for Graph Classification
arXiv (Cornell University)(2022)
摘要
Graph Neural Networks (GNNs) are widely used for graph representationlearning. Despite its prevalence, GNN suffers from two drawbacks in the graphclassification task, the neglect of graph-level relationships, and thegeneralization issue. Each graph is treated separately in GNN messagepassing/graph pooling, and existing methods to address overfitting operate oneach individual graph. This makes the graph representations learnt lesseffective in the downstream classification. In this paper, we propose aClass-Aware Representation rEfinement (CARE) framework for the task of graphclassification. CARE computes simple yet powerful class representations andinjects them to steer the learning of graph representations towards betterclass separability. CARE is a plug-and-play framework that is highly flexibleand able to incorporate arbitrary GNN backbones without significantlyincreasing the computational cost. We also theoretically prove that CARE has abetter generalization upper bound than its GNN backbone throughVapnik-Chervonenkis (VC) dimension analysis. Our extensive experiments with 11well-known GNN backbones on 9 benchmark datasets validate the superiority andeffectiveness of CARE over its GNN counterparts.
更多查看译文
关键词
Graph neural network,Representation learning,Graph classification
AI 理解论文
溯源树
样例
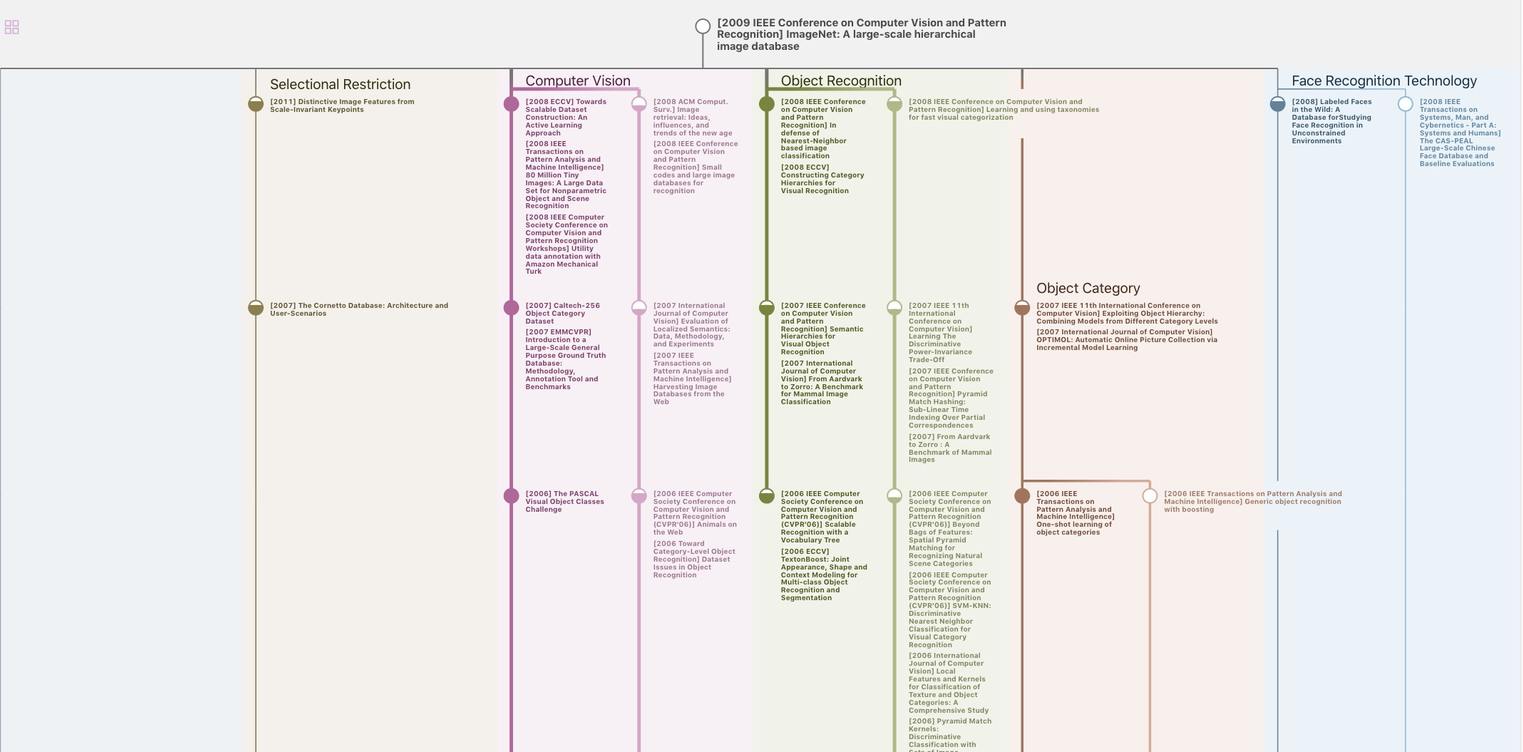
生成溯源树,研究论文发展脉络
Chat Paper
正在生成论文摘要