Empirical Gateaux Derivatives for Causal Inference
NeurIPS 2022(2022)
摘要
We study a constructive algorithm that approximates Gateaux derivatives for statistical functionals by finite-differencing, with a focus on causal inference functionals. We consider the case where probability distributions are not known a priori but also need to be estimated from data. These estimated distributions lead to empirical Gateaux derivatives, and we study the relationships between empirical, numerical, and analytical Gateaux derivatives. Starting with a case study of estimating the mean potential outcome (hence average treatment effect), we instantiate the exact relationship between finite-differences and the analytical Gateaux derivative. We then derive requirements on the rates of numerical approximation in perturbation and smoothing that preserve the statistical benefits of one-step adjustments, such as rate-double-robustness. We then study more complicated functionals such as dynamic treatment regimes and the linear-programming formulation for policy optimization in infinite-horizon Markov decision processes. The newfound ability to approximate bias adjustments in the presence of arbitrary constraints illustrates the usefulness of constructive approaches for Gateaux derivatives. We also find that the statistical structure of the functional (rate-double robustness) can permit less conservative rates of finite-difference approximation. This property, however, can be specific to particular functionals, e.g. it occurs for the mean potential outcome (hence average treatment effect) but not the infinite-horizon MDP policy value.
更多查看译文
关键词
causal inference,double robustness,bias-adjustment,influence function,semiparametric,offline reinforcement learning
AI 理解论文
溯源树
样例
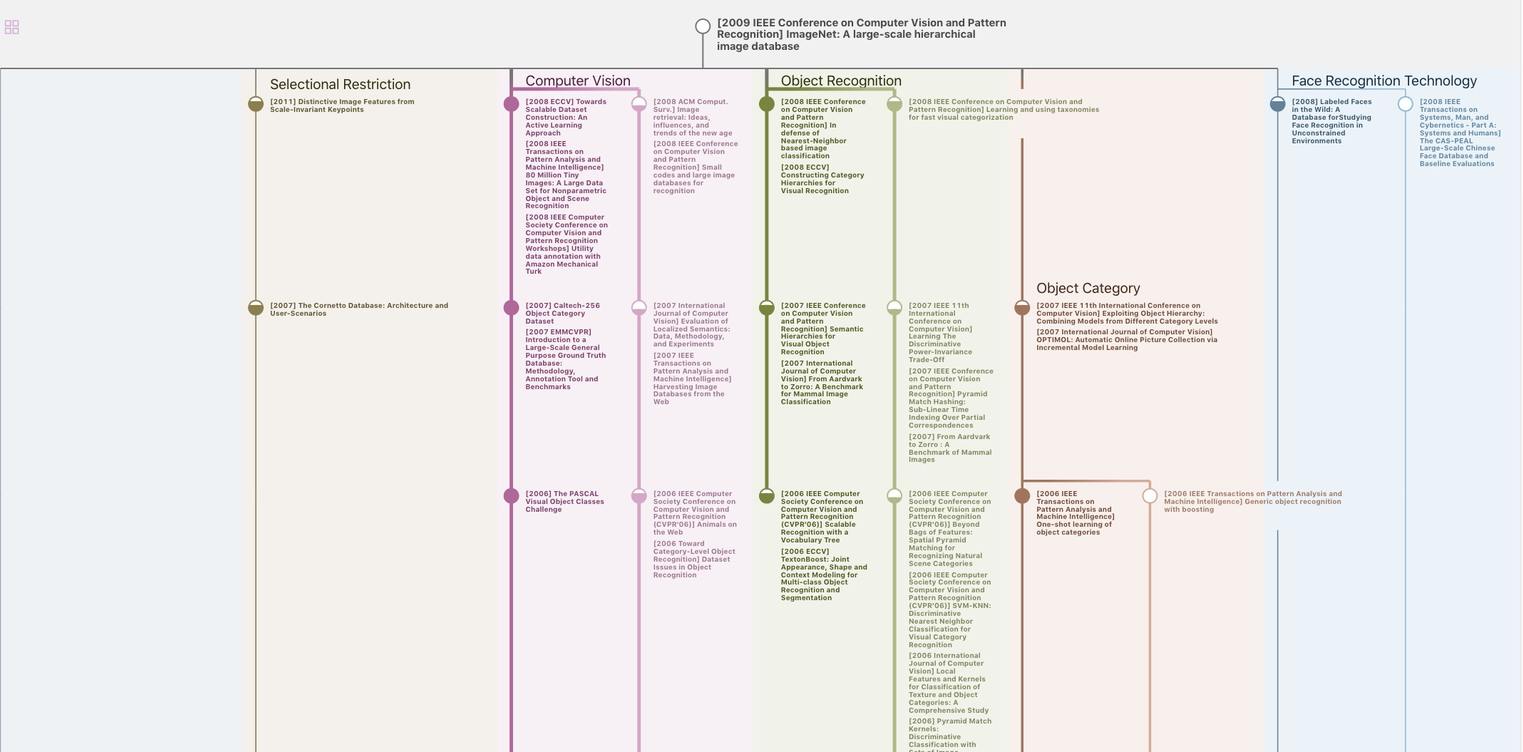
生成溯源树,研究论文发展脉络
Chat Paper
正在生成论文摘要