Multi-Biometric Unified Network for Cloth-Changing Person Re-Identification
IEEE transactions on image processing(2023)
摘要
Person re-identification (re-ID) aims to match the same person across different cameras. However, most existing re-ID methods assume that people wear the same clothes in different views, which limit their performance in identifying target pedestrians who change clothes. Cloth-changing re-ID is a quite challenging problem as clothes occupying a large number of pixels in an image becomes invalid or even misleads information. To tackle this problem, we propose a novel Multi-biometric Unified Network (MBUNet) for learning the robustness of cloth-changing re-ID model by exploiting clothing-independent cues. Specifically, we first introduce a multi-biological feature branch to extract a variety of biological features, such as the head, neck, and shoulders to resist cloth-changing. Then, a differential feature attention module (DFAM) is embedded in this branch, which can extract discriminative fine-grained biological features. Besides, we design a differential recombination on max pooling (DRMP) strategy and simultaneously apply a direction-adaptive graph convolutional layer to mine more robust global and pose features. Finally, we propose a Lightweight Domain Adaptation Module (LDAM) that combines the attention mechanism before and after the waveblock to capture and enhance transferable features across scenarios. To further improve the performance of the model, we also integrate mAP optimization into the objective function of our model for joint training to solve the discrete optimization problem of mAP. Extensive experiments on five cloth-changing re-ID datasets demonstrate the advantages of our proposed MBUNet. The code is available at https://github.com/liyeabc/MBUNet.
更多查看译文
关键词
Person re-identification,cloth-changing,deep learning
AI 理解论文
溯源树
样例
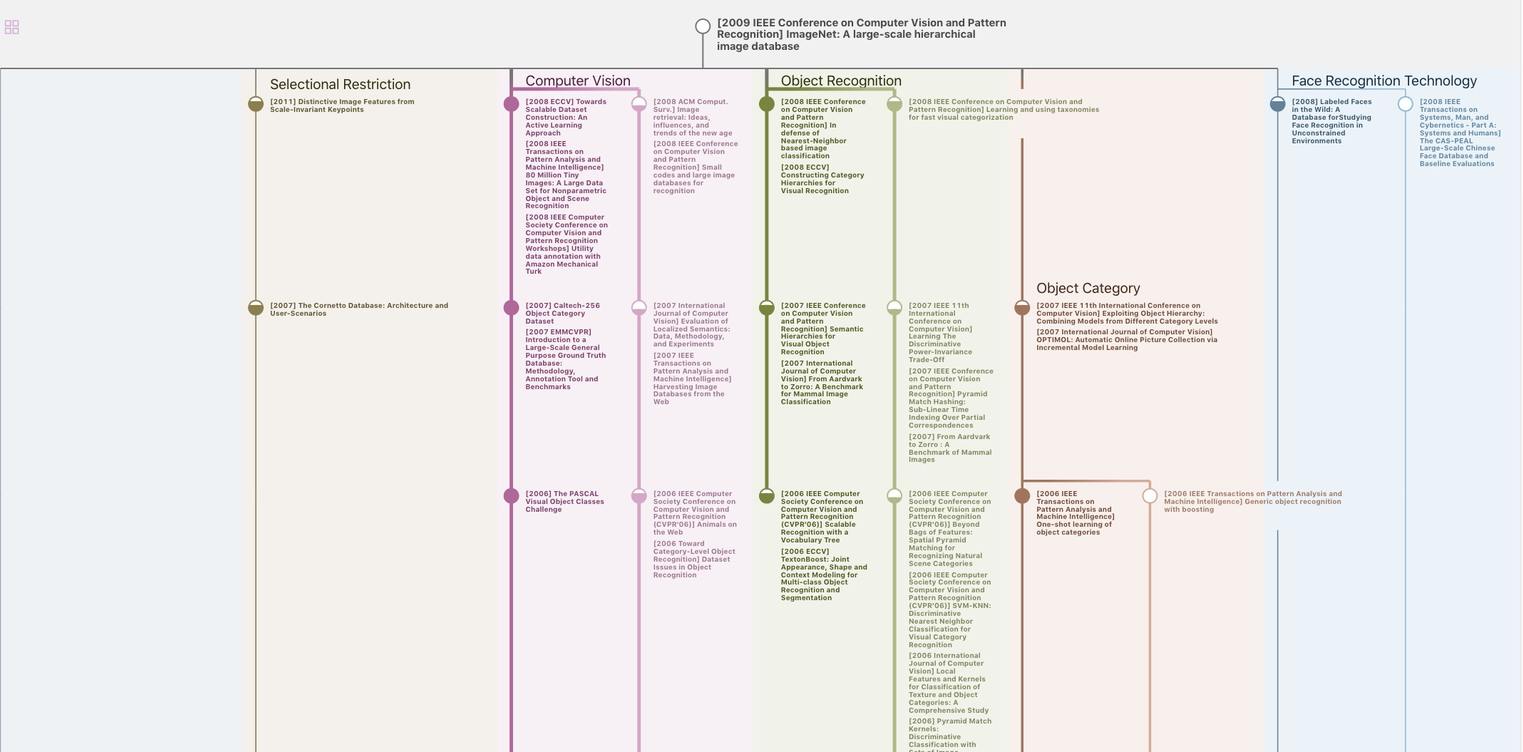
生成溯源树,研究论文发展脉络
Chat Paper
正在生成论文摘要