Quantifying the Privacy-Vs-performance Trade-Offs for Fine-Grained Wireless Network Measurement Data.
ACM International Conference on Applications, Technologies, Architectures, and Protocols for Computer Communication(2022)
摘要
Real-world network measurements are critical to building performant and resilient networks at scale. However, access to such data exposes end-users to significant privacy risks; and this is particularly true for wireless network measurements. In this paper, we apply six state-of-the-art differentially private (DP) algorithms, that span data-independent/dependent and workload-aware/unaware classes, to privatize queries from real-world WiFi traces on a large-scale campus network. We analyze utility-vs-privacy trade-offs involved in constructing privatized queries for canonical network resource provisioning tasks. We present the following results: (1) for count and histogram queries, the utility of the Laplacian-algorithm shows comparable (or better) performance compared to more complex data-aware DP algorithms, (2) for a given query-type and DP algorithm, the utility-to-noise trade-off varies for each distinct network metric, and finally, (3) we implement a state-of-the-art DP algorithm for trajectory analysis that reveals that there exist significant challenges in accurately reconstructing privatized network mobility trajectories, for relatively small trajectory lengths, even with relaxed privacy budgets.
更多查看译文
关键词
Differential Privacy,Data Quality,Location Privacy
AI 理解论文
溯源树
样例
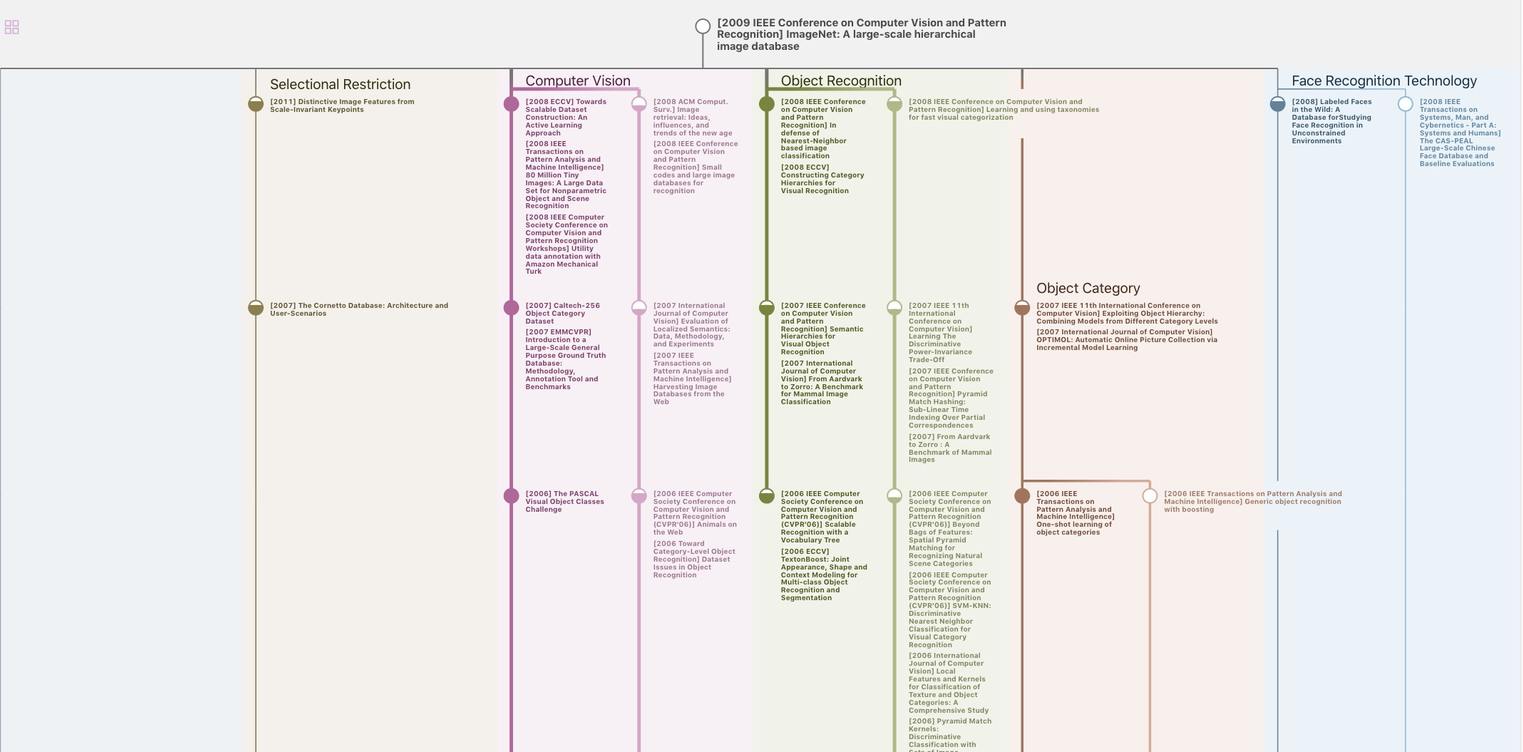
生成溯源树,研究论文发展脉络
Chat Paper
正在生成论文摘要