Deblurring Galaxy Images with Tikhonov Regularization on Magnitude Domain
Publication of the Astronomical Society of Japan(2022)
Natl Astron Observ Japan | Nagoya Univ
Abstract
We propose a regularization-based deblurring method that works efficiently for galaxy images. The spatial resolution of a ground-based telescope is generally limited by seeing conditions and much worse than space-based telescopes. This circumstance has generated considerable research interest in restoration of spatial resolution. Since image deblurring is a typical inverse problem and often ill-posed, solutions tend to be unstable. To obtain a stable solution, much research has adopted regularization-based methods for image deblurring, but the regularization term is not necessarily appropriate for galaxy images. Although galaxies have an exponential or Sersic profile, the conventional regularization assumes the image profiles to behave linear in space. The significant deviation between the assumption and real situation leads to blurring the images and smoothing out the detailed structures. Clearly, regularization on logarithmic, i.e. magnitude domain, should provide a more appropriate assumption, which we explore in this study. We formulate a problem of deblurring galaxy images by an objective function with a Tikhonov regularization term on magnitude domain. We introduce an iterative algorithm minimizing the objective function with a primal-dual splitting method. We investigate the feasibility of the proposed method using simulation and observation images. In the simulation, we blur galaxy images with a realistic point spread function and add both Gaussian and Poisson noises. For the evaluation with the observed images, we use galaxy images taken by the Subaru HSC-SSP. Both of these evaluations show that our method successfully recovers the spatial resolution of the images and significantly outperforms the conventional methods. The code is publicly available from the Github ( https://github.com/kzmurata-astro/PSFdeconv_amag ).
MoreTranslated text
Key words
galaxies: structure,methods: data analysis,techniques: image processing
PDF
View via Publisher
AI Read Science
AI Summary
AI Summary is the key point extracted automatically understanding the full text of the paper, including the background, methods, results, conclusions, icons and other key content, so that you can get the outline of the paper at a glance.
Example
Background
Key content
Introduction
Methods
Results
Related work
Fund
Key content
- Pretraining has recently greatly promoted the development of natural language processing (NLP)
- We show that M6 outperforms the baselines in multimodal downstream tasks, and the large M6 with 10 parameters can reach a better performance
- We propose a method called M6 that is able to process information of multiple modalities and perform both single-modal and cross-modal understanding and generation
- The model is scaled to large model with 10 billion parameters with sophisticated deployment, and the 10 -parameter M6-large is the largest pretrained model in Chinese
- Experimental results show that our proposed M6 outperforms the baseline in a number of downstream tasks concerning both single modality and multiple modalities We will continue the pretraining of extremely large models by increasing data to explore the limit of its performance
Try using models to generate summary,it takes about 60s
Must-Reading Tree
Example
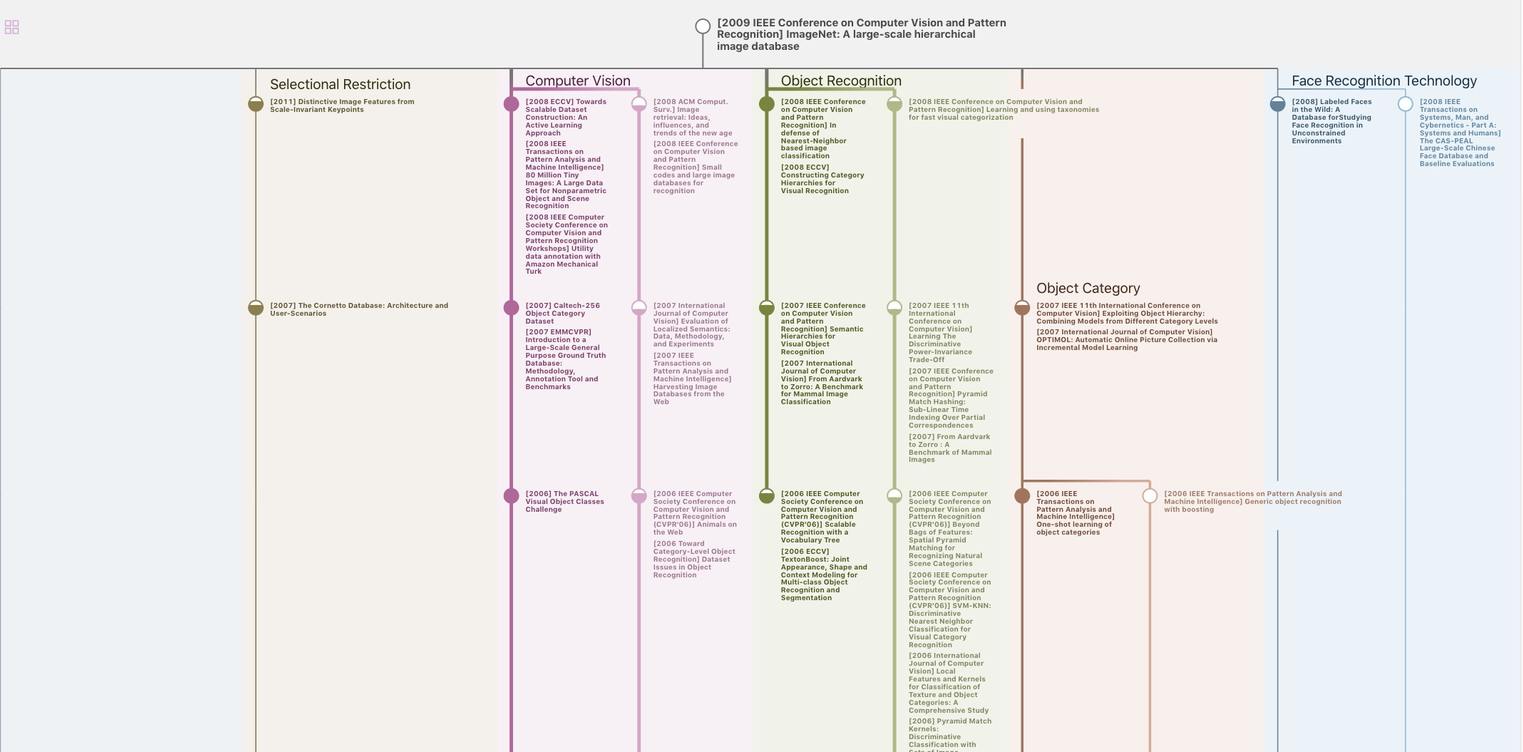
Generate MRT to find the research sequence of this paper
Data Disclaimer
The page data are from open Internet sources, cooperative publishers and automatic analysis results through AI technology. We do not make any commitments and guarantees for the validity, accuracy, correctness, reliability, completeness and timeliness of the page data. If you have any questions, please contact us by email: report@aminer.cn
Chat Paper
GPU is busy, summary generation fails
Rerequest