Latent Stochastic Differential Equations for Change Point Detection.
IEEE ACCESS(2023)
Natl Res Univ Higher Sch Econ HSE Univ
Abstract
Automated analysis of complex systems based on multiple readouts remains a challenge. Change point detection algorithms are aimed to locating abrupt changes in the time series behaviour of a process. In this paper, we present a novel change point detection algorithm based on Latent Neural Stochastic Differential Equations (SDE). Our method learns a non-linear deep learning transformation of the process into a latent space and estimates a SDE that describes its evolution over time. The algorithm uses the likelihood ratio of the learned stochastic processes in different timestamps to find change points of the process. We demonstrate the detection capabilities and performance of our algorithm on synthetic and real-world datasets. The proposed method outperforms the state-of-the-art algorithms on the majority of our experiments.
MoreTranslated text
Key words
Time series analysis,Signal processing algorithms,Deep learning,Approximation algorithms,Task analysis,Monitoring,Machine learning algorithms,Anomaly detection,Machine learning,change point detection,deep learning,machine learning,timeseries
PDF
View via Publisher
AI Read Science
Must-Reading Tree
Example
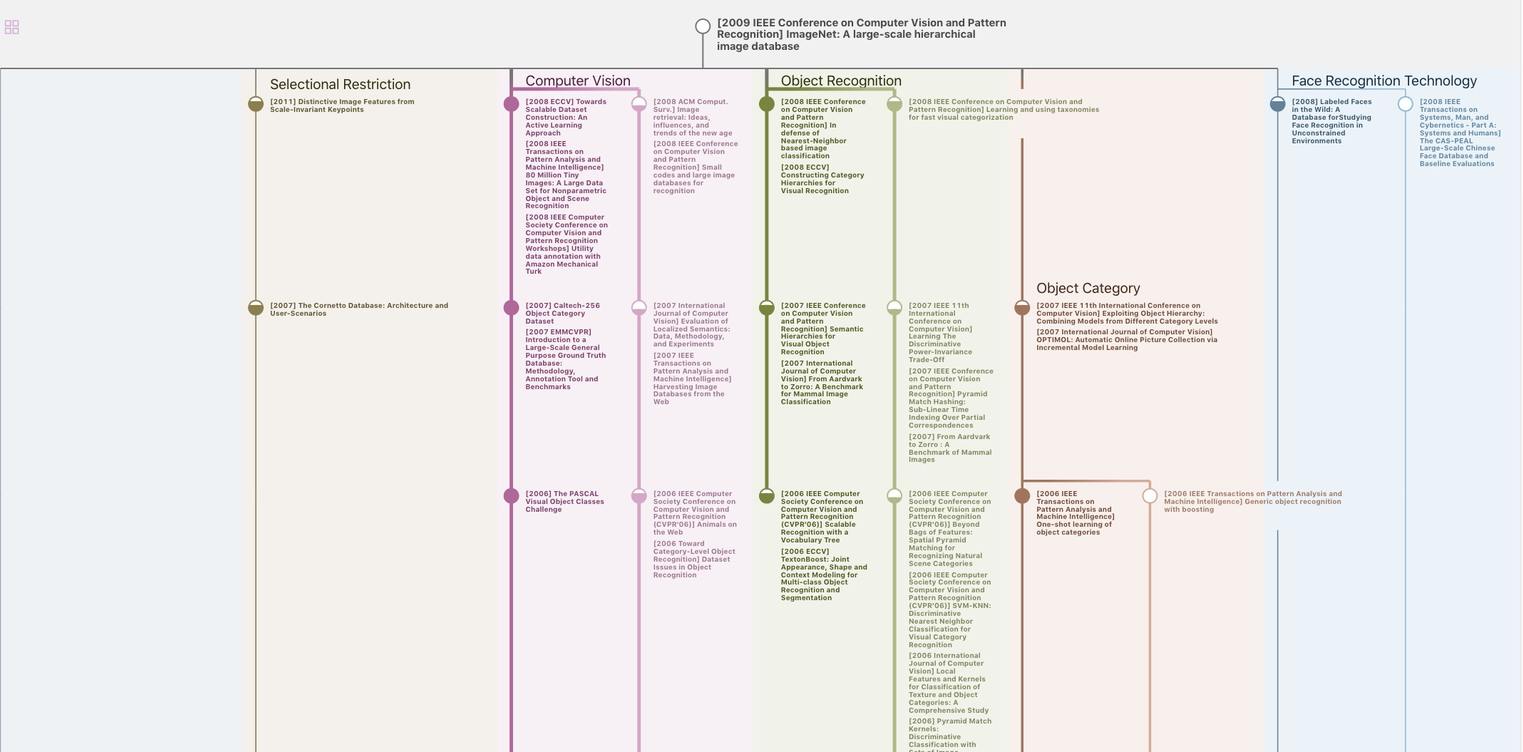
Generate MRT to find the research sequence of this paper
Related Papers
2012
被引用23 | 浏览
2001
被引用53 | 浏览
2017
被引用94 | 浏览
2018
被引用15 | 浏览
2021
被引用40 | 浏览
Data Disclaimer
The page data are from open Internet sources, cooperative publishers and automatic analysis results through AI technology. We do not make any commitments and guarantees for the validity, accuracy, correctness, reliability, completeness and timeliness of the page data. If you have any questions, please contact us by email: report@aminer.cn
Chat Paper
去 AI 文献库 对话