Improving Multilayer-Perceptron(MLP)-based Network Anomaly Detection with Birch Clustering on CICIDS-2017 Dataset
arxiv(2022)
摘要
Machine learning algorithms have been widely used in intrusion detection systems, including Multi-layer Perceptron (MLP). In this study, we proposed a two-stage model that combines the Birch clustering algorithm and MLP classifier to improve the performance of network anomaly multi-classification. In our proposed method, we first apply Birch or Kmeans as an unsupervised clustering algorithm to the CICIDS-2017 dataset to pre-group the data. The generated pseudo-label is then added as an additional feature to the training of the MLP-based classifier. The experimental results show that using Birch and K-Means clustering for data pre-grouping can improve intrusion detection system performance. Our method can achieve 99.73% accuracy in multi-classification using Birch clustering, which is better than similar researches using a stand-alone MLP model.
更多查看译文
关键词
Intrusion Detection,Anomaly Detection,CICIDS2017 dataset,Multilayer Perceptron,Multi-classification,Clustering Algorithm
AI 理解论文
溯源树
样例
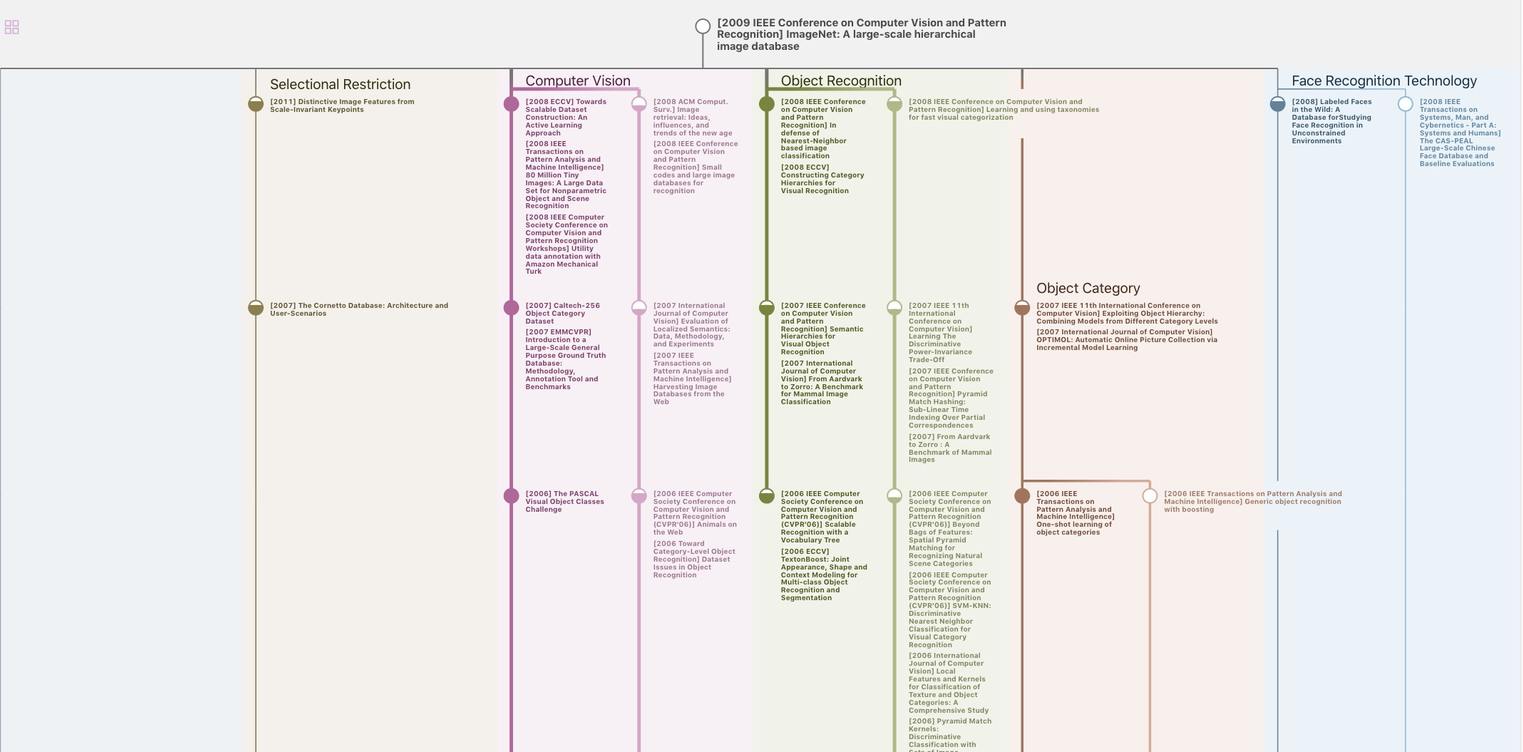
生成溯源树,研究论文发展脉络
Chat Paper
正在生成论文摘要