Make Fairness More Fair: Fair Item Utility Estimation and Exposure Re-Distribution
KDD '22: Proceedings of the 28th ACM SIGKDD Conference on Knowledge Discovery and Data Mining(2022)
摘要
The item fairness issue has become one of the significant concerns with the development of recommender systems in recent years, focusing on whether items' exposures are consistent with their utilities. So the measurement of item unfairness depends on the modeling of item utility, and most previous approaches estimated item utility simply based on user-item interaction logs in recommender systems. The Click-through rate (CTR) is the most popular one. However, we argue that these types of item utilities (named observed utility here) measurements may result in unfair exposures of items. The number of exposure for each item is uneven, and recommendation methods select the exposure audiences (users). In this work, we propose the concept of items' fair utility, defined as the proportion of users who are interested in the item among all users. Firstly, we conduct a large-scale random exposure experiment to collect the fair utility in a real-world recommender application. Significant differences are observed between the fair utility and the widely used observed utility (CTR). Then, intending to obtain fair utility at a low cost, we propose an exploratory task for real-time estimations of fair utility with handy historical interaction logs. Encouraging results are achieved, validating the feasibility of fair utility projections. Furthermore, we present a fairness-aware re-distribution framework and conduct abundant simulation experiments, adopting fair utility to improve fairness and overall recommendation performance at the same time. Online and offline results show that both item fairness and recommendation quality can be improved simultaneously by introducing item fair utility.
更多查看译文
AI 理解论文
溯源树
样例
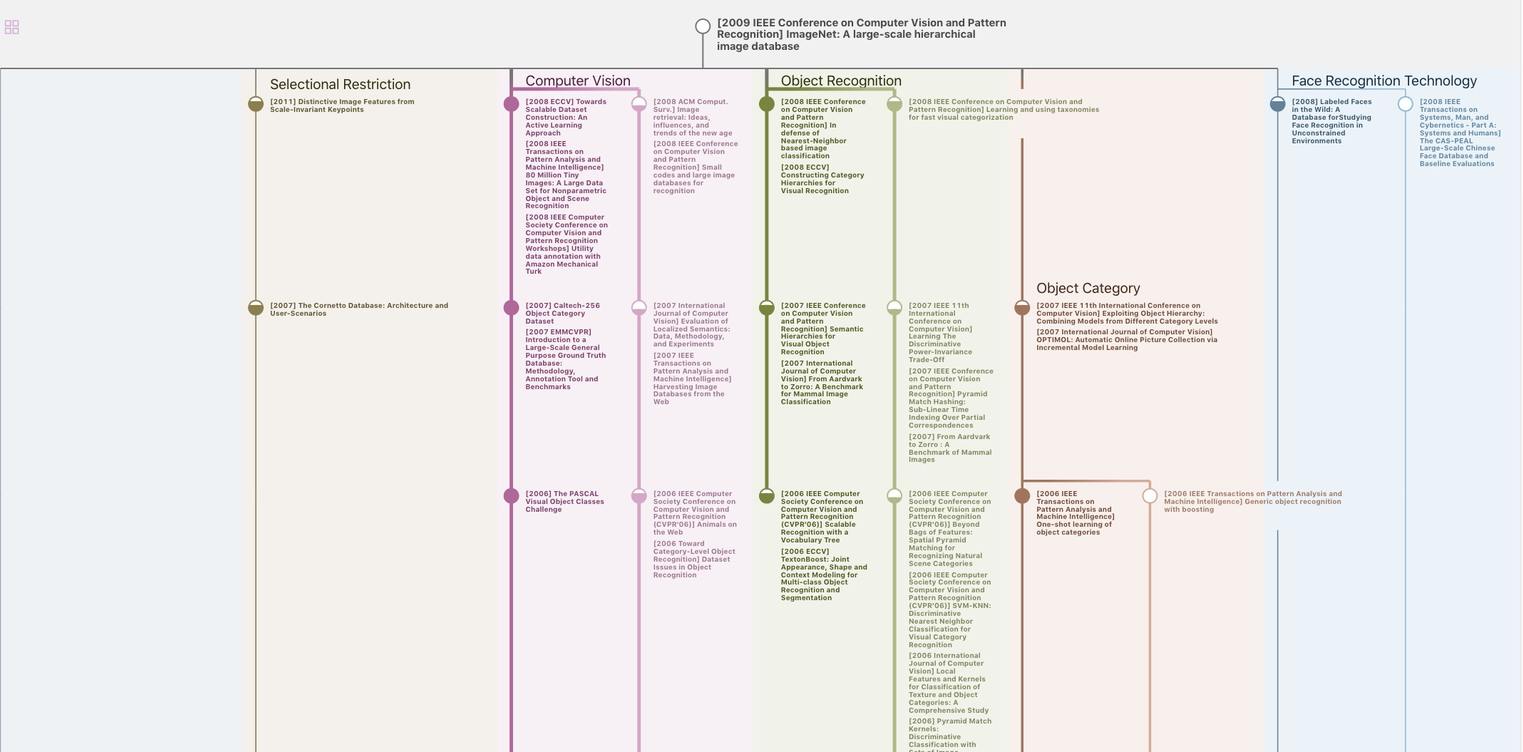
生成溯源树,研究论文发展脉络
Chat Paper
正在生成论文摘要