External Knowledge Infusion for Tabular Pre-training Models with Dual-adapters
KDD '22: Proceedings of the 28th ACM SIGKDD Conference on Knowledge Discovery and Data Mining(2022)
摘要
Tabular pre-training models have received increasing attention due to the wide-ranging applications for tabular data analysis. However, most of the existing solutions are directly built upon the tabular data with a mixture of non-semantic and semantic contents. According to the statistics, only 30% of tabular data in wikitables are semantic entities that are surrounded and isolated by enormous irregular characters such as numbers, strings, symbols, etc. Despite the small portion, such semantic entities are crucial for table understanding. This paper attempts to enhance the existing tabular pre-training model by injecting common-sense knowledge from external sources. Compared with the knowledge injection in the natural language pre-training models, the tabular model naturally requires overcoming the domain gaps between external knowledge and tabular data with significant differences in both structures and contents. To this end, we propose the dual-adapters inserted within the pre-trained tabular model for flexible and efficient knowledge injection. The two parallel adapters are trained by the knowledge graph triplets and semantically augmented tables respectively for infusion and alignment with the tabular data. In addition, a path-wise attention layer is attached below to fuse the cross-domain representation with the weighted contribution. Finally, to verify the effectiveness of our proposed knowledge injection framework, we extensively test it on 5 different application scenarios covering both zero-shot and finetuning-based tabular understanding tasks over the cell, column, and tables levels.
更多查看译文
关键词
knowledge,models,pre-training,dual-adapters
AI 理解论文
溯源树
样例
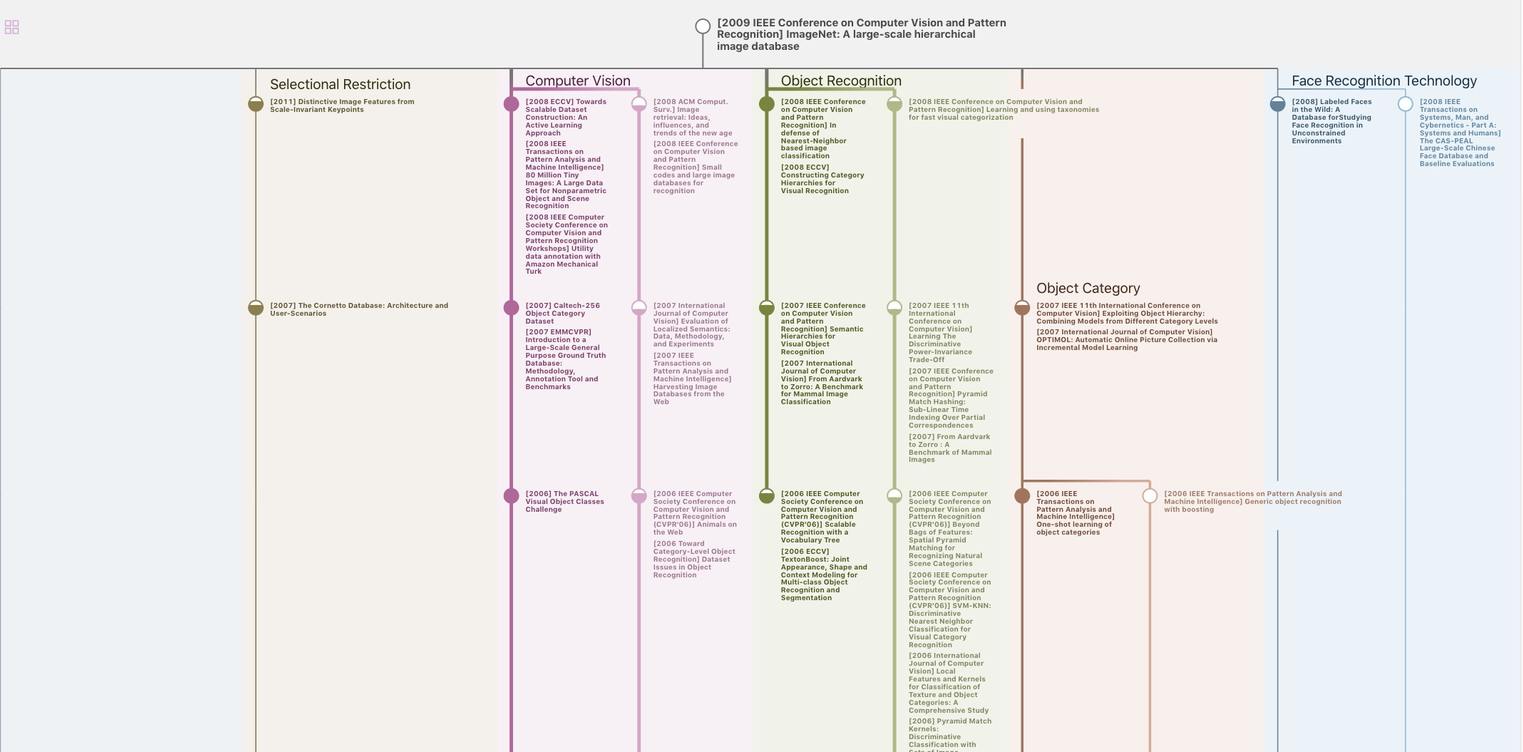
生成溯源树,研究论文发展脉络
Chat Paper
正在生成论文摘要