Connecting the Hosts: Street-Level IP Geolocation with Graph Neural Networks
Proceedings of the 28th ACM SIGKDD Conference on Knowledge Discovery and Data Mining(2022)
摘要
Pinpointing the geographic location of an IP address is important for a range of location-aware applications spanning from targeted advertising to fraud prevention. The majority of traditional measurement-based and recent learning-based methods either focus on the efficient employment of topology or utilize data mining to find clues of the target IP in publicly available sources. Motivated by the limitations in existing works, we propose a novel framework named GraphGeo, which provides a complete processing methodology for street-level IP geolocation with the application of graph neural networks. It incorporates IP hosts knowledge and kinds of neighborhood relationships into the graph to infer spatial topology for high-quality geolocation prediction. We explicitly consider and alleviate the negative impact of uncertainty caused by network jitter and congestion, which are pervasive in complicated network environments. Extensive evaluations across three large-scale real-world datasets demonstrate that GraphGeo significantly reduces the geolocation errors compared to the state-of-the-art methods. Moreover, the proposed framework has been deployed on the web platform as an online service for 6 months.
更多查看译文
关键词
IP Geolocation,Network Topology,Graph Neural Networks,Uncertain Inference,Ubiquitous Computing
AI 理解论文
溯源树
样例
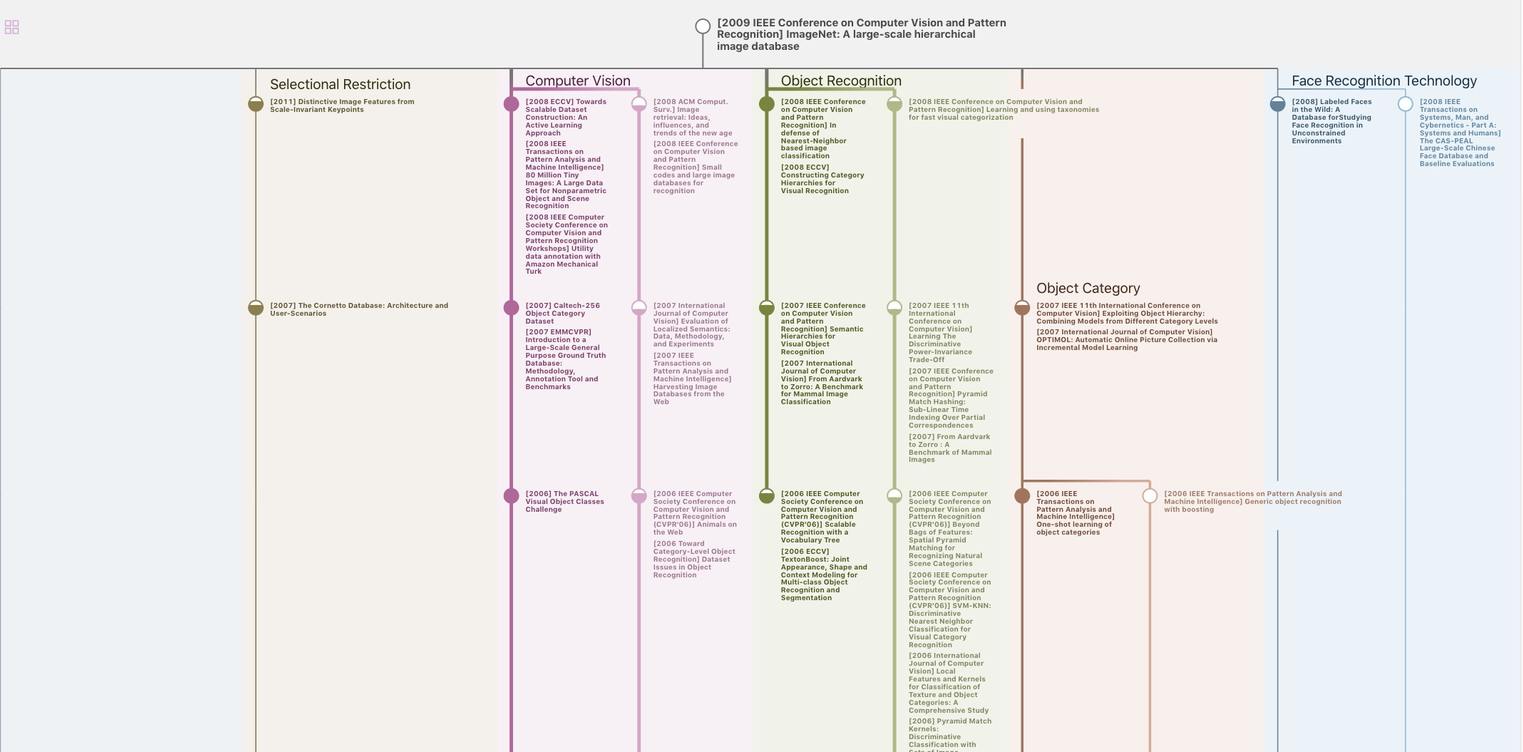
生成溯源树,研究论文发展脉络
Chat Paper
正在生成论文摘要