Out-of-distribution Detection via Frequency-regularized Generative Models
WACV(2023)
摘要
Modern deep generative models can assign high likelihood to inputs drawn from outside the training distribution, posing threats to models in open-world deployments. While much research attention has been placed on defining new test-time measures of OOD uncertainty, these methods do not fundamentally change how deep generative models are regularized and optimized in training. In particular, generative models are shown to overly rely on the background information to estimate the likelihood. To address the issue, we propose a novel frequency-regularized learning (FRL) framework for OOD detection, which incorporates highfrequency information into training and guides the model to focus on semantically relevant features. FRL effectively improves performance on a wide range of generative architectures, including variational auto-encoder, GLOW, and PixelCNN++. On a new large-scale evaluation task, FRL achieves the state-of-the-art performance, outperforming a strong baseline Likelihood Regret by 10.7% (AUROC) while achieving 147x faster inference speed. Extensive ablations show that FRL improves the OOD detection performance while preserving the image generation quality. Code is available at https://github.com/mu-cai/FRL.
更多查看译文
关键词
Algorithms: Explainable,fair,accountable,privacy-preserving,ethical computer vision,Computational photography,image and video synthesis
AI 理解论文
溯源树
样例
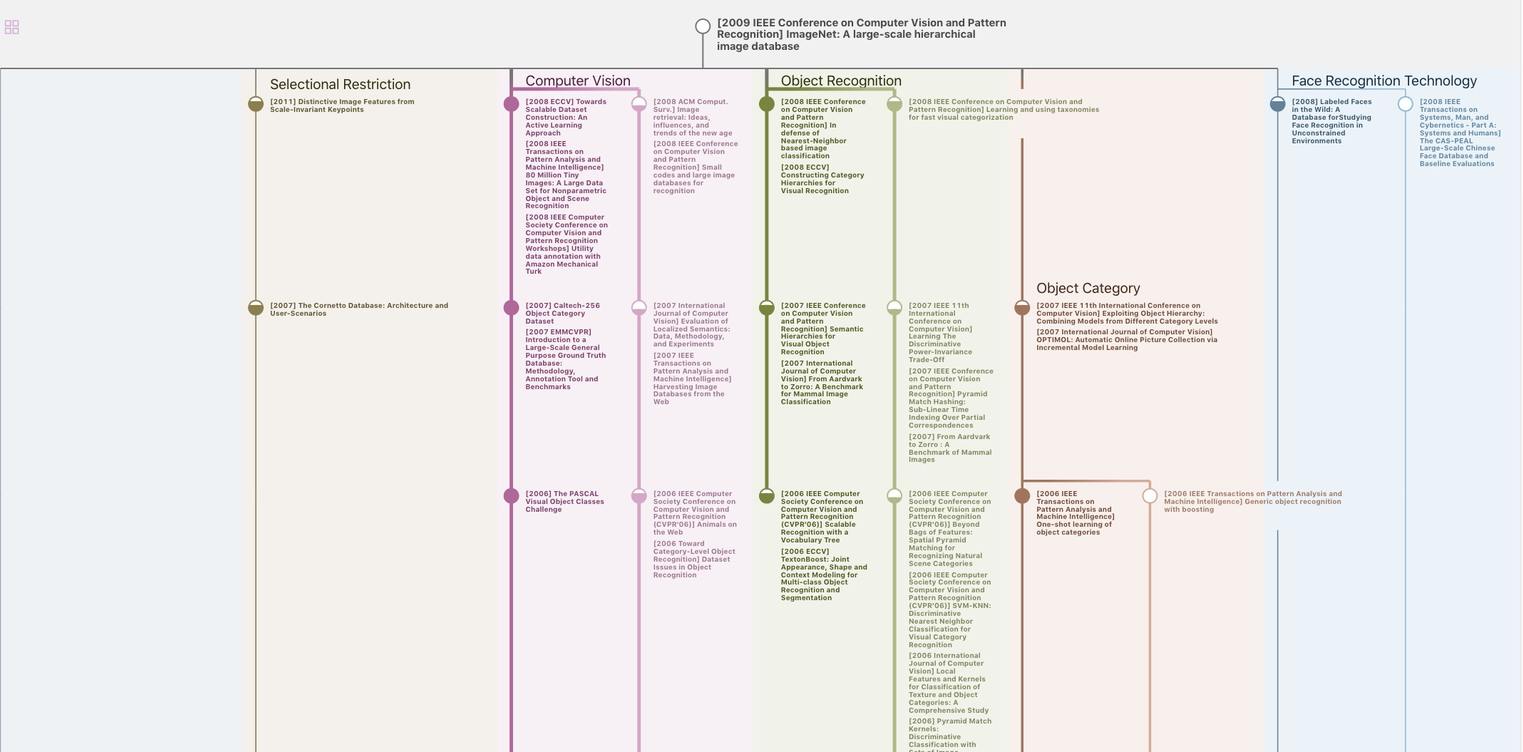
生成溯源树,研究论文发展脉络
Chat Paper
正在生成论文摘要