Application Runtime Estimation for AURIX Embedded MCU Using Deep Learning
EMBEDDED COMPUTER SYSTEMS: ARCHITECTURES, MODELING, AND SIMULATION, SAMOS 2022(2022)
摘要
Estimating execution time is a crucial task during the development of safety-critical embedded systems. Processor simulation or emulation tools on various abstraction levels offer a trade-off between accuracy and runtime. Typically, this requires detailed knowledge of the processor architecture and high manual effort to construct adequate models. In this paper, we explore how deep learning may be used as an alternative approach for building processor performance models. First, we describe how to obtain training data from recorded execution traces. Next, we evaluate various neural network architectures and hyperparameter values. The accuracy of the best network variants is finally compared to two simple baseline models and a mechanistic model based on the QEMU emulator. As an outcome of this evaluation, a model based on the Wavenet architecture is identified, which outperforms all other approaches by achieving a mean absolute percentage error of only 1.63%.
更多查看译文
关键词
Embedded processor,Performance estimation,Artificial neural networks
AI 理解论文
溯源树
样例
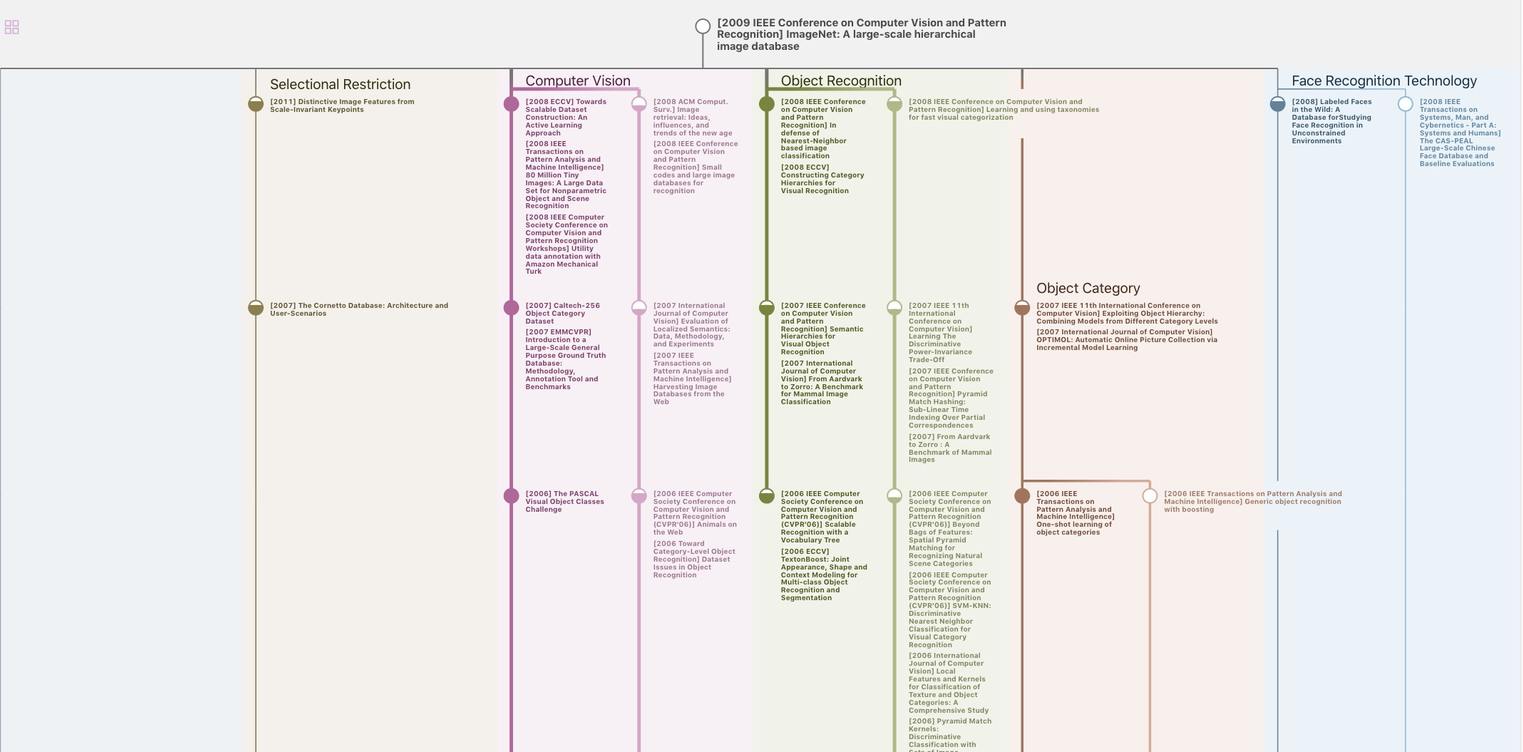
生成溯源树,研究论文发展脉络
Chat Paper
正在生成论文摘要