Deep Learning and Infrared Spectroscopy: Representation Learning with a beta-Variational Autoencoder
JOURNAL OF PHYSICAL CHEMISTRY LETTERS(2022)
摘要
ABSTRACT: Infrared (IR) spectra contain detailed and extensive information about the chemical composition and bonding environment in a sample. However, this information is difficult to extract from complex heterogeneous systems because of overlapping absorptions due to different generative factors. We implement a deep learning approach to study the complex spectroscopic changes that occur in cross-linked polyethylene (PEXa) pipe by training a ??-variational autoencoder (??-VAE) on a database of PEX-a pipe spectra. We show that the ??-VAE outperforms principal component analysis (PCA) and learns interpretable and independent representations of the generative factors of variance in the spectra. We apply the ??-VAE encoder to a hyperspectrum of a crack in the wall of a pipe to evaluate the spatial distribution of these learned representations. This study shows how deep learning architectures like ??-VAE can enhance the analysis of spectroscopic data of complex heterogeneous systems.
更多查看译文
AI 理解论文
溯源树
样例
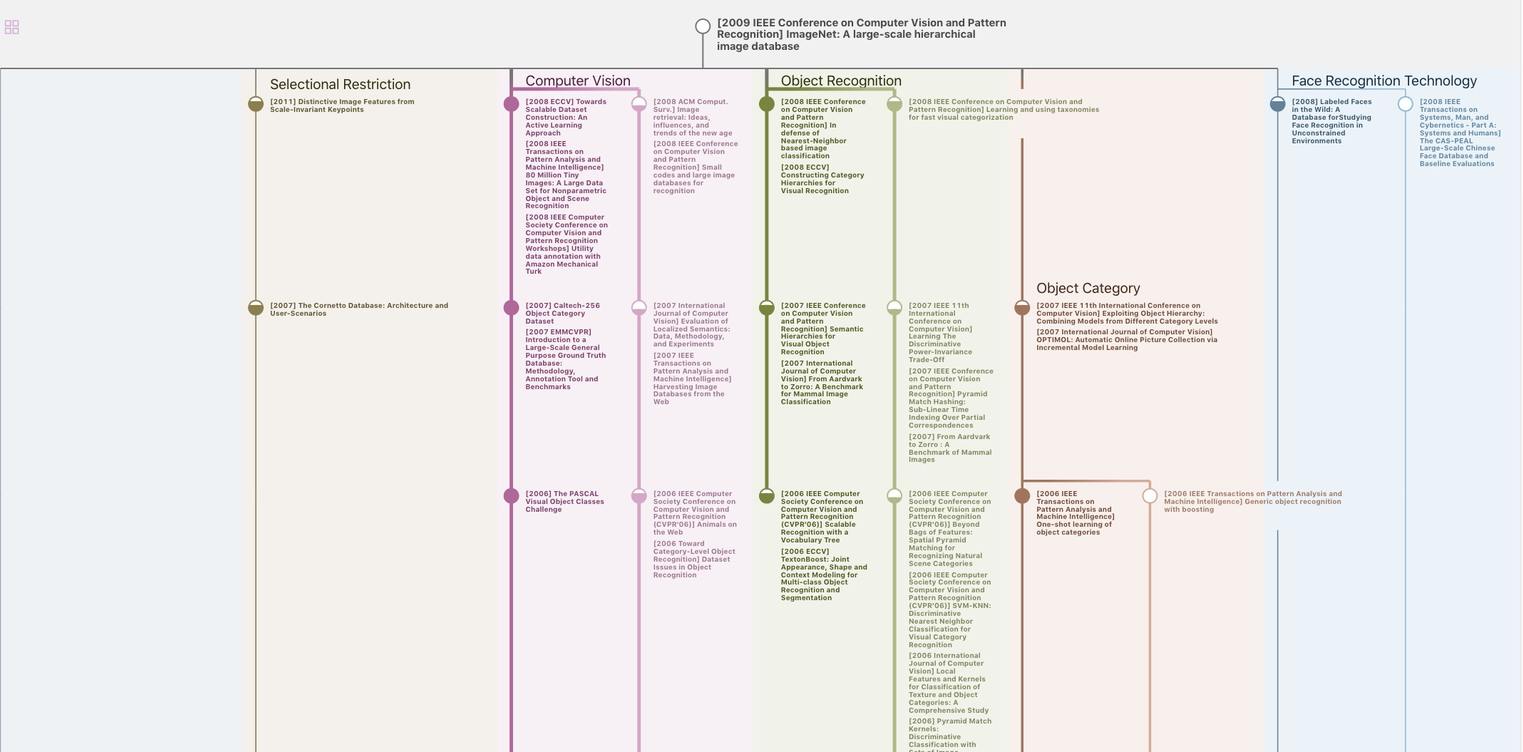
生成溯源树,研究论文发展脉络
Chat Paper
正在生成论文摘要