The Performance Analysis of Time Series Data Augmentation Technology for Small Sample Communication Device Recognition
IEEE TRANSACTIONS ON RELIABILITY(2023)
关键词
Time series analysis,Deep learning,Interpolation,Convolution,Neural networks,Training,Feature extraction,Communication device recognition,complex neural network,small sample recognition,time series data augmentation,virtual confrontation training (VAT)
AI 理解论文
溯源树
样例
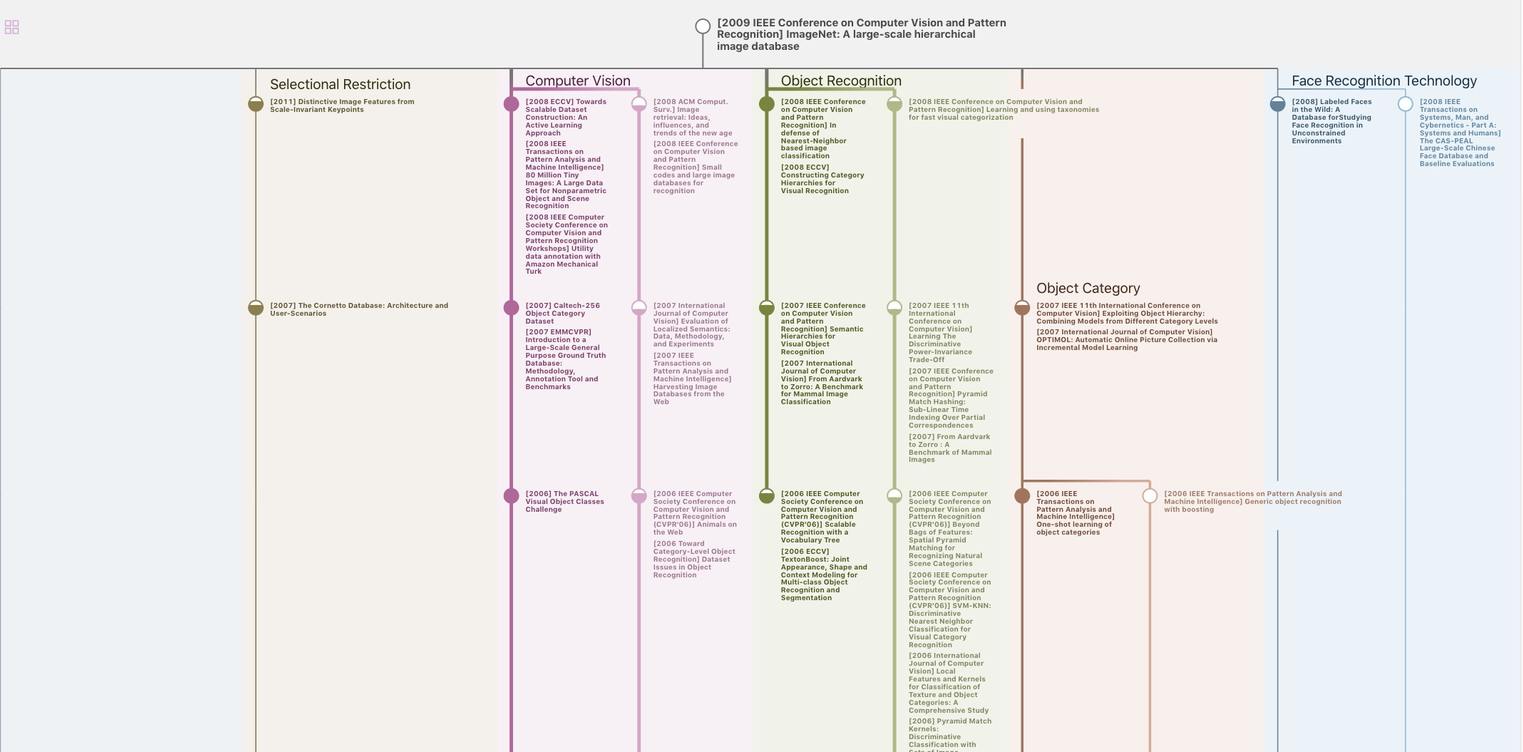
生成溯源树,研究论文发展脉络
Chat Paper
正在生成论文摘要