An evaluation of machine learning for genomic prediction of hairy syndrome in dairy cattle
ANIMAL SCIENCE PAPERS AND REPORTS(2022)
摘要
There is a growing body of literature that recognizes the importance of understanding the adaptation of the cattle populations to the climate conditions. Among others heat stress is one of the causes of reduction in feed intake, decreased milk yields, shortened lactation and reduced fertility. Genomic prediction of the phenotype is one of the most frequently stated problem in connection with genome wide association studies (GWAS) and genomic selection. Investigation on genomic prediction of hairy syndrome in cattle is important for our increased understanding of adaptive evolutions due to the climate change. This research examines the emerging role in the context of genomic prediction of hairy and slick condition of the cattle using both Bayesian learning (BL) (Bayesian ridge regression, Bayesian LASSO, Bayes A, Bayes B and Bayes C and machine learning (ML) methods (weighted subspace random forest (wRF), gradient boosted trees (GBT), na??ve Bayes (NB) and K-nearest neighbors (KNN) under various experimental designs. The dataset included 99 (37 cases and 62 controls: defined by visual inspection of hairiness) crossbred cows genotyped for 712 222 SNPs. This study set out with the aim of assessing the importance of linkage disequilibrium, population structure, and various SNPs selection process (for only ML) for genomic prediction of hairiness using BL and ML models. The most obvious finding to emerge from this study is the superiority of ML model over BL models for genomic prediction of the phenotype. This study supports evidence from previous observations on beneficial usage of ML model in genetics and genomics research. The relevance of wRF is clearly supported by the current findings. The wRF with GWAS selected SNPs of 15000 gave the best prediction accuracy (with standard error in parenthesis, Area Under Curve=0.998 (0.004)). Despite its relatively small sampling size: these data suggest that ML prediction of hairiness can be achieved through high prediction accuracies hence the finding of this study have a number of important dairy cattle breeding implications for future practice in response to the climate change problem.
更多查看译文
关键词
Bayesian learning,genomic prediction,hairy syndrome,machine learning
AI 理解论文
溯源树
样例
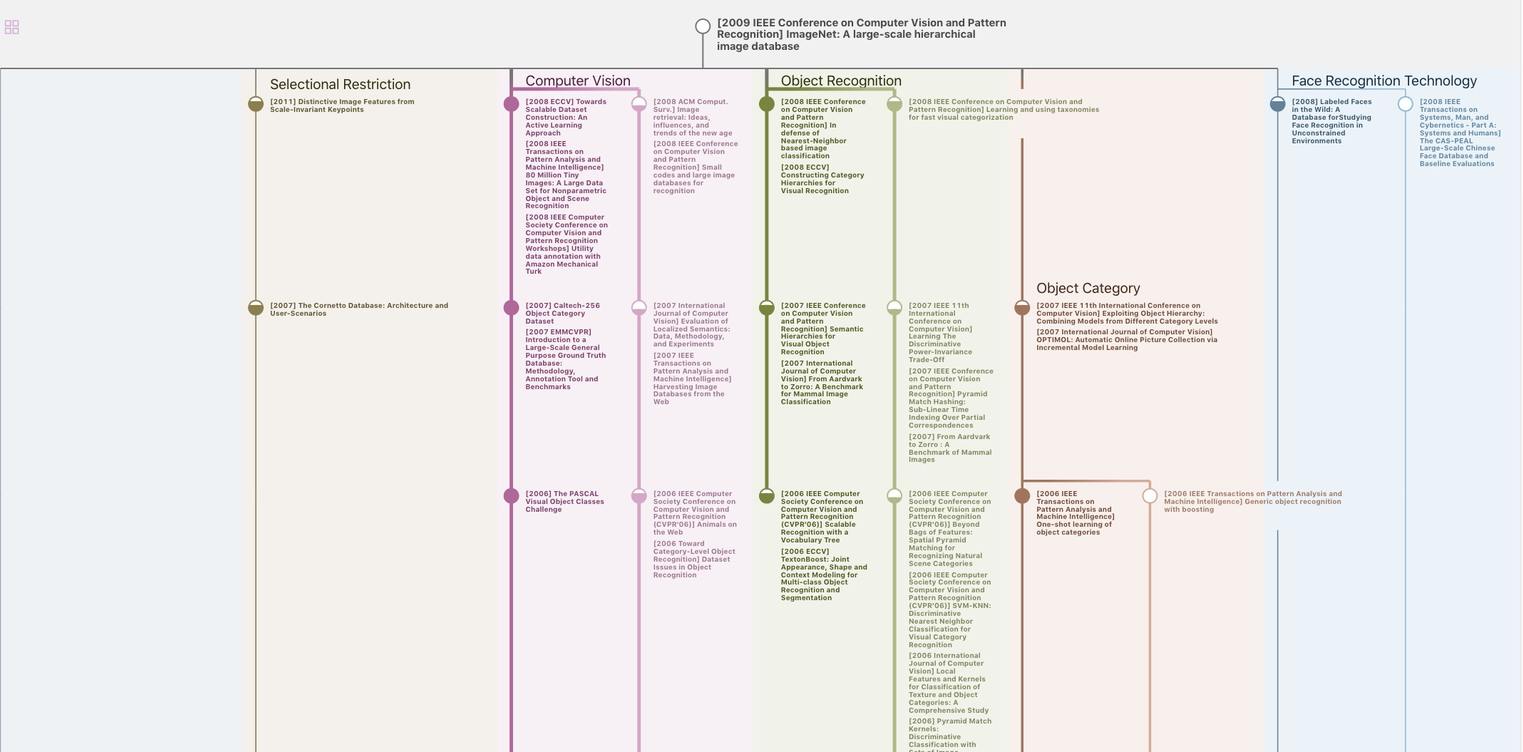
生成溯源树,研究论文发展脉络
Chat Paper
正在生成论文摘要