Relevance-based label distribution feature selection via convex optimization
Information sciences(2022)
摘要
In label distribution learning, high dimensionality is one of the most prominent character-istics of the data, which increases the model complexity and computational cost. Feature selection is an efficient technique to mitigate the "curse of dimensionality". The current label distribution feature selection approach based on mutual information employs the heuristic search algorithm to identify the most discriminative features. However, this method can be time-consuming and fall into local optimum. Motivated by this, in this paper, an approach named relevance-based label distribution feature selection via convex optimization is proposed, which takes both feature relevance and label relevance (i.e., label correlation) into account. For features, the relevance to labels calculated by mutual infor-mation is considered into a convex optimization function to guide the feature selection process. Compared with the heuristic search, the learning framework for optimization pur -poses is more conducive to reducing repetitive computations and avoiding the local opti-mum. For labels, the Pearson correlation coefficient is exploited to describe the correlation information between labels, aiming to enhance the generalization ability of the learning model. Comprehensive experiments are conducted on twenty datasets, and the results demonstrate the effectiveness of the proposed method compared with eight state-of -the-art methods in terms of six evaluation metrics.(c) 2022 Elsevier Inc. All rights reserved.
更多查看译文
关键词
Feature selection,Mutual information,Label correlation,Label distribution,Feature relevance,Convex optimization
AI 理解论文
溯源树
样例
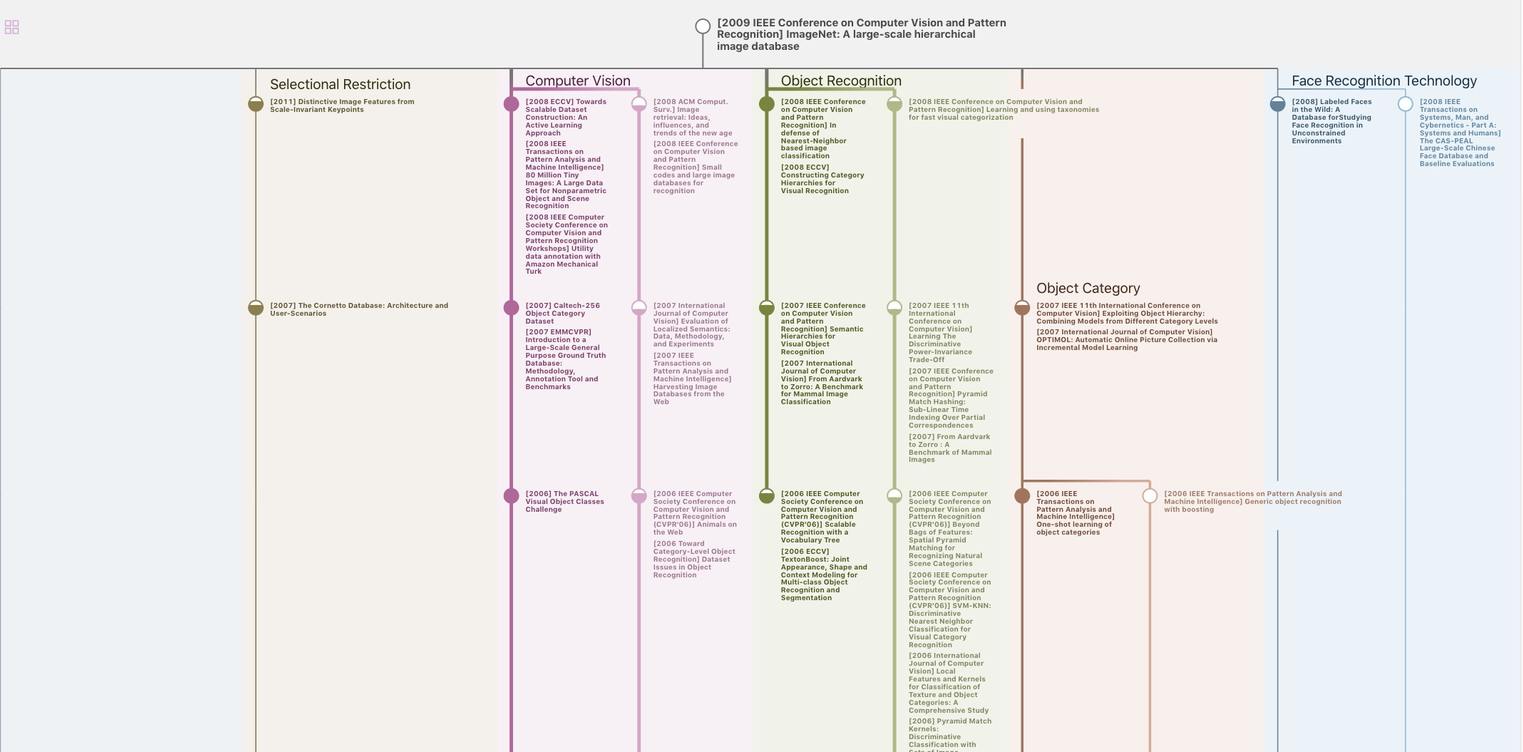
生成溯源树,研究论文发展脉络
Chat Paper
正在生成论文摘要