Prediction of Compressive Strength in Plain and Blended Cement Concretes Using a Hybrid Artificial Intelligence Model
Case studies in construction materials(2022)
摘要
Concrete is a complex engineering material with different ingredients and design variables. Therefore, an accurate prediction of concrete compressive strength (CCS) should consider its nonlinearity. Data-driven machine learning approaches can solve highly non-linear problems. The objective of this paper is to utilize computational intelligence techniques, especially the support vector machine (SVM) and the genetic algorithm (GA) as the hybridized model (SVM-GA) to reliably predict concrete compressive strength based on a set of independent variables such as Ordinary Portland Cement (OPC), Silica Fume (SF), Fly As (FA), Cement Content, Water Content. Such an approach provides a fast and simple way to optimize parameter settings to obtain an optimum laboratory condition of the CCS process. The study shows that the concrete compression strength increases with increasing silica fume (SF), cement content and curing time, and a higher correlation coefficient in SF was observed compared to cement content. In addition, the correlation coefficients for Fly Ash (FA), water and aggregates were found to be −0.38, −0.28 and −0.28, respectively, which has indicated a significant decrease in concrete compressive strength with these parameters and that the addition of FA is more significant in decreasing the compressive strength of concrete than water content and both coarse and fine aggregates and this can be explained by the low pozzolanic reactivity of FA in the early stages of concrete hydration. The experimental results demonstrated that the proposed model has promising potential with correlations of R2 = 0.99 achieved during the testing stages of the prediction of concrete compression strength. Furthermore, the experimental results demonstrate that the predicted values obtained from the model (SVM-GA) correspond closely to the experimental results with the root mean square error (RMSE)= 0.002.
更多查看译文
关键词
Compressive strength,Blended cement,Prediction,Artificial intelligence,Support vector machine,Genetic algorithm
AI 理解论文
溯源树
样例
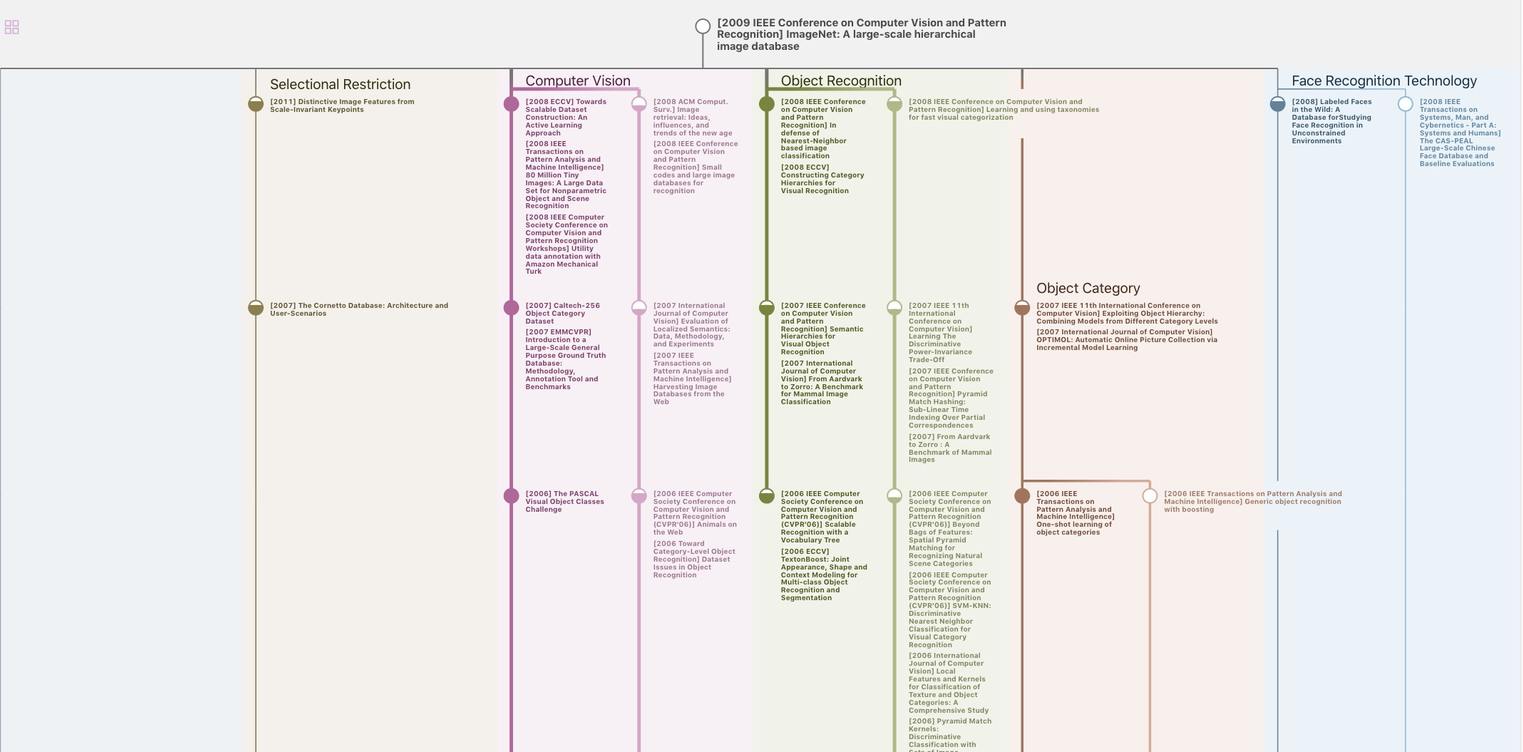
生成溯源树,研究论文发展脉络
Chat Paper
正在生成论文摘要