A Weighted Deep Learning Model with Sampling Strategy for Unbalanced Bearing Condition Assessment
2022 IEEE 5th International Electrical and Energy Conference (CIEEC)(2022)
摘要
Rolling bearings play an extremely important role in rotating machinery. The unique position of bearings dictates that in the event of a failure, this could result in huge economic losses as well as endangering personal safety. Therefore, the assessment of the failure state of bearings has been a popular area of research. This paper addresses two challenges that exist in traditional bearing condition assessment methods: the first is the need to manually extract features from bearing data, which is overly dependent on professional knowledge; the second is the general imbalance between faulty and normal samples, which affects the performance of the model. The paper proposes a two-layer CNN-LSTM weighted deep learning model with sampling strategy (wTLCL-O) to address these challenges, which contains two layers of CNN, two layers of LSTM with both an oversampling strategy and a weighted cost-sensitive loss function. The article conducts experiments on the French PRONOSTIA bearing failure dataset, and the results show that wTLCL-O outperforms other traditional algorithms in terms of robustness and stability, and improves the prediction accuracy of bearing condition assessment under non-equilibrium conditions.
更多查看译文
关键词
Bearing condition assessment,deep learning,feature extraction,sample imbalance,CNN-LSTM
AI 理解论文
溯源树
样例
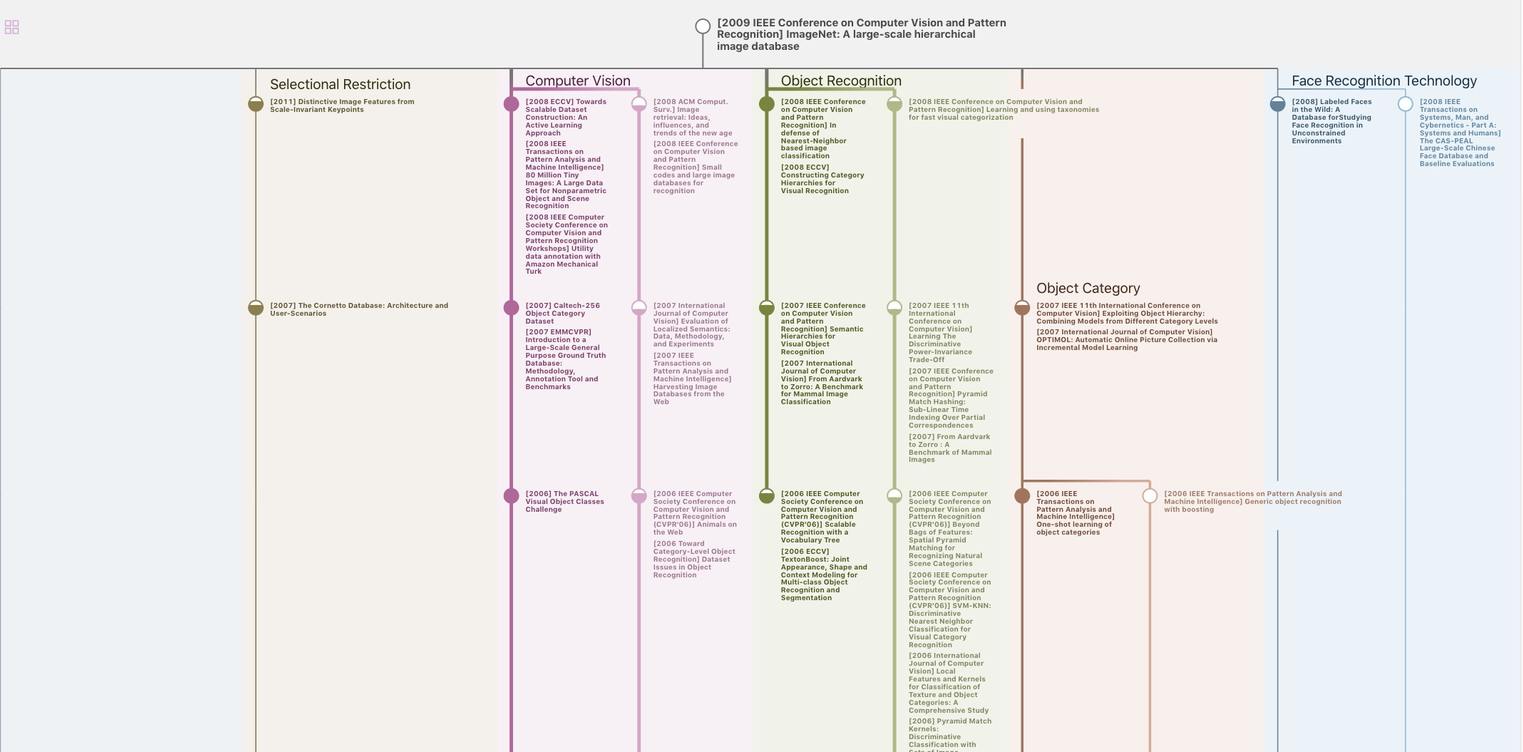
生成溯源树,研究论文发展脉络
Chat Paper
正在生成论文摘要