Accuracy of A New Method for Semi-Quantitative Assessment of Right Ventricular Ejection Fraction by Cardiac Mri: Right Ventricular Fractional Diameter Changes
European Journal of Radiology(2013)SCI 2区SCI 3区
Abstract
Objective: Longitudinal shortening is traditionally considered the predominant part of global right ventricular (RV) systolic function. Less attention has been paid to transverse contraction. The aim of this study was to evaluate RV transverse motion by cardiovascular magnetic resonance (CMR) in a large cohort of patients and to assess its relationship with RV ejection fraction (RVEF).Study design: We retrospectively analyzed the CMR scans of 300 patients referred to our center in 2010. RVEF was determined from short axis sequences using the volumetric method. Transverse parameters called RV fractional diameter changes were calculated after measuring RV diastolic and systolic diameters at basal and mid-level in short axis view (respectively FBDC and FMDC). We also measured the tricuspid annular plane systolic excursion (TAPSE) as a longitudinal reference.Results: Our population was divided into 2 groups according to RVEF. 250 patients had a preserved RVEF (>40%) and 50 had a RV dysfunction (RVEF <= 40%). Transverse and longitudinal motions were significantly reduced in the group with RV dysfunction (p < .0001). After ROC analysis, areas under the curve for FBDC, FMDC and TAPSE, were respectively 0.79, 0.82 and 0.72, with the highest specificity and sensitivity respectively of 88% and 68% for FMDC (threshold at 20%) for predicting RV dysfunction. FMDC had an excellent negative predictive value of 93%.Conclusion: RV fractional diameter changes, especially at the mid-level, appear to be accurate for semi-quantitative assessment of RV function by CMR. A cut-off of 20% for FMDC differentiates patients with a low (EF <= 40%) or a preserved RVEF. (C) 2013 Elsevier Ireland Ltd. All rights reserved.
MoreTranslated text
Key words
Cardiovascular Magnetic Resonance,Short Axis,Short Axis View,Transverse Motion,Right Ventricular Ejection Fraction
PDF
View via Publisher
AI Read Science
AI Summary
AI Summary is the key point extracted automatically understanding the full text of the paper, including the background, methods, results, conclusions, icons and other key content, so that you can get the outline of the paper at a glance.
Example
Background
Key content
Introduction
Methods
Results
Related work
Fund
Key content
- Pretraining has recently greatly promoted the development of natural language processing (NLP)
- We show that M6 outperforms the baselines in multimodal downstream tasks, and the large M6 with 10 parameters can reach a better performance
- We propose a method called M6 that is able to process information of multiple modalities and perform both single-modal and cross-modal understanding and generation
- The model is scaled to large model with 10 billion parameters with sophisticated deployment, and the 10 -parameter M6-large is the largest pretrained model in Chinese
- Experimental results show that our proposed M6 outperforms the baseline in a number of downstream tasks concerning both single modality and multiple modalities We will continue the pretraining of extremely large models by increasing data to explore the limit of its performance
Try using models to generate summary,it takes about 60s
Must-Reading Tree
Example
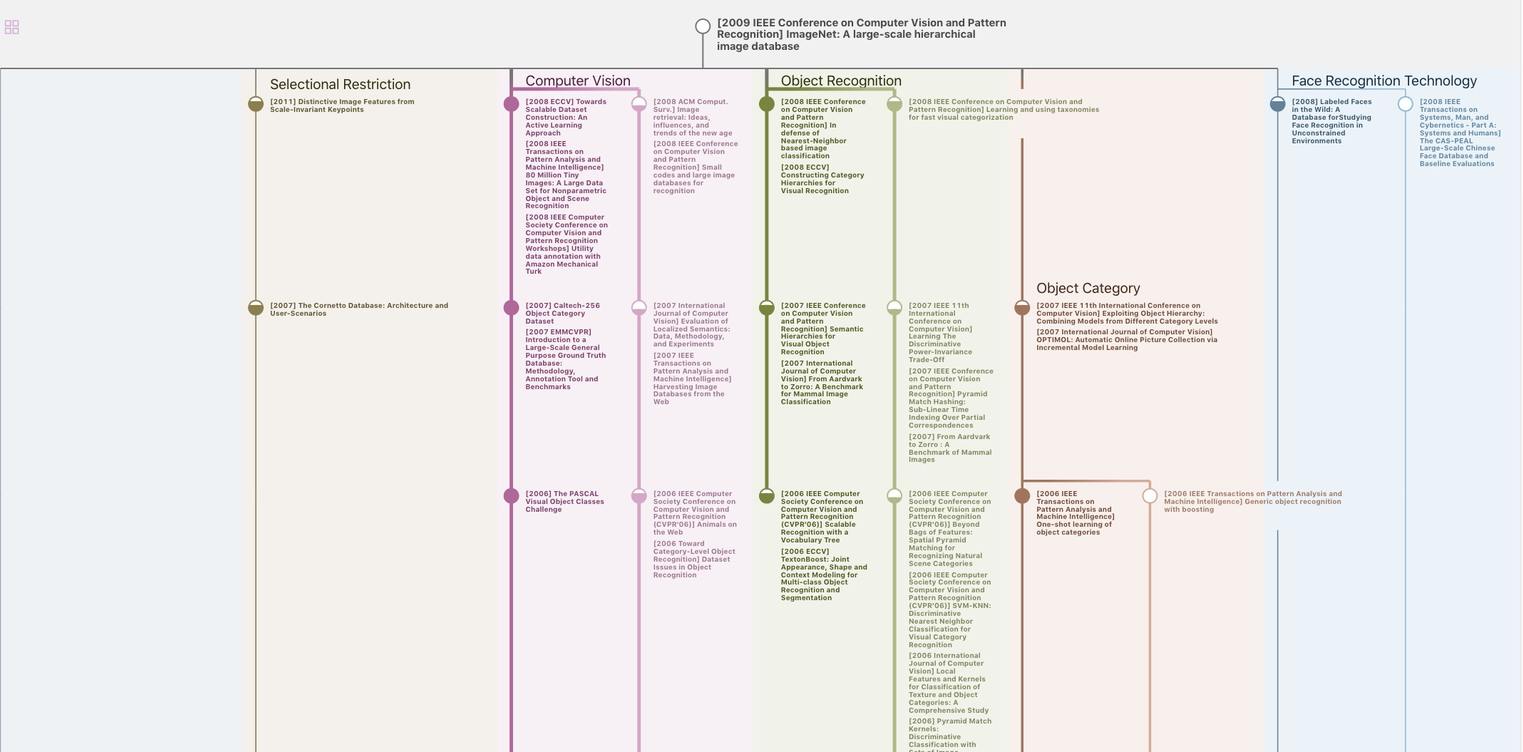
Generate MRT to find the research sequence of this paper
Related Papers
2004
被引用115 | 浏览
2007
被引用132 | 浏览
1994
被引用199 | 浏览
2004
被引用740 | 浏览
2006
被引用291 | 浏览
2010
被引用199 | 浏览
1997
被引用321 | 浏览
Two‐Dimensional Assessment of Right Ventricular Function: an Echocardiographic–MRI Correlative Study
2007
被引用343 | 浏览
1993
被引用208 | 浏览
2012
被引用135 | 浏览
2008
被引用284 | 浏览
Data Disclaimer
The page data are from open Internet sources, cooperative publishers and automatic analysis results through AI technology. We do not make any commitments and guarantees for the validity, accuracy, correctness, reliability, completeness and timeliness of the page data. If you have any questions, please contact us by email: report@aminer.cn
Chat Paper
去 AI 文献库 对话