Adaptive kernel density estimation proposal in gravitational wave data analysis
arxiv(2023)
摘要
The Markov Chain Monte Carlo approach is frequently used within a Bayesian framework to sample the target posterior distribution. Its efficiency strongly depends on the proposal distribution used to build the chain. The best jump proposal is the one that closely resembles the unknown target distribution; therefore, we suggest an adaptive proposal distribution based on kernel density estimation (KDE). We group the model's parameters according to their correlation and build a KDE based on the already accepted points for each group. We update the KDE-based proposal until it stabilizes. We argue that such a proposal distribution could be efficient in applications where the data volume is increasing. We tested it on several astrophysical datasets (IPTA and LISA) and have shown that, in some cases, the KDE-based proposal also helps to reduce the chains' autocorrelation length. The efficiency of this proposal distribution is reduced in case of strong correlations between a large group of parameters.
更多查看译文
关键词
gravitational wave data analysis,estimation
AI 理解论文
溯源树
样例
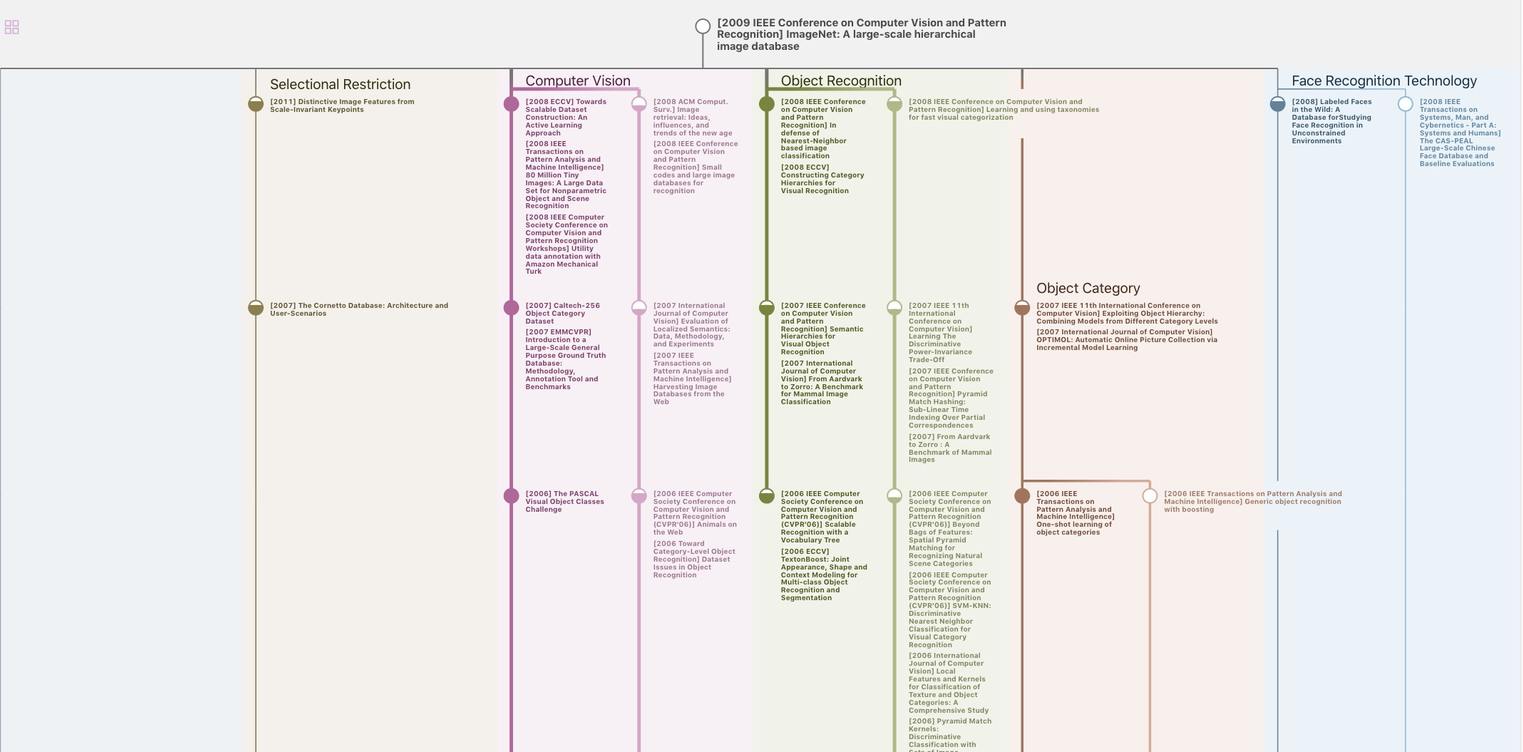
生成溯源树,研究论文发展脉络
Chat Paper
正在生成论文摘要