Circulating Tumor DNA As a Cancer Biomarker: an Overview of Biological Features and Factors That May Impact on Ctdna Analysis.
Frontiers in oncology(2022)SCI 3区
Biomed Sci Res Inst Puerta Hierro Majadahonda | Atrys Hlth
Abstract
Cancer cells release nucleic acids, freely or associated with other structures such as vesicles into body fluids, including blood. Among these nucleic acids, circulating tumor DNA (ctDNA) has emerged as a minimally invasive biomarker for tumor molecular profiling. However, certain biological characteristics of ctDNA are still unknown. Here, we provide an overview of the current knowledge about ctDNA biological features, including size and structure as well as the mechanisms of ctDNA shedding and clearance, and the physio-pathological factors that determine ctDNA levels. A better understanding of ctDNA biology is essential for the development of new methods that enable the analysis of ctDNA.
MoreTranslated text
Key words
ctDNA= circulating tumor DNA,ctDNA kinetics,biomarker,liquid biopsy,monitoring
求助PDF
上传PDF
View via Publisher
AI Read Science
AI Summary
AI Summary is the key point extracted automatically understanding the full text of the paper, including the background, methods, results, conclusions, icons and other key content, so that you can get the outline of the paper at a glance.
Example
Background
Key content
Introduction
Methods
Results
Related work
Fund
Key content
- Pretraining has recently greatly promoted the development of natural language processing (NLP)
- We show that M6 outperforms the baselines in multimodal downstream tasks, and the large M6 with 10 parameters can reach a better performance
- We propose a method called M6 that is able to process information of multiple modalities and perform both single-modal and cross-modal understanding and generation
- The model is scaled to large model with 10 billion parameters with sophisticated deployment, and the 10 -parameter M6-large is the largest pretrained model in Chinese
- Experimental results show that our proposed M6 outperforms the baseline in a number of downstream tasks concerning both single modality and multiple modalities We will continue the pretraining of extremely large models by increasing data to explore the limit of its performance
Upload PDF to Generate Summary
Must-Reading Tree
Example
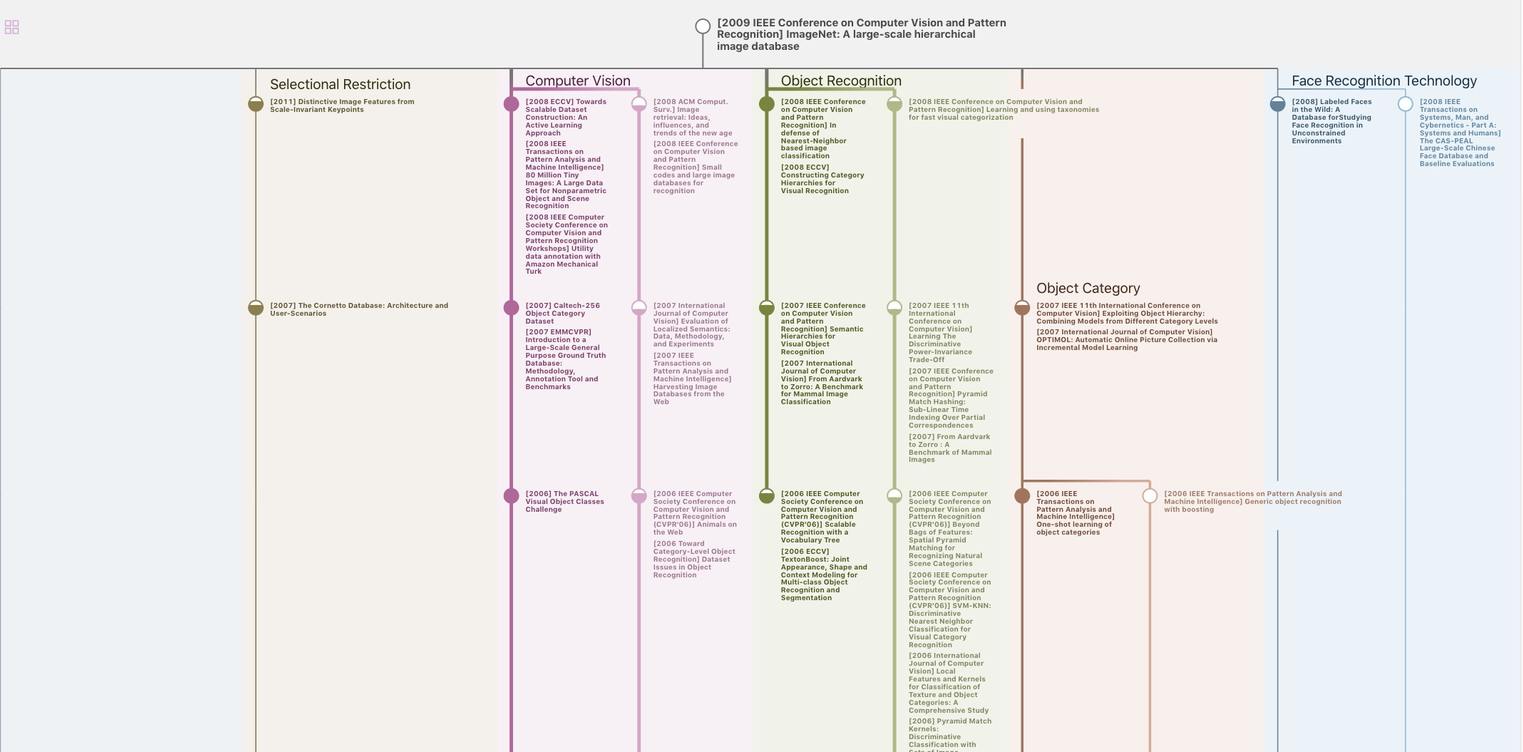
Generate MRT to find the research sequence of this paper
Related Papers
2004
被引用105 | 浏览
1983
被引用89 | 浏览
2007
被引用41 | 浏览
2005
被引用1522 | 浏览
2016
被引用1239 | 浏览
Cell-free DNA and Next-Generation Sequencing in the Service of Personalized Medicine for Lung Cancer
2016
被引用65 | 浏览
2017
被引用672 | 浏览
2018
被引用277 | 浏览
2018
被引用326 | 浏览
2016
被引用31 | 浏览
2019
被引用20 | 浏览
2020
被引用194 | 浏览
2020
被引用28 | 浏览
2020
被引用88 | 浏览
2020
被引用14 | 浏览
Data Disclaimer
The page data are from open Internet sources, cooperative publishers and automatic analysis results through AI technology. We do not make any commitments and guarantees for the validity, accuracy, correctness, reliability, completeness and timeliness of the page data. If you have any questions, please contact us by email: report@aminer.cn
Chat Paper