Entity Resolution with Hierarchical Graph Attention Networks
PROCEEDINGS OF THE 2022 INTERNATIONAL CONFERENCE ON MANAGEMENT OF DATA (SIGMOD '22)(2022)
摘要
Entity Resolution (ER) links entities that refer to the same real-world entity from different sources. Existing work usually takes pairs of entities as input and judges those pairs independently. However, there is often interdependence between different pairs of ER decisions, e.g., the entities from the same data source are usually semantically related to each other. Furthermore, current ER approaches are mainly based on attribute similarity comparison, but ignore interdependence between attributes. To address the limits of existing methods, we propose HierGAT, a new method for ER based on a Hierarchical Graph Attention Transformer Network, which can model and exploit the interdependence between different ER decisions. The benefit of our method comes from: 1) The graph attention network model for joint ER decisions; 2) The graph-attention capability to identify the discriminative words from attributes and find the most discriminative attributes. Furthermore, we propose to learn contextual embeddings to enrich word embeddings for better performance. The experimental results on benchmark datasets show that HierGAT outperforms DeepMatcher by up to 32.5% of F1 score and up to 8.7% of F1 score compared with Ditto.
更多查看译文
关键词
entity resolution, collective entity linking, hierarchical heterogeneous graph, graph attention networks
AI 理解论文
溯源树
样例
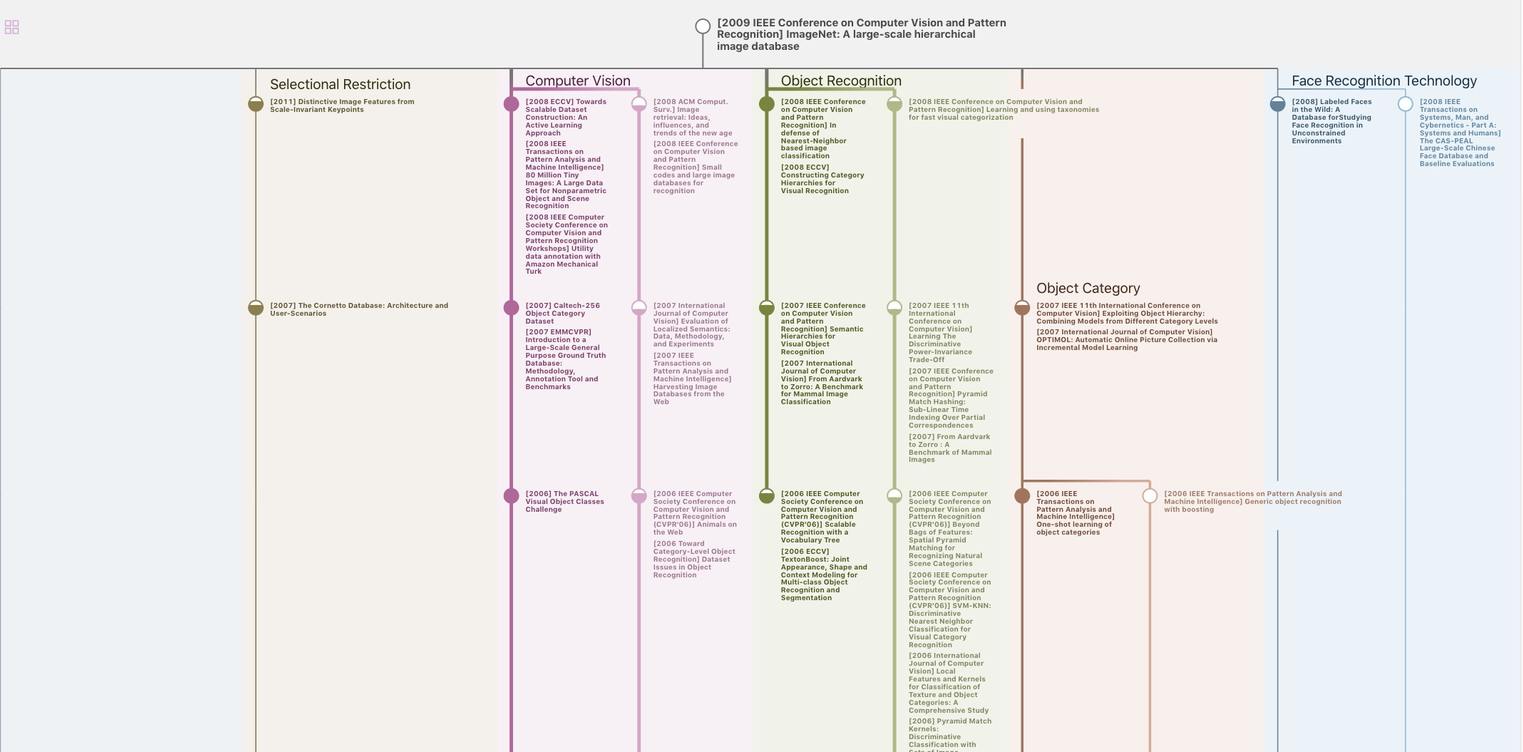
生成溯源树,研究论文发展脉络
Chat Paper
正在生成论文摘要