KDE-GAN
Proceedings of the Genetic and Evolutionary Computation Conference Companion(2022)
摘要
Generative Adversarial Networks (GAN) is a powerful algorithm to reconstruct artificial data that is similar to a set of given data. Evolutionary GAN (E-GAN) is a state-of-the-art variant of GAN. E-GAN combines evolutionary elements such as mutation, fitness evaluation and selection to address vanishing gradient and mode collapse problems of classic GAN. This paper further improves E-GAN by proposing Knowledge Distillation and Knowledge Transfer. The new method, known as Knowledge Distillation E-GAN (KDE-GAN), incorporates the student-teacher architecture and fine-tuning of transfer learning to help the evolutionary GAN training process. Our experiments on benchmark data sets show that KDE-GAN can improve performance and efficiency. Knowledge distillation and knowledge transfer can indeed accelerate the learning process yet reach better FIDs (Frechet Inception Distance).
更多查看译文
AI 理解论文
溯源树
样例
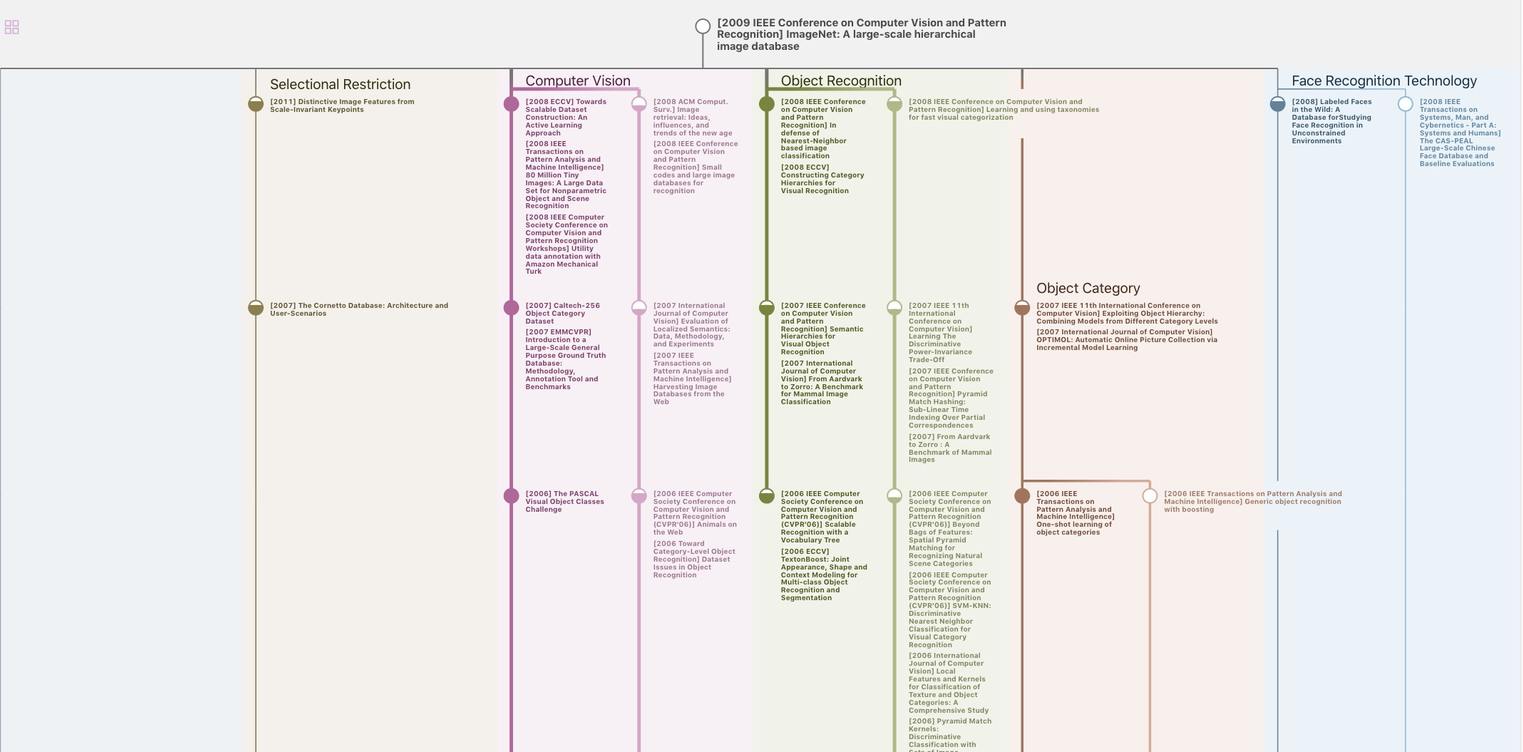
生成溯源树,研究论文发展脉络
Chat Paper
正在生成论文摘要