A Self-Training Teacher-Student Model with an Automatic Label Grader for Abdominal Skeletal Muscle Segmentation
ARTIFICIAL INTELLIGENCE IN MEDICINE(2022)
Key words
Image segmentation,Semi-supervised learning,Self-attention,Teacher-student model,Skeletal muscle
AI Read Science
Must-Reading Tree
Example
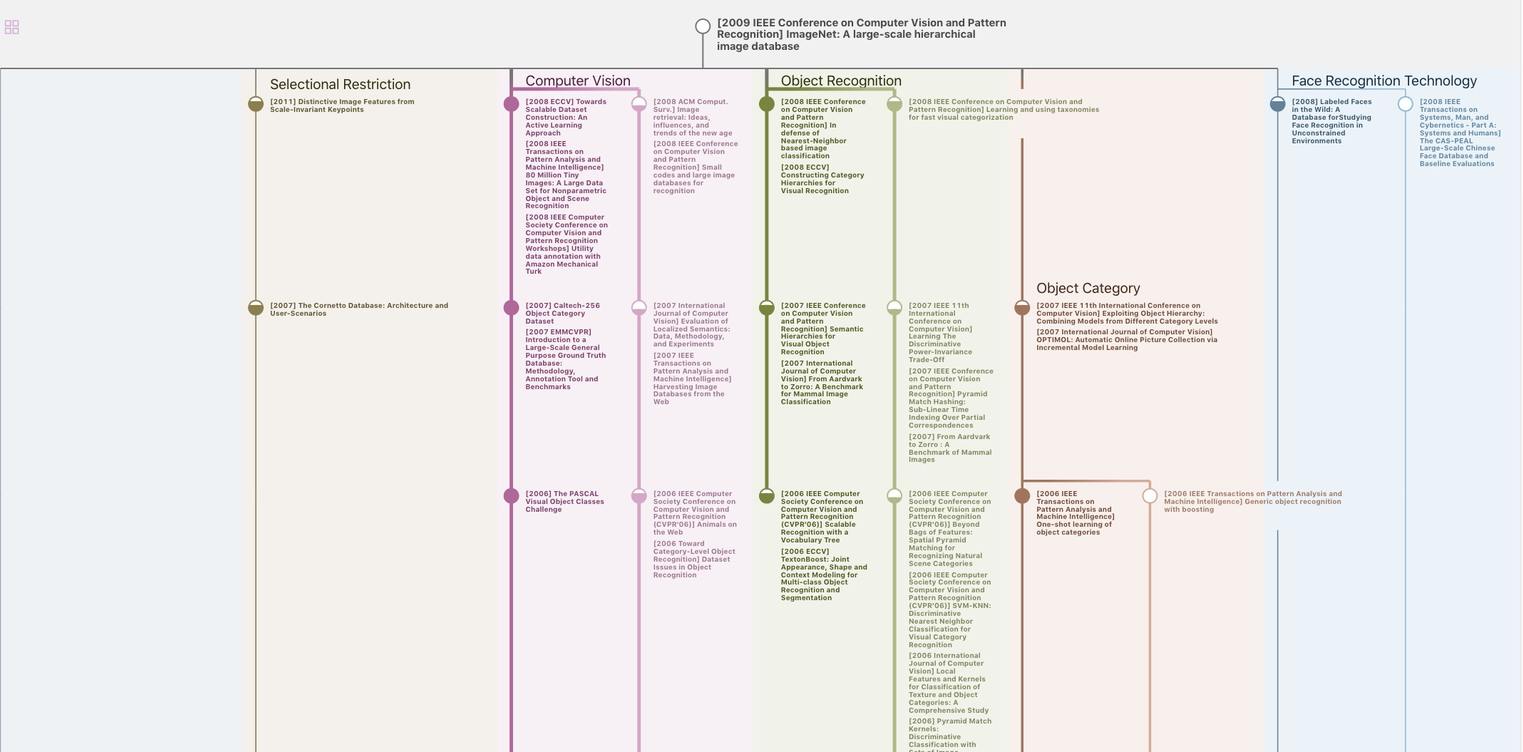
Generate MRT to find the research sequence of this paper
Chat Paper
Summary is being generated by the instructions you defined