Self-Supervision Can Be a Good Few-Shot Learner.
Computing Research Repository (CoRR)(2022)
University of Science and Technology of China | Huawei Noah’s Ark Lab
Abstract
Existing few-shot learning (FSL) methods rely on training with a large labeled dataset, which prevents them from leveraging abundant unlabeled data. From an information-theoretic perspective, we propose an effective unsupervised FSL method, learning representations with self-supervision. Following the InfoMax principle, our method learns comprehensive representations by capturing the intrinsic structure of the data. Specifically, we maximize the mutual information (MI) of instances and their representations with a low-bias MI estimator to perform self-supervised pre-training. Rather than supervised pre-training focusing on the discriminable features of the seen classes, our self-supervised model has less bias toward the seen classes, resulting in better generalization for unseen classes. We explain that supervised pre-training and self-supervised pre-training are actually maximizing different MI objectives. Extensive experiments are further conducted to analyze their FSL performance with various training settings. Surprisingly, the results show that self-supervised pre-training can outperform supervised pre-training under the appropriate conditions. Compared with state-of-the-art FSL methods, our approach achieves comparable performance on widely used FSL benchmarks without any labels of the base classes.
MoreTranslated text
Key words
Few-shot image classification,Self-supervised learning
PDF
View via Publisher
AI Read Science
AI Summary
AI Summary is the key point extracted automatically understanding the full text of the paper, including the background, methods, results, conclusions, icons and other key content, so that you can get the outline of the paper at a glance.
Example
Background
Key content
Introduction
Methods
Results
Related work
Fund
Key content
- Pretraining has recently greatly promoted the development of natural language processing (NLP)
- We show that M6 outperforms the baselines in multimodal downstream tasks, and the large M6 with 10 parameters can reach a better performance
- We propose a method called M6 that is able to process information of multiple modalities and perform both single-modal and cross-modal understanding and generation
- The model is scaled to large model with 10 billion parameters with sophisticated deployment, and the 10 -parameter M6-large is the largest pretrained model in Chinese
- Experimental results show that our proposed M6 outperforms the baseline in a number of downstream tasks concerning both single modality and multiple modalities We will continue the pretraining of extremely large models by increasing data to explore the limit of its performance
Try using models to generate summary,it takes about 60s
Must-Reading Tree
Example
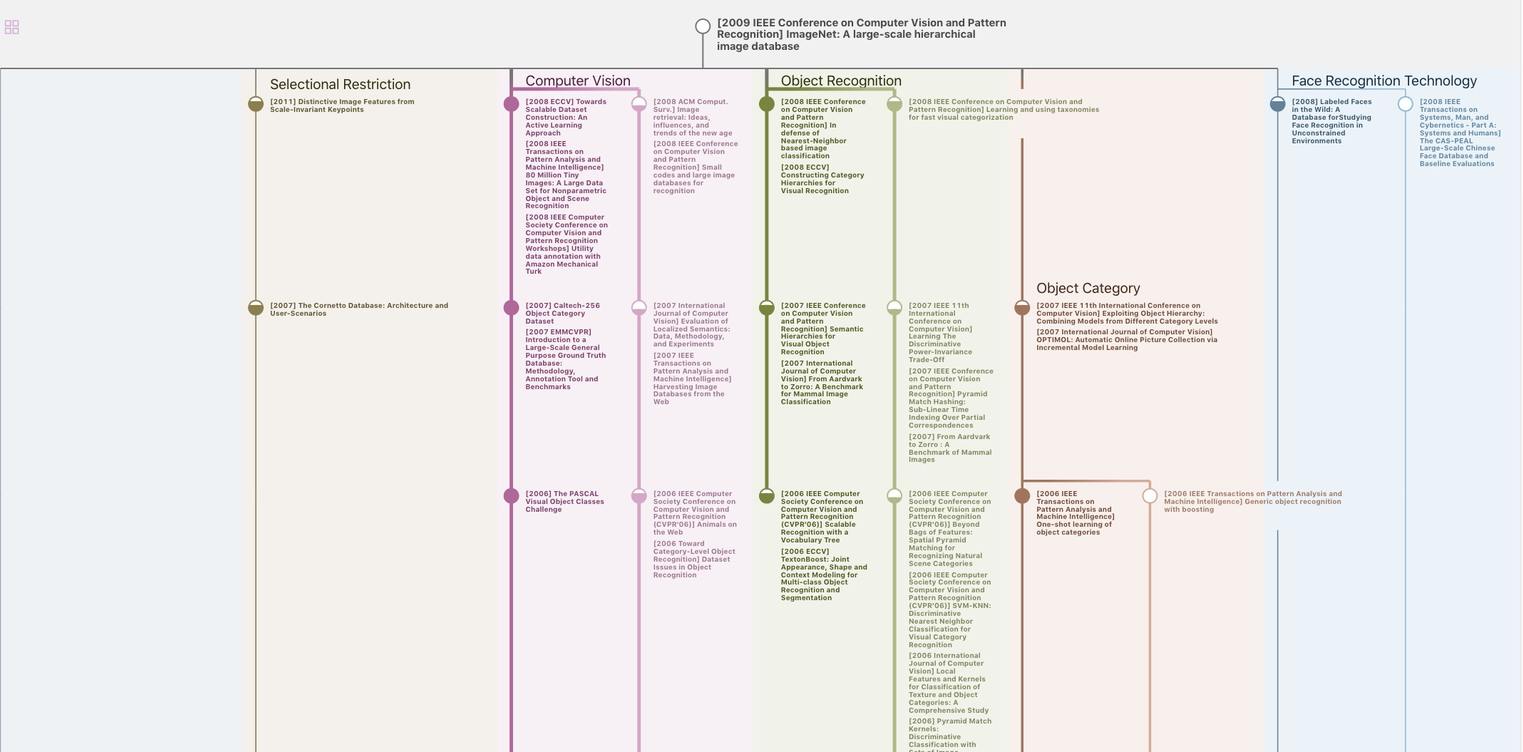
Generate MRT to find the research sequence of this paper
Related Papers
IEEE Transactions on Pattern Analysis and Machine Intelligence 2006
被引用1551 | 浏览
Data Disclaimer
The page data are from open Internet sources, cooperative publishers and automatic analysis results through AI technology. We do not make any commitments and guarantees for the validity, accuracy, correctness, reliability, completeness and timeliness of the page data. If you have any questions, please contact us by email: report@aminer.cn
Chat Paper
去 AI 文献库 对话