Attention Based Large Scale Multi-agent Reinforcement Learning
2022 5th International Conference on Artificial Intelligence and Big Data (ICAIBD)(2022)
摘要
Learning in large scale Multi-Agent Reinforcement Learning is fundamentally difficult due to the curse of dimensionality. In homogeneous multi-agent setting, mean field theory provides an effective way of scaling MARL to environments with many agents by abstracting other agents to a virtual mean agent, which assumes the impact of each player on the outcome is equal and infinitesimal. However, in some real scenarios, it is only several neighboring agents that affect the decision-making of an agent, need not all other agents. In addition, different neighboring agents may have different degrees of influence on the decision-making of an agent. In this paper, not restricted to homogeneous setting, we propose Adaptive Mean Field Multi-Agent Reinforcement Learning (AMF-MARL), which is based on the attention mechanism and can be used to deal with many agent scenarios in which there may be different influence relationships among agents. Specifically, we firstly derive the mean field approximation with adaptive weight. Then, we propose the Adaptive Mean Field Q-learning (AMF-Q) approach, and describe how to obtain the adaptive weight. Finally, we conduct experiment to study the learning effectiveness of proposed approach.
更多查看译文
关键词
large scale,multi-agent reinforcement learning,adaptive mean field approximation
AI 理解论文
溯源树
样例
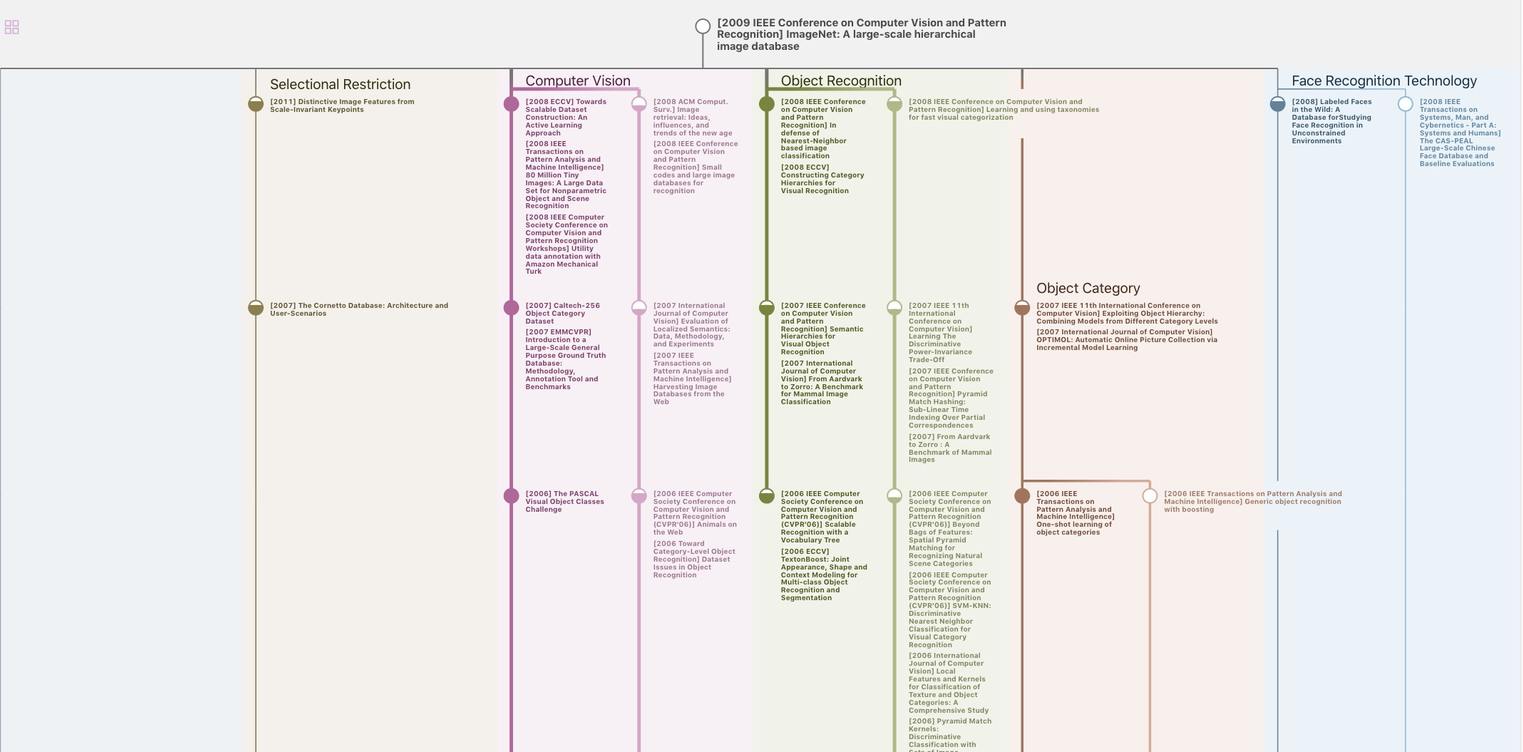
生成溯源树,研究论文发展脉络
Chat Paper
正在生成论文摘要