The ASTRI-Horn Cherenkov Camera: Improvements on the Hardware and Software Components
Advances in Optical and Mechanical Technologies for Telescopes and Instrumentation V(2022)
Abstract
ASTRI-Horn is an imaging atmospheric Cherenkov telescope developed by the Italian National Institute for Astrophysics (INAF), installed at the Serra La Nave Astronomical Station on Mount Etna (Italy). ASTRI-Horn detected the Crab proving the validity of its innovative camera and of the dual mirror configuration. Henceforth the telescope will play another important role. It will be the test bench for the upcoming cameras to be adopted for the ASTRI Mini-Array, a project led by INAF to build and operate an array of nine Cherenkov telescopes at the Observatorio del Teide (Tenerife, Spain). Moreover, the ASTRI-Horn camera will be used to test new technological solutions and explore innovative Cherenkov observation techniques. The Cherenkov camera uses Silicon-Photo Multiplier (SiPM) detectors. The fast front-end electronics implemented in the CITIROC ASIC is based on a custom peak-detector mode, which measures the electric pulses generated by the Cherenkov light flashes. The compact camera embeds all the components of a reliable thermal cooling system. This contribution gives a description of the upgrades of the ASTRI-Horn camera, which are the results of the lesson learnt during these years of sky observations. The improvements aim at correcting the drawbacks detected so far and at increasing the overall performance of the camera. The main ones are the increment of the power supplied to the photodetectors, the redesign of the Lids kinematic chain, a more efficient embedded calibration system, new control software routines and GUI.
MoreTranslated text
求助PDF
上传PDF
View via Publisher
AI Read Science
AI Summary
AI Summary is the key point extracted automatically understanding the full text of the paper, including the background, methods, results, conclusions, icons and other key content, so that you can get the outline of the paper at a glance.
Example
Background
Key content
Introduction
Methods
Results
Related work
Fund
Key content
- Pretraining has recently greatly promoted the development of natural language processing (NLP)
- We show that M6 outperforms the baselines in multimodal downstream tasks, and the large M6 with 10 parameters can reach a better performance
- We propose a method called M6 that is able to process information of multiple modalities and perform both single-modal and cross-modal understanding and generation
- The model is scaled to large model with 10 billion parameters with sophisticated deployment, and the 10 -parameter M6-large is the largest pretrained model in Chinese
- Experimental results show that our proposed M6 outperforms the baseline in a number of downstream tasks concerning both single modality and multiple modalities We will continue the pretraining of extremely large models by increasing data to explore the limit of its performance
Upload PDF to Generate Summary
Must-Reading Tree
Example
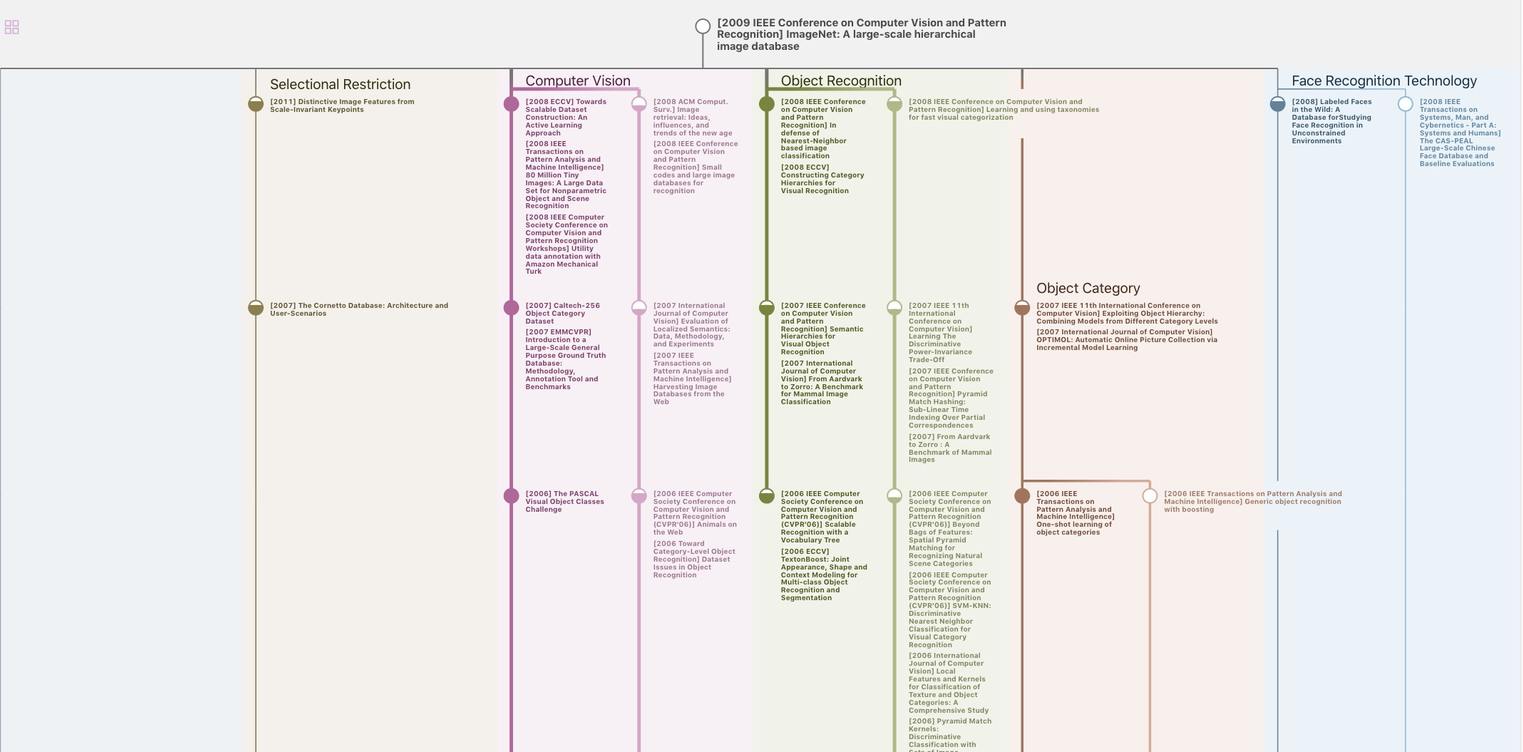
Generate MRT to find the research sequence of this paper
Related Papers
Data Disclaimer
The page data are from open Internet sources, cooperative publishers and automatic analysis results through AI technology. We do not make any commitments and guarantees for the validity, accuracy, correctness, reliability, completeness and timeliness of the page data. If you have any questions, please contact us by email: report@aminer.cn
Chat Paper
GPU is busy, summary generation fails
Rerequest