Semi-supervised Image Deraining Using Knowledge Distillation
IEEE Transactions on Circuits and Systems for Video Technology(2022)
摘要
Image deraining has achieved considerable progress based on supervised learning with synthetic training pairs, but is usually limited in handling real-world rainy images. Although semi-supervised methods are suggested to exploit real-world rainy images when training deep deraining models, their performances are still notably inferior. To address this crucial issue, this work proposes a semi-supervised image deraining network with knowledge distillation (SSID-KD) for better exploiting real-world rainy images. In particular, the consistency of feature distribution of rain streaks extracted from synthetic and real-world rainy images is enforced by adopting knowledge distillation. Moreover, as for the backbone in SSID-KD, we propose the multi-scale feature fusion module and the pyramid fusion module to better extract deep features of rainy images. SSID-KD can relieve the problem of over-deraining or under-deraining for real-world rainy images, while it can keep comparable performance with supervised deraining methods on several benchmark datasets. Extensive experiments on both synthetic and real-world rainy images have validated that our SSID-KD not only can achieve better deraining results than existing semi-supervised deraining methods but also are quantitatively comparable with state-of-the-art supervised deraining methods. Benefiting from the well exploration of real-world rainy images, our SSID-KD can obtain more visually plausible deraining results. The source code and trained models are publicly available at
https://github.com/cuiyixin555/SSID-KD
.
更多查看译文
关键词
Single image deraining,knowledge distillation,semi-supervised learning
AI 理解论文
溯源树
样例
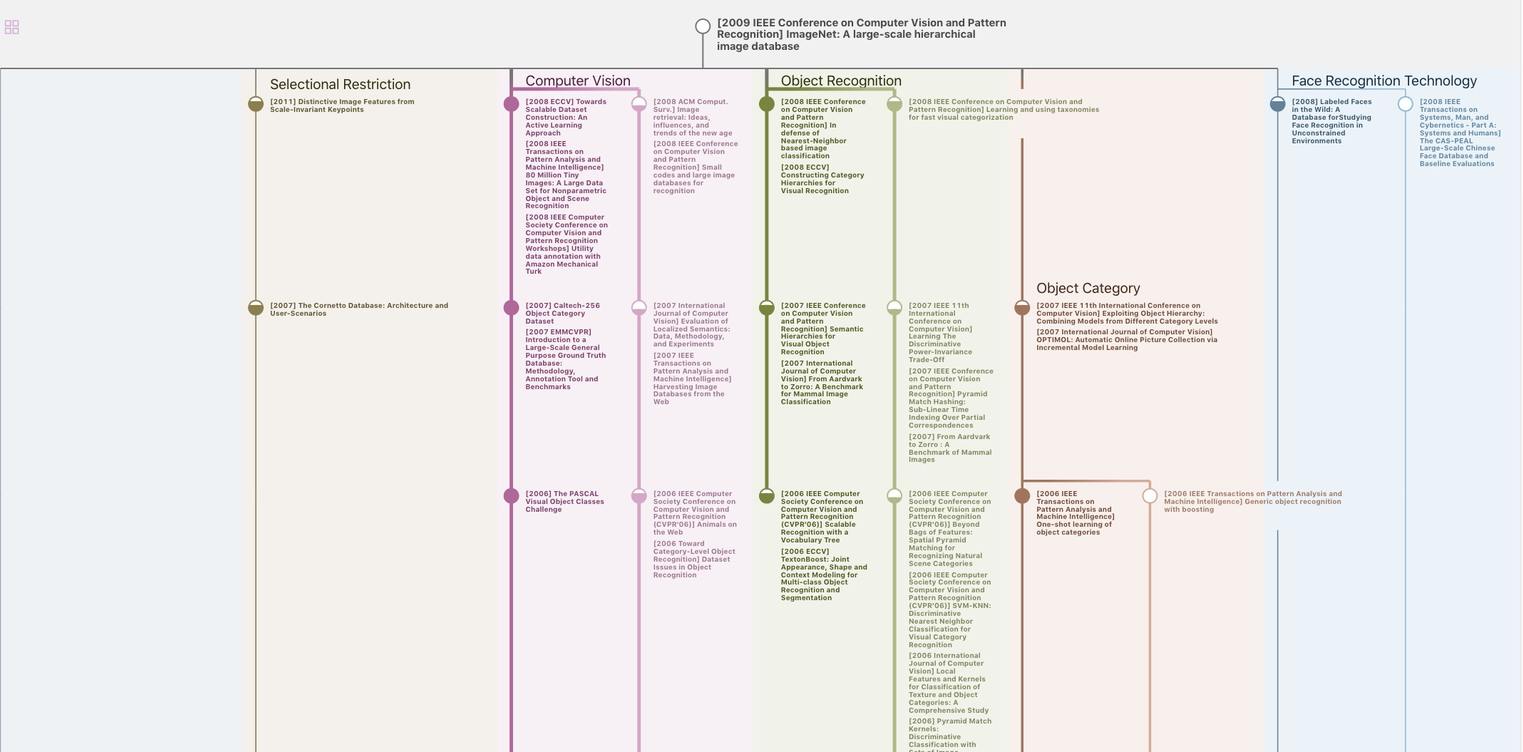
生成溯源树,研究论文发展脉络
Chat Paper
正在生成论文摘要