Weighted mean field reinforcement learning for large-scale UAV swarm confrontation
Applied Intelligence(2022)
摘要
Finding the optimal game strategy is a difficult problem in unmanned aerial vehicle (UAV) swarm confrontation. As an effective solution to the sequential decision-making problem, multi-agent reinforcement learning (MARL) provides a promising way to realize intelligent countermeasures. However, there are two challenges in applying MARL to large-scale UAV swarm confrontation: i) the curse of dimensionality caused by the excessive scale of UAV clusters and ii) the generalization problem caused by the dynamically changing UAV cluster size. To address these problems, we propose a novel MARL paradigm, called Weighted Mean Field Reinforcement Learning , where the pairwise communication between any UAV and its neighbors is modeled as that between a central UAV and the virtual UAV, which is abstracted from the weighted mean effect of neighboring UAVs. This approach reduces the multi-agent problem to a two-agent problem, which can reduce the input dimension of the agent and adapt to the changing cluster size. The communication content between UAVs includes actions and local observations. Actions can enhance the cooperation between UAVs and alleviate the non-stationarity of the environment, while local observations can expand the perception range of the central UAV so that it can obtain more useful information about the environment. The attention mechanism is leveraged to enable UAVs to select more valuable information flexibly, making our method more scalable than other algorithms. Combining this paradigm with double Q-learning and actor-critic algorithms, we propose weighted mean field Q-learning (WMFQ) and weighted mean field actor-critic (WMFAC) algorithms. Experiments on our constructed UAV swarm confrontation environment verify the effectiveness and scalability of our algorithms.
更多查看译文
关键词
Multi-agent reinforcement learning,Unmanned aerial vehicle,Swarm confrontation,Attention mechanism
AI 理解论文
溯源树
样例
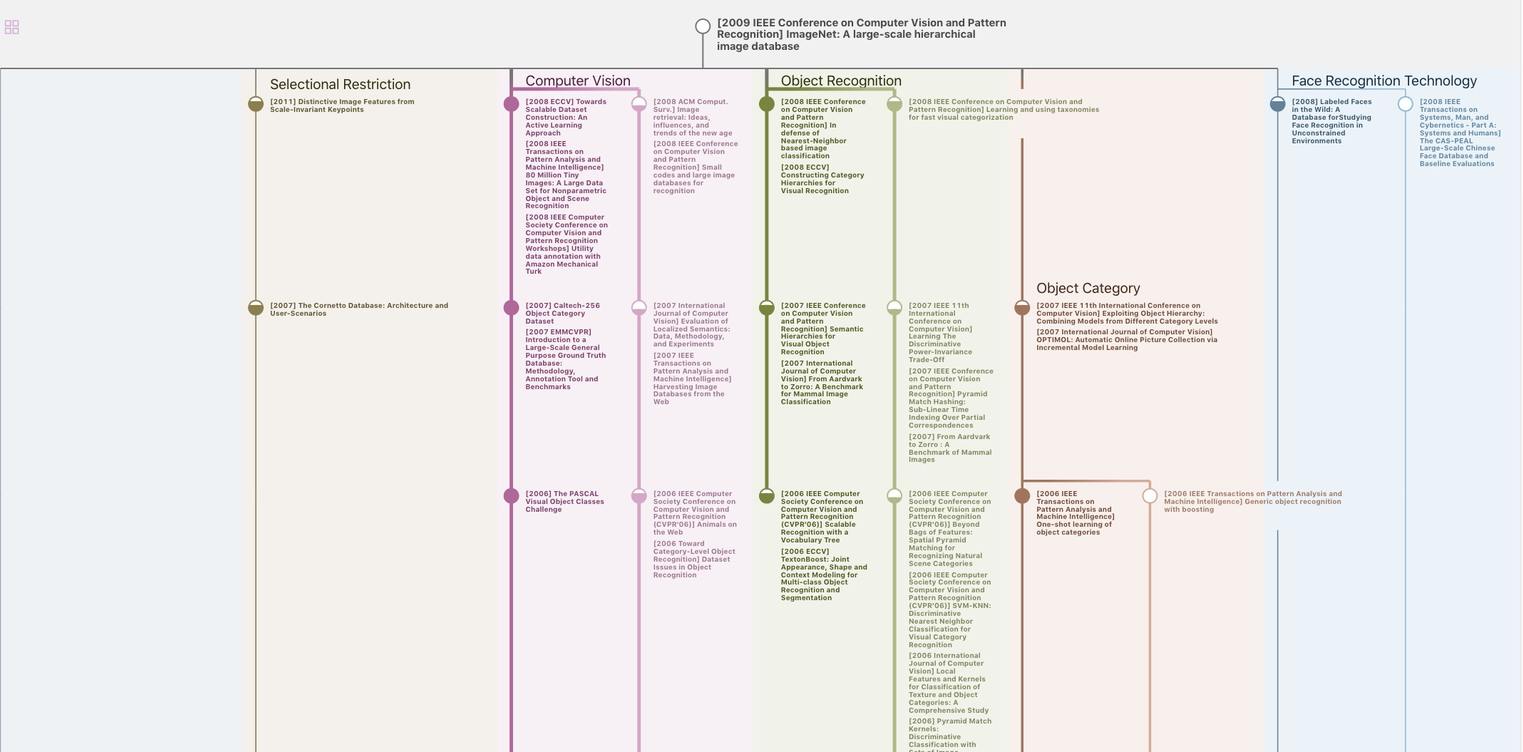
生成溯源树,研究论文发展脉络
Chat Paper
正在生成论文摘要