Few-shot Driver Identification Via Meta-Learning.
Expert systems with applications(2022)
摘要
Driver identification in connected transportation is useful for usage-based insurance, personalized assisted driving, fleet management etc. Capturing the driving style from data behind the wheel benefits such applications without requiring extra costs and offending drivers’ biometric fingerprint privacy (e.g., facial recognition). However, the driver group to be identified may change, which leads to poor learning ability in a method based on depth representations of driving styles for new drivers and produces a sharp decline in generalization ability. Meta-learning is an exciting subfield of machine learning that equips deep learning models with the ability to learn, especially when the given data sample is very limited. This paper addresses a distinctively novel driver identification problem, where the prior model is supposed to quickly adapt to varying numbers of drivers, especially when few examples are available. First, based on a public driving dataset, a set of training and testing tasks is specifically designed for few-shot driver identification. Then, based on the popular model-agnostic meta-learning (MAML) framework, a feature autoencoder regularized learner is proposed to avoid the commonly encountered memorization problem and improve the generalization ability of the identification model. Three versions of meta-models are derived concerning computation and classification effectiveness. Finally, the experimental results show that the proposed method is superior to the previous baselines.
更多查看译文
关键词
Driver identification,Driving style,Meta-learning,Few-shot learning
AI 理解论文
溯源树
样例
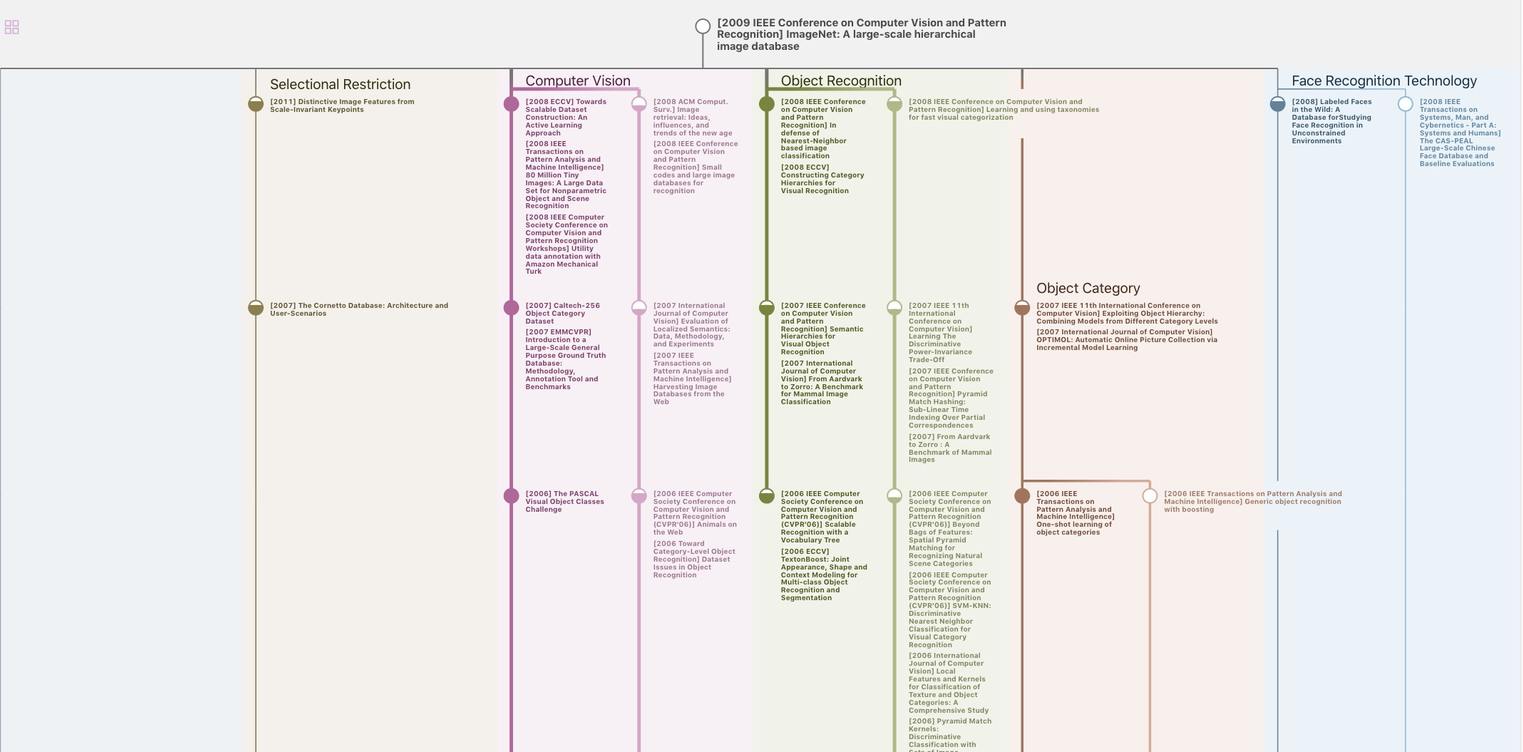
生成溯源树,研究论文发展脉络
Chat Paper
正在生成论文摘要