Convolutional Neural Network for Measurement of Suspended Solids and Turbidity
APPLIED SCIENCES-BASEL(2022)
摘要
The great potential of the convolutional neural networks (CNNs) provides novel and alternative ways to monitor important parameters with high accuracy. In this study, we developed a soft sensor model for dynamic processes based on a CNN for the measurement of suspended solids and turbidity from a single image of the liquid sample to be measured by using a commercial smartphone camera (Android or IOS system) and light-emitting diode (LED) illumination. For this, an image dataset of liquid samples illuminated with white, red, green, and blue LED light was taken and used to train a CNN and fit a multiple linear regression (MLR) by using different color lighting, we evaluated which color gives more accurate information about the concentration of suspended particles in the sample. We implemented a pre-trained AlexNet model, and an MLR to estimate total suspended solids (TSS), and turbidity values in liquid samples based on suspended particles. The proposed technique obtained high goodness of fit (R-2 = 0.99). The best performance was achieved using white light, with an accuracy of 98.24% and 97.20% for TSS and turbidity, respectively, with an operational range of 0-800 mgL(-1), and 0-306 NTU. This system was designed for aquaculture environments and tested with both commercial fish feed and paprika. This motivates further research with different aquatic environments such as river water, domestic and industrial wastewater, and potable water, among others.
更多查看译文
关键词
computer vision for measurements, convolutional neural network (CNN), transfer learning, total suspended solids (TSS), turbidity
AI 理解论文
溯源树
样例
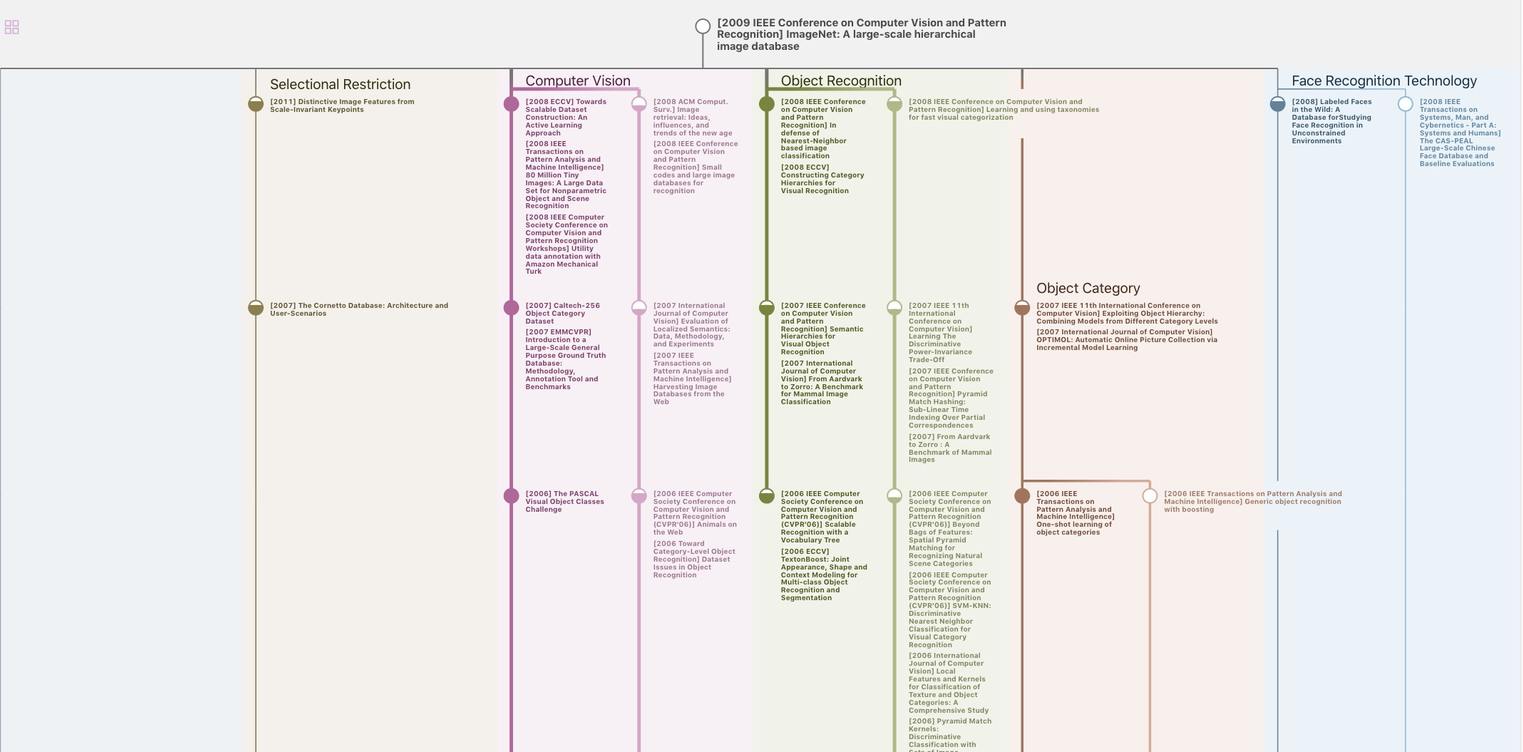
生成溯源树,研究论文发展脉络
Chat Paper
正在生成论文摘要