Data Imputation for Multivariate Time Series Sensor Data with Large Gaps of Missing Data
IEEE sensors journal(2022)
摘要
Imputation of missing sensor-collected data is often an important step prior to machine learning and statistical data analysis. One particular data imputation challenge is filling large data gaps when the only related data comes from the same sensor station. In this paper, we propose a framework to improve the popular multivariate imputation by chained equations (MICE) method for dealing with missing data. One key strategy we use to improve model accuracy is to reshape the original sensor data to leverage the correlation between the missing data and the observed data. We demonstrate our framework using data from continuous water quality monitoring stations in Vermont. Because of possible irregularly spaced peaks throughout the time series, the reshaped data is split into extreme and normal values and two MICE models are built. We also recommend that sensor-collected data should be transformed to meet the machine learning model assumptions. According to our experimental results, these strategies can improve MICE data imputation model accuracy at least 23% for large data gaps based on $\text {R}^{{2}}$ values and are promising to be applied for other data imputation algorithms.
更多查看译文
关键词
Data imputation,large missing data gap,MICE,multivariate,time series
AI 理解论文
溯源树
样例
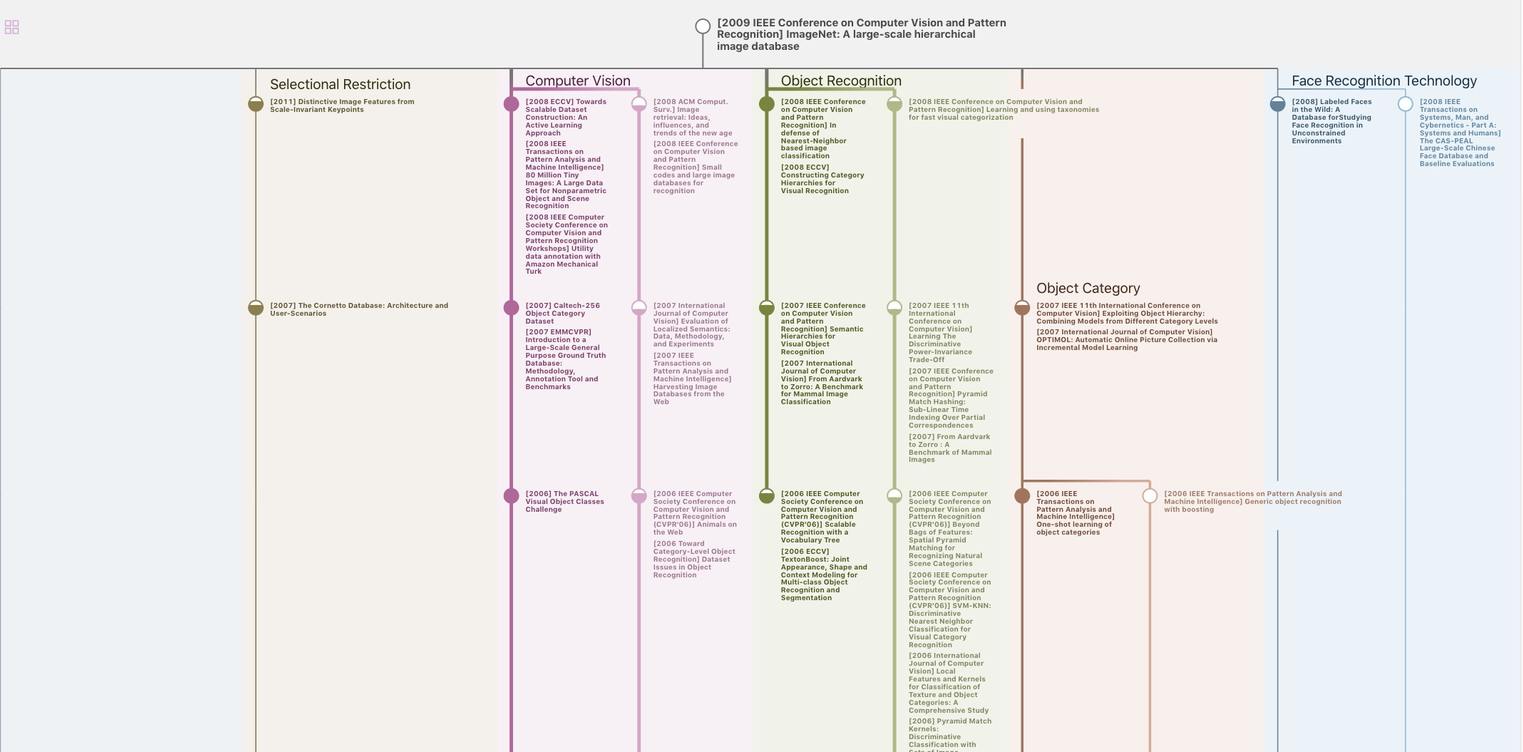
生成溯源树,研究论文发展脉络
Chat Paper
正在生成论文摘要