Robust Multi-Label Classification with Enhanced Global and Local Label Correlation
MATHEMATICS(2022)
摘要
Data representation is of significant importance in minimizing multi-label ambiguity. While most researchers intensively investigate label correlation, the research on enhancing model robustness is preliminary. Low-quality data is one of the main reasons that model robustness degrades. Aiming at the cases with noisy features and missing labels, we develop a novel method called robust global and local label correlation (RGLC). In this model, subspace learning reconstructs intrinsic latent features immune from feature noise. The manifold learning ensures that outputs obtained by matrix factorization are similar in the low-rank latent label if the latent features are similar. We examine the co-occurrence of global and local label correlation with the constructed latent features and the latent labels. Extensive experiments demonstrate that the classification performance with integrated information is statistically superior over a collection of state-of-the-art approaches across numerous domains. Additionally, the proposed model shows promising performance on multi-label when noisy features and missing labels occur, demonstrating the robustness of multi-label classification.
更多查看译文
关键词
multi-label classification,label correlations,noisy features,missing labels,robustness
AI 理解论文
溯源树
样例
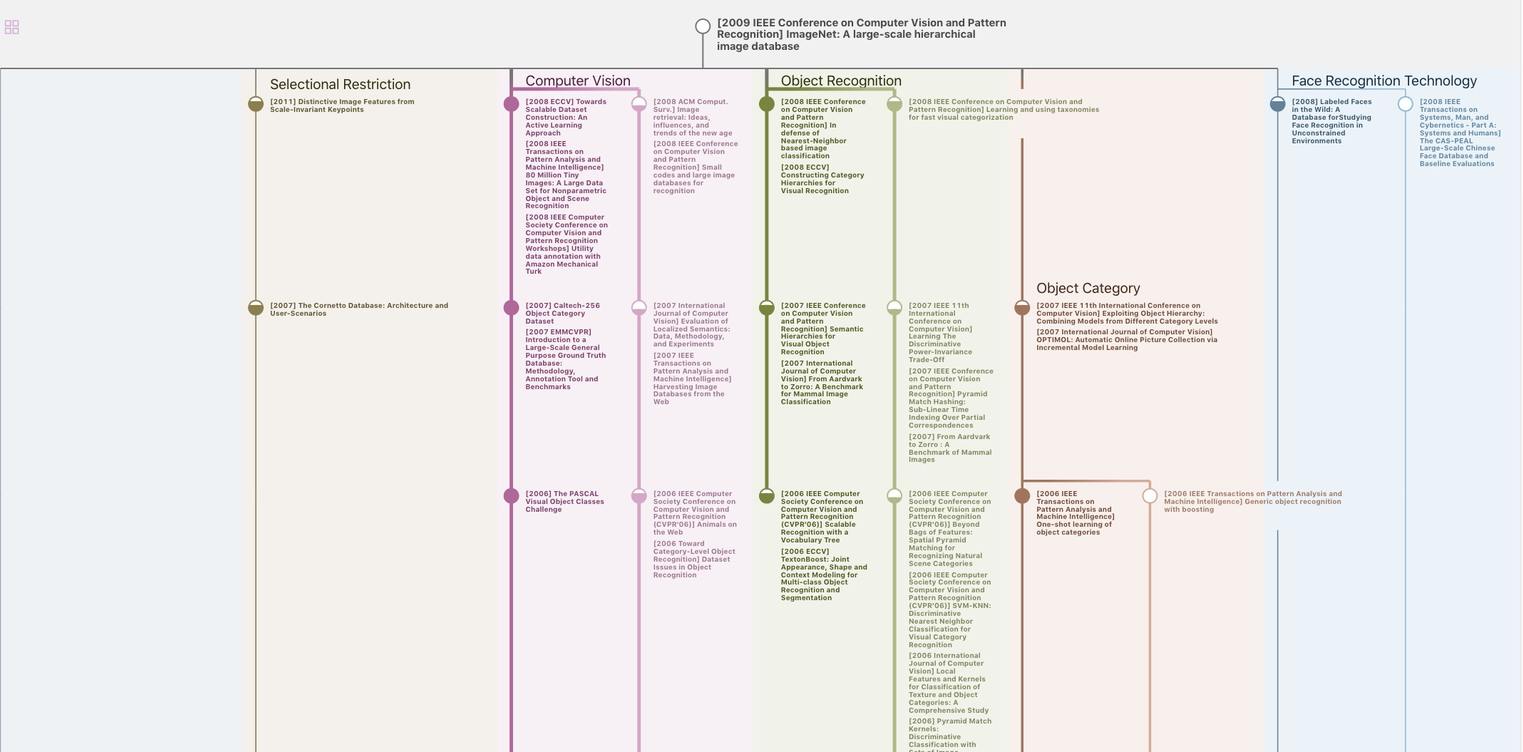
生成溯源树,研究论文发展脉络
Chat Paper
正在生成论文摘要