CGTF: Convolution-Guided Transformer for Infrared and Visible Image Fusion
IEEE TRANSACTIONS ON INSTRUMENTATION AND MEASUREMENT(2022)
摘要
Deep learning has been successfully applied to infrared and visible image fusion due to its powerful ability of feature representation. Existing most deep learning-based infrared and visible image fusion methods mainly utilize pure convolution model or pure transformer model, which leads to that the fused image cannot preserve long-range dependences (global context) and local features simultaneously. To this end, we propose a convolution-guided transformer framework for infrared and visible image fusion (CGTF), which aims to combine the local features of convolutional network and the long-range dependence features of transformer to produce satisfactory fused image. In CGTF, the local features are calculated by convolution feature extraction module (CFEM), and then, the local features are used to guide the transformer feature extraction module (TFEM) to capture the long-range dependences of the image, which can overcome not only the lack of long-range dependences that exist in convolutional fusion methods but also the deficiency of local feature that exists in transformer models. Moreover, the convolution-guided transformer fusion framework can consider the inherent relationship of local feature and long-range dependences due to the alternate use of CFEM and transformer module. In addition, to strengthen local feature propagation, we employ dense connections among CFEMs. Ablation experiments demonstrate the effectiveness of convolution-guided transformer fusion framework and loss function. We employ two datasets to compare our method with other nine methods, which include three traditional methods, five deep learning-based methods, and one transformer-based method. Qualitative and quantitative experiments demonstrate the advantages of our method.
更多查看译文
关键词
Transformers,Feature extraction,Convolution,Image fusion,Fuses,Convolutional neural networks,Deep learning,Image fusion,infrared image,transformer,visible image
AI 理解论文
溯源树
样例
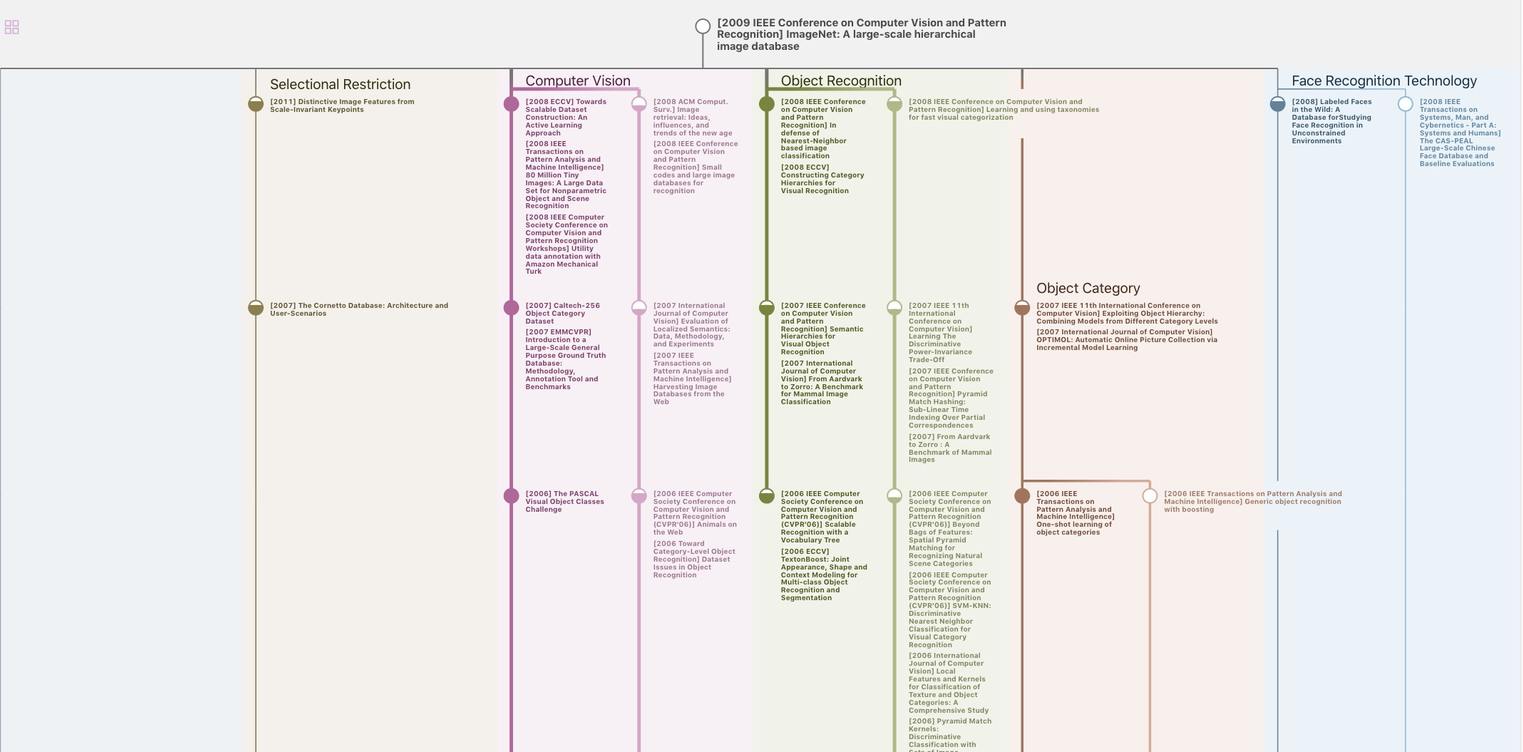
生成溯源树,研究论文发展脉络
Chat Paper
正在生成论文摘要