Productive Performance and Physicochemical Quality of Grapes for Processing Grown on Different Rootstocks
Pesquisa Agropecuaria Brasileira(2022)SCI 4区
Univ Estadual Paulista | Agencia Paulista Tecnol Agronegocio
Abstract
Abstract The objective of this work was to evaluate the productive performance and physicochemical characteristics of the Isabel Precoce, BRS Carmem, BRS Cora, and IAC 138-22 Máximo grape (Vitis labrusca) cultivars for juice grafted onto the 'IAC 572 Jales' and 'IAC 766 Campinas' rootstocks. A randomized complete block design, in a 4×2 factorial arrangement, was used. Productive performance was evaluated through: production per plant; yield; number of clusters per vine; and physical characteristics of clusters, berries, and rachis. The physicochemical characteristics of must composition were also determined through the content of soluble solids, reducing sugars, titratable acidity, maturity index, and pH. Since there was no significant interaction between cultivars and rootstocks for most of the evaluated characteristics, each factor was analyzed separately. The 'IAC 766 Campinas' rootstock provided a higher yield and more suitable physicochemical characteristics for the four cultivars. However, regardless of the rootstock used, cultivars BRS Carmem, IAC 138-22 Máximo, and Isabel Precoce were more productive than BRS Cora.
MoreTranslated text
Key words
Vitis labrusca,grape juice,hybrid grapes,tropical viticulture
PDF
View via Publisher
AI Read Science
AI Summary
AI Summary is the key point extracted automatically understanding the full text of the paper, including the background, methods, results, conclusions, icons and other key content, so that you can get the outline of the paper at a glance.
Example
Background
Key content
Introduction
Methods
Results
Related work
Fund
Key content
- Pretraining has recently greatly promoted the development of natural language processing (NLP)
- We show that M6 outperforms the baselines in multimodal downstream tasks, and the large M6 with 10 parameters can reach a better performance
- We propose a method called M6 that is able to process information of multiple modalities and perform both single-modal and cross-modal understanding and generation
- The model is scaled to large model with 10 billion parameters with sophisticated deployment, and the 10 -parameter M6-large is the largest pretrained model in Chinese
- Experimental results show that our proposed M6 outperforms the baseline in a number of downstream tasks concerning both single modality and multiple modalities We will continue the pretraining of extremely large models by increasing data to explore the limit of its performance
Try using models to generate summary,it takes about 60s
Must-Reading Tree
Example
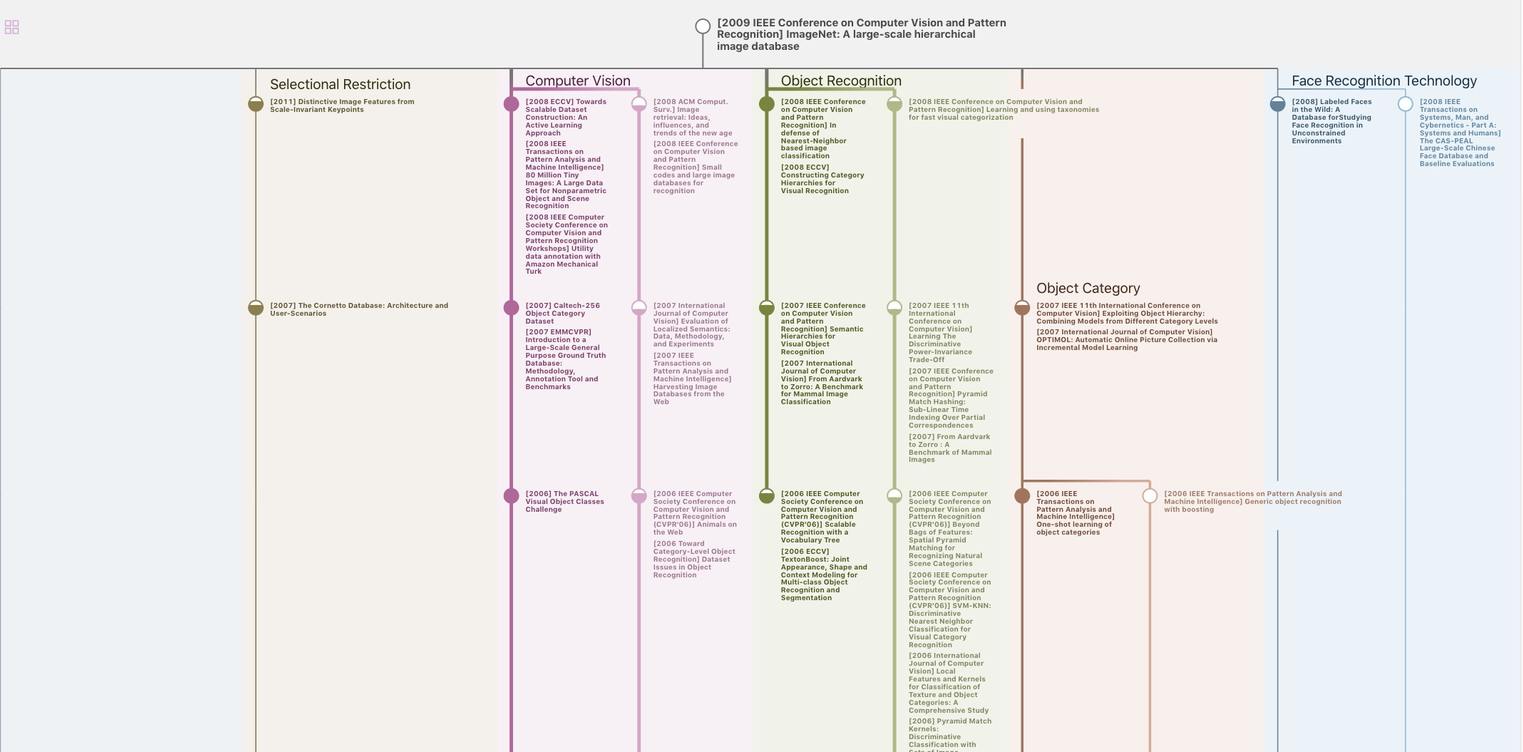
Generate MRT to find the research sequence of this paper
Related Papers
HORTICULTURAE 2023
被引用0
Data Disclaimer
The page data are from open Internet sources, cooperative publishers and automatic analysis results through AI technology. We do not make any commitments and guarantees for the validity, accuracy, correctness, reliability, completeness and timeliness of the page data. If you have any questions, please contact us by email: report@aminer.cn
Chat Paper
GPU is busy, summary generation fails
Rerequest