Unsteady flow prediction from sparse measurements by compressed sensing reduced order modeling
Computer methods in applied mechanics and engineering(2022)
摘要
Prediction of complex fluid flows from sparse and noisy sensor measurements is widely applied to many engineering fields. In the present study, a novel compressed sensing reduced-order modeling framework has been proposed to predict unsteady flow fields from sparse and noisy sensor observations, which includes offline learning and online forecasting. In the offline learning stage, the Long Short Term Memory (LSTM) model is used for modeling the dynamic evolution of the sensor signals. Moreover, the sparsity-promoting Dynamic Mode Decomposition (DMD) algorithm is applied to extract spatio-temporal coherent structures from high-dimensional fluid dynamic system and choose optimal DMD modes for flow reconstruction. In order to establish the correlation between sensor space and feature-based coherent structures space, the Deep Neural Network (DNN) is employed. In the online forecasting stage, the trained framework is applied to predict the flow fields from limited sensor measurements in the actual experiment setup. In the current work, the proposed framework is applied in two numerical examples, including the flow past a circle cylinder at Re = 100 characterized by laminar flow and at Re = 3900 characterized by turbulent flow. It is shown that the proposed framework performs accurately and robustly in both prediction of sensor measurements and unsteady flow fields. Especially, the important physical features and quantities can be well maintained for both laminar and turbulent flow, even if high level noise is involved. Therefore, the proposed framework is a promising tool for sparse representation from complex flow fields in realistic experiments. (C)& nbsp;2022 Elsevier B.V. All rights reserved.
更多查看译文
关键词
Compressed sensing,Machine learning,Direct numerical simulation,Reduced-order model,Turbulent flow
AI 理解论文
溯源树
样例
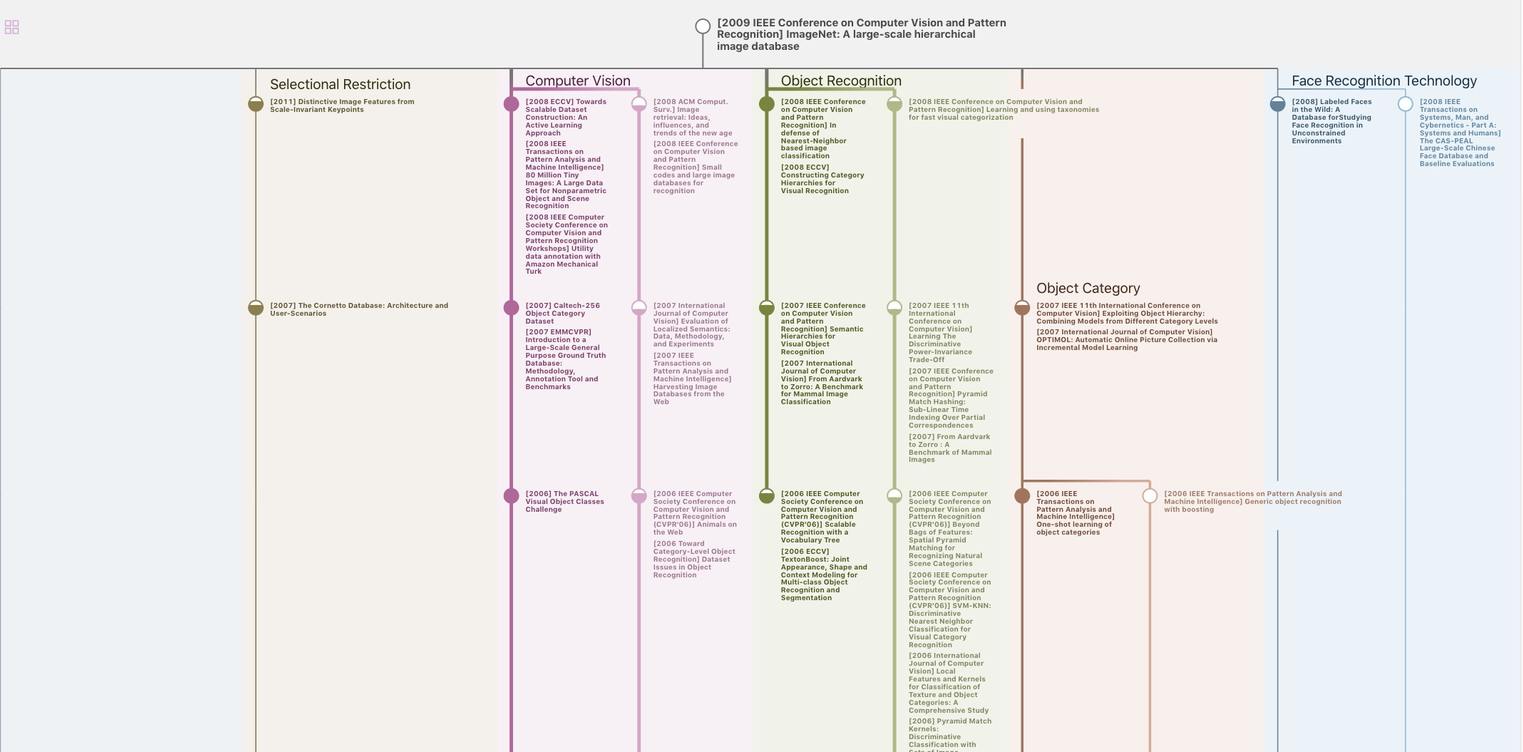
生成溯源树,研究论文发展脉络
Chat Paper
正在生成论文摘要