Iterative Learning for Heterogeneous Systems
IEEE/ASME transactions on mechatronics(2022)
摘要
Iterative learning control (ILC) aims to improve a system’s performance by learning from previous iterations in repetitive operations. Traditional ILC requires the system to operate in a strictly repetitive way and the learning process is within the confines of one single system. This article proposes an iterative learning method that extends traditional ILC from a temporal learning process within one single system to a spatial learning process between two heterogeneous systems. This learning mechanism is made viable by designing learning filters which bridge the dynamics of different systems. To design such learning filters, first, a dynamic system that consists of two heterogeneous systems and two learning filters is constructed; second, the two learning filters are decoupled from the known dynamics step by step. Each filter is successfully placed in the feedback loop of a reconstructed system consecutively, which enables us to transfer the filter design problem into a controller design problem that can be solved by leveraging the H-infinity optimal control theory. Meanwhile, the solvability of the learning filters, the stability of the system, and the learning convergence are rigorously analyzed. The proposed new algorithm is applied to the trajectory tracking problem of quadrotor drones. Both numerical and experimental studies have been conducted to validate the effectiveness of the proposed iterative learning method for multiple heterogeneous systems.
更多查看译文
关键词
Learning systems,Iterative methods,Dynamical systems,Tuning,Drones,Convergence,Trajectory,Heterogeneous systems,H-infinity optimal control,iterative learning
AI 理解论文
溯源树
样例
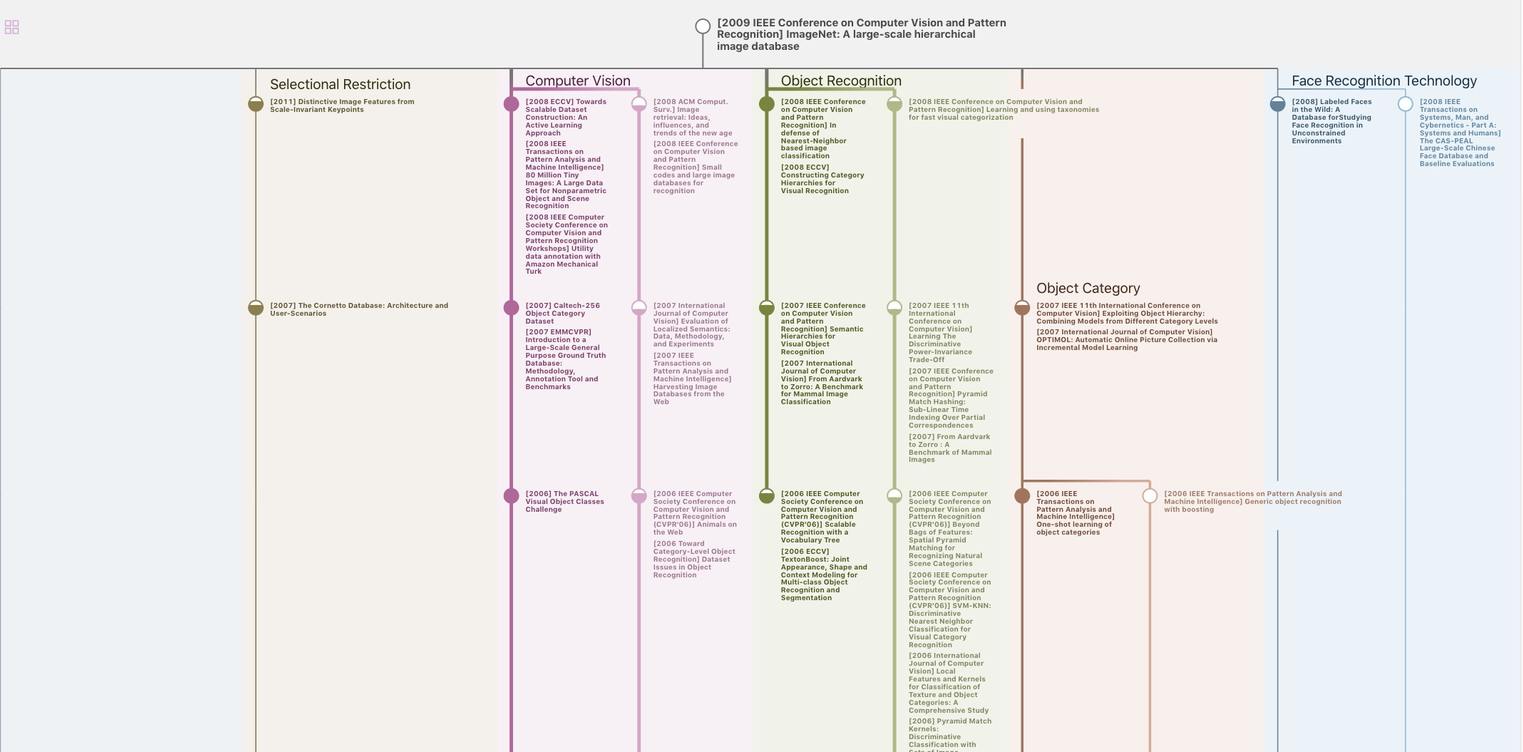
生成溯源树,研究论文发展脉络
Chat Paper
正在生成论文摘要