Deep Learning of Nonlinear Flame Fronts Development Due to Darrieus–Landau Instability
Social Science Research Network(2022)
摘要
The Darrieus–Landau instability is studied using a data-driven, deep neural network approach. The task is set up to learn a time-advancement operator mapping any given flame front to a future time. A recurrent application of such an operator rolls out a long sequence of predicted flame fronts, and a learned operator is required to not only make accurate short-term predictions but also reproduce characteristic nonlinear behavior, such as fractal front structures and detached flame pockets. Using two datasets of flame front solutions obtained from a heavy-duty direct numerical simulation and a light-duty modeling equation, we compare the performance of three state-of-art operator-regression network methods: convolutional neural networks, Fourier neural operator (FNO), and deep operator network. We show that, for learning complicated front evolution, FNO gives the best recurrent predictions in both the short and long term. A consistent extension allowing the operator-regression networks to handle complicated flame front shape is achieved by representing the latter as an implicit curve.
更多查看译文
关键词
Deep Learning,Nonlinear Systems,Model Reduction,Inverse Problems
AI 理解论文
溯源树
样例
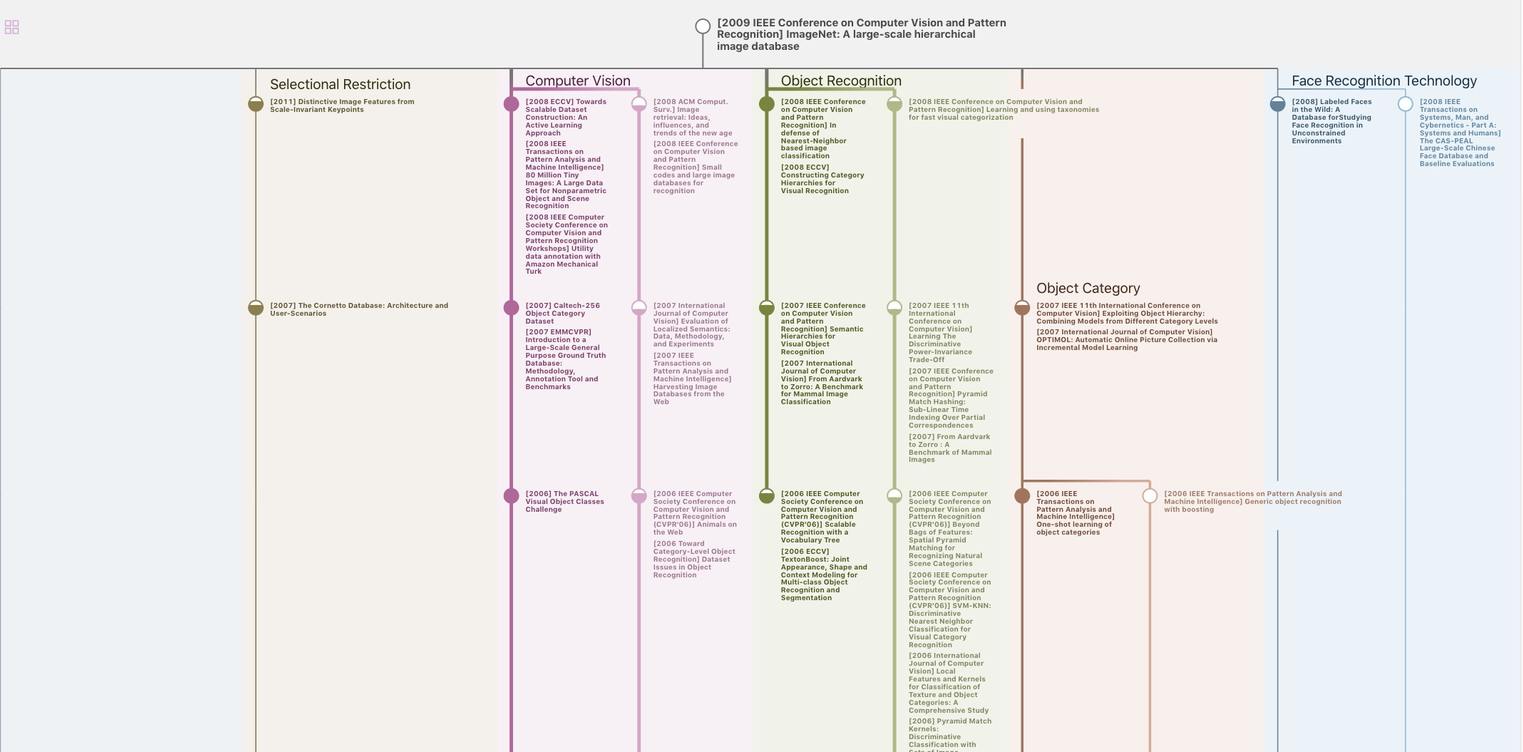
生成溯源树,研究论文发展脉络
Chat Paper
正在生成论文摘要