A Study on the Predictability of Sample Learning Consistency
arxiv(2022)
摘要
Curriculum Learning is a powerful training method that allows for faster and better training in some settings. This method, however, requires having a notion of which examples are difficult and which are easy, which is not always trivial to provide. A recent metric called C-Score acts as a proxy for example difficulty by relating it to learning consistency. Unfortunately, this method is quite compute intensive which limits its applicability for alternative datasets. In this work, we train models through different methods to predict C-Score for CIFAR-100 and CIFAR-10. We find, however, that these models generalize poorly both within the same distribution as well as out of distribution. This suggests that C-Score is not defined by the individual characteristics of each sample but rather by other factors. We hypothesize that a sample's relation to its neighbours, in particular, how many of them share the same labels, can help in explaining C-Scores. We plan to explore this in future work.
更多查看译文
关键词
sample learning consistency,predictability
AI 理解论文
溯源树
样例
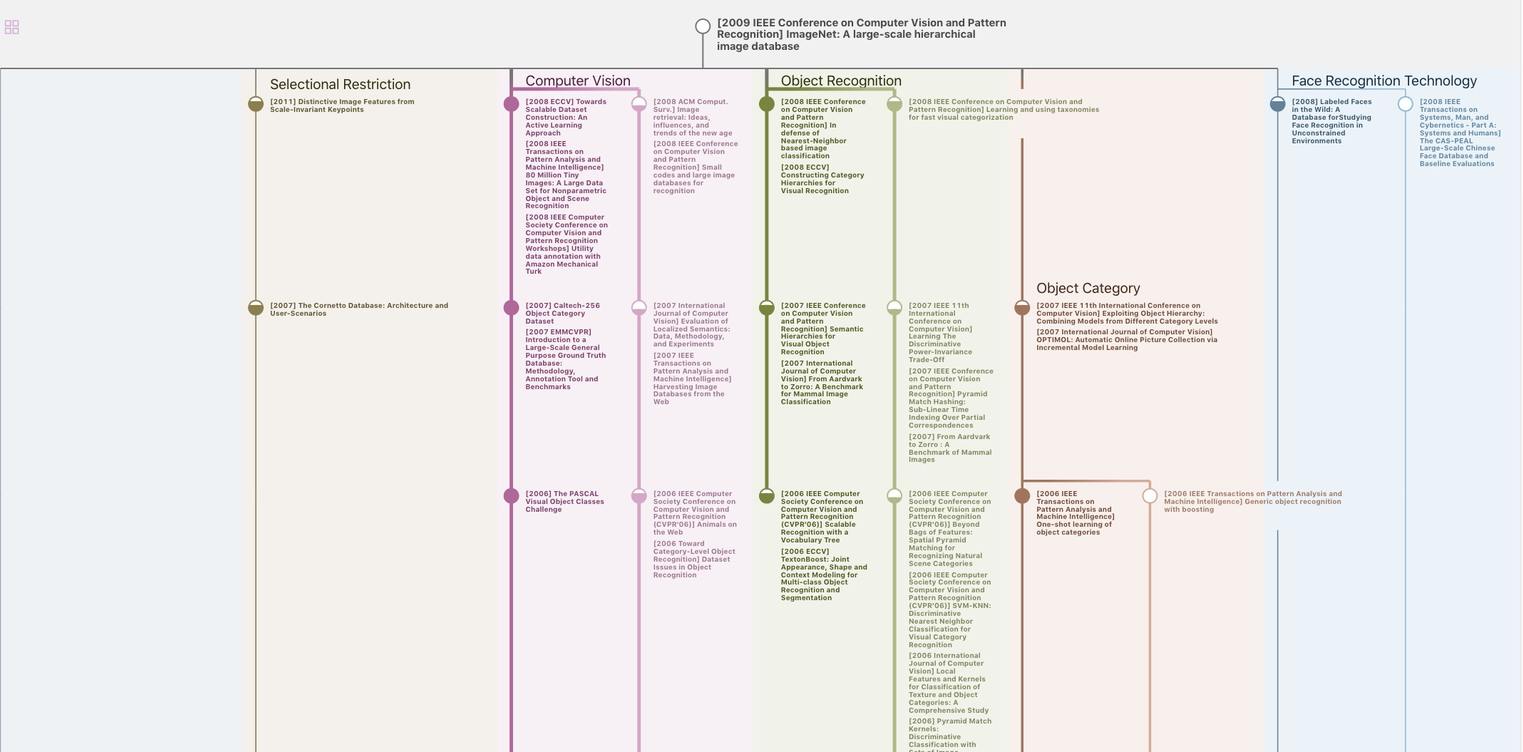
生成溯源树,研究论文发展脉络
Chat Paper
正在生成论文摘要