SPANet: Successive Pooling Attention Network for Semantic Segmentation of Remote Sensing Images
IEEE journal of selected topics in applied earth observations and remote sensing(2022)
摘要
In the convolutional neural network, the precise segmentation of small-scale objects and object boundaries in remote sensing images is a great challenge. As the model gets deeper, low-level features with geometric information and high-level features with semantic information cannot be obtained simultaneously. To alleviate this problem, a successive pooling attention network (SPANet) was proposed. The SPANet mainly consists of ResNet50 as the backbone, successive pooling attention module (SPAM), and feature fusion module (FFM). Specifically, the SPANet uses two parallel branches to extract high-level features by ResNet50 and low-level features by the first 11 layers of ResNet50. Then, both the high- and low-level features are fed to the SPAM, which is mainly composed of a successive pooling operator and a self-attention submodule, for further extracting deeper multiscale and salient features. In addition, the low- and high-level features after the SPAM are fused by the FFM to achieve the complementarity of spatial and geometric information. This fusion module alleviates the problem of the accurate segmentation of object edges. Finally, the high-level features and enhanced low-level features of the two branches are fused to obtain the final prediction results. Experiments show that the proposed SPANet achieves a good segmentation effect compared with other models on two remotely sensed datasets.
更多查看译文
关键词
Attention mechanism,convolutional neural network,remote sensing images,semantic segmentation,successive pooling
AI 理解论文
溯源树
样例
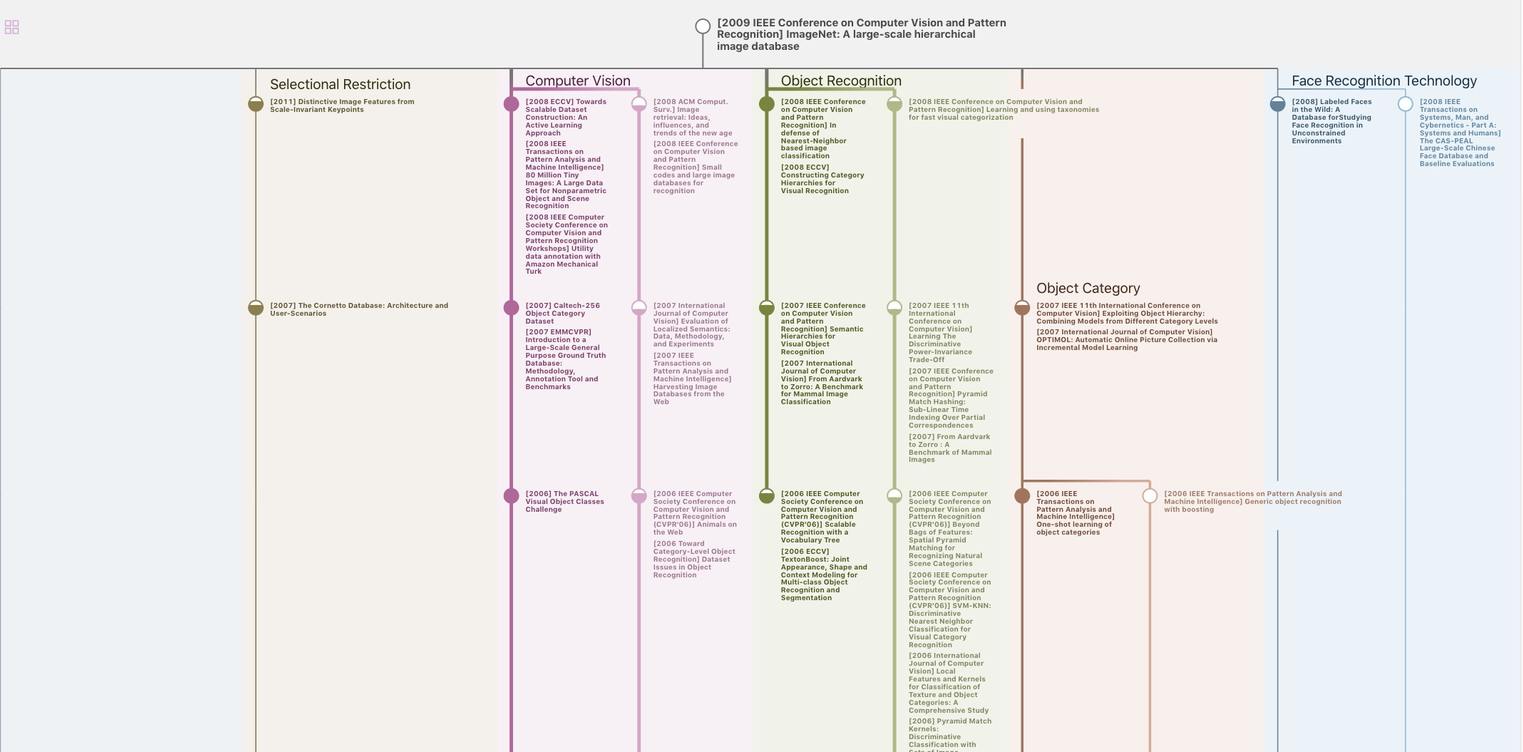
生成溯源树,研究论文发展脉络
Chat Paper
正在生成论文摘要