Interpretation Horizon Adjustments Using Machine Learning During Iterative Model Updates: a Case Study from Offshore Gabon
83rd EAGE Annual Conference & Exhibition(2022)
Abstract
Summary In this paper we present a machine-learning (ML) assisted interpretation workflow that aids in horizon adjustment during model updates. The ML workflow predicts the position of the new horizon on the updated seismic volume by utilizing previous horizons and its associated legacy seismic volume as input training data. This ML prediction capability significantly advanced the efficiency of model building by minimizing the time-intensive manual adjustments of horizon interpretation during each earth model update iteration. Moreover, the ML-assisted horizon delineates high-resolution surface details, consistently tracks the geologic events, and improves connectivity in each updated model. The quality of the ML-assisted horizon is superior to standard production horizons generated within condensed project timelines. More impressively, the ML-assisted interpretation workflow improves turnaround time efficiency by 85% for each interpretation adjust of the top of salt horizon on a complex Gabon offshore dataset.
MoreTranslated text
AI Read Science
Must-Reading Tree
Example
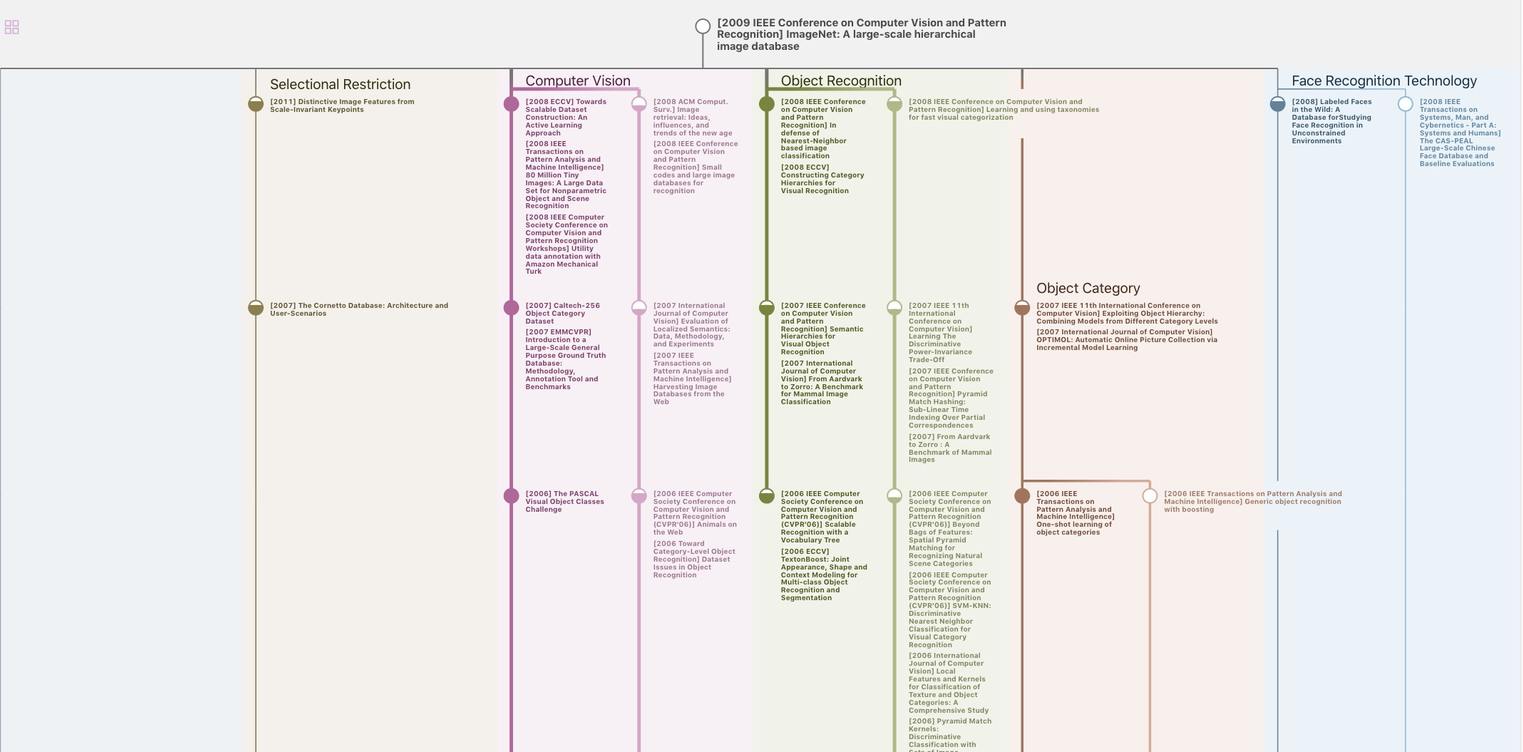
Generate MRT to find the research sequence of this paper
Chat Paper
Summary is being generated by the instructions you defined