Bucket Detector Signal Denoising for Low-Light-Level Single-Pixel Imaging Based on Denoising Convolution Neural Network
Social Science Research Network(2022)
摘要
Single-pixel imaging (SPI) systems usually require a long integration time to obtain high-quality images in a low-light-level (LLL) environment, seriously affecting the imaging efficiency. Conversely, if the exposure time is shortened, the signal can be drowned in noise. A denoising convolution neural network (DnCNN) is a powerful tool for reducing the noise of bucket detectors. However, the generation of noisy signals for training such networks has not been studied in depth. In this study, we investigated the noise characteristics to obtain a real noise model, generated a series of simulated noisy signals based on the real noise model to train a DnCNN, and tested the network using real experimental data. Experiments showed that this method can effectively reduce the noise of the bucket detector signal and improve the quality of the formed image. Furthermore, we showed the factors affecting the performance of our DnCNN in the given system. The proposed method opens up a new way to obtain noisy datasets at low cost, and represents a significant step toward applying deep learning to SPI.
更多查看译文
AI 理解论文
溯源树
样例
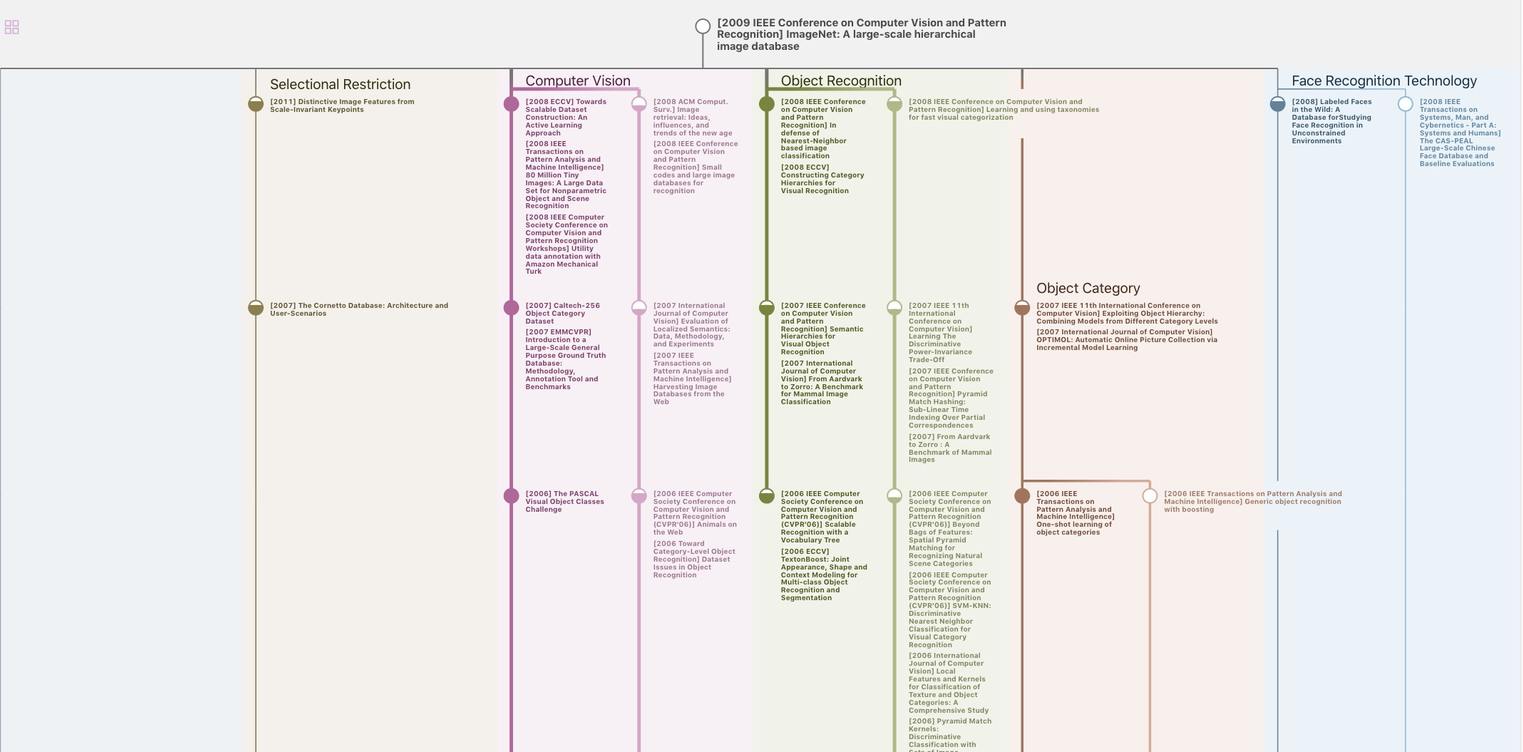
生成溯源树,研究论文发展脉络
Chat Paper
正在生成论文摘要