Local Correlation Ensemble with GCN Based on Attention Features for Cross-domain Person Re-ID
ACM Transactions on Multimedia Computing, Communications, and Applications(2023)
摘要
Person re-identification (Re-ID) has achieved great success in single-domain. However, it remains a challenging task to adapt a Re-ID model trained on one dataset to another one. Unsupervised domain adaption (UDA) was proposed to migrate a model from a labeled source domain to an unlabeled target domain. The main difference in the cross-domain is different background styles. Although the style transfer approach effectively reduces inter-domain gaps, it ignores the reduction of intra-class differences. Clustering-based pipelinesmaintain state-of-the-art performance for UDA by learning domain-independent features; however, most existing models do not sufficiently exploit the rich unlabeled samples in target domains due to unsatisfactory clustering. Thus, we propose a novel local correlation ensemble model that focuses on the diversity of intra-class information and the reliability of class centers. Specifically, a pedestrian attention module is proposed to enable the encoder to pay more attention to the person's features to relieve interference caused by the shared background style. Furthermore, we propose a priority-distance graph convolutional network (PDGCN) module that employs a graph convolutional network network to predict the priority of a node as a class center and then calculates the distance between nodes with high priority values to screen out the class center nodes. Finally, the encoder features ( local) and PDGCN features (context-aware) are combined to perform person Re-ID. The results of experiments on the large-scale public Re-ID datasets verified the effectiveness of the proposed method.
更多查看译文
关键词
Cross-domain,cluster,person Re-ID,GCN,attention
AI 理解论文
溯源树
样例
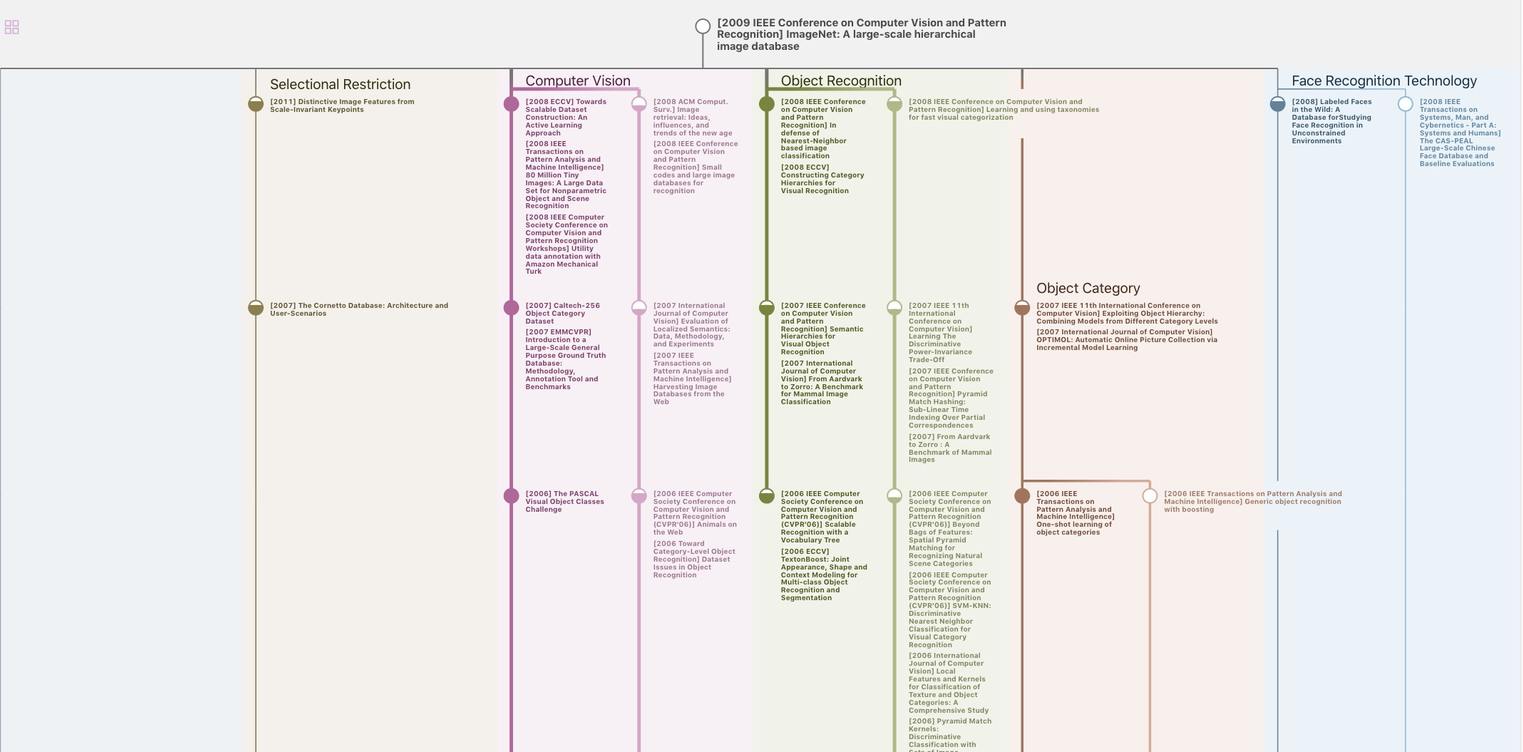
生成溯源树,研究论文发展脉络
Chat Paper
正在生成论文摘要