Precise and Fast Segmentation of Offshore Farms in High-Resolution SAR Images Based on Model Fusion and Half-Precision Parallel Inference
IEEE JOURNAL OF SELECTED TOPICS IN APPLIED EARTH OBSERVATIONS AND REMOTE SENSING(2022)
Abstract
In aquaculture, using high-resolution synthetic aperture radar (SAR) images to precisely segment offshore farms is helpful for reasonable layout planning and statistics of breeding density. However, conventional segmentation methods tend to have low accuracy and slow inference speed. Therefore, we propose a novel, precise, and fast segmentation scheme for offshore farms in high-resolution SAR images based on model fusion and half-precision parallel inference. Specifically, we propose several new high-performance improved UNet++ methods and reasonably fuse the test results. At the same time, a simulated annealing strategy and a morphological closing operation are introduced to improve the segmentation accuracy. In addition, we find that resizing the images to 256 x 256 pixels is better than 512 x 512 pixels for this task, which not only has higher segmentation accuracy but can also increase the inference speed by nearly 13%. Furthermore, a novel half-precision parallel inference strategy is proposed, which can fully utilize the GPU and increase the inference speed by 72.6%. Compared with some state-of-the-art methods, the proposed scheme that merges two improved UNet++ achieves superior accuracy with a frequency weighted intersection over union of 0.9876 and a single image inference time of 0.0218 s on the high-resolution SAR offshore farm dataset.
MoreTranslated text
Key words
Image segmentation,Feature extraction,Task analysis,Radar polarimetry,Licenses,Simulated annealing,Data mining,Half-precision parallel inference,improved UNET plus plus,SAR images,segmentation,simulated annealing
AI Read Science
Must-Reading Tree
Example
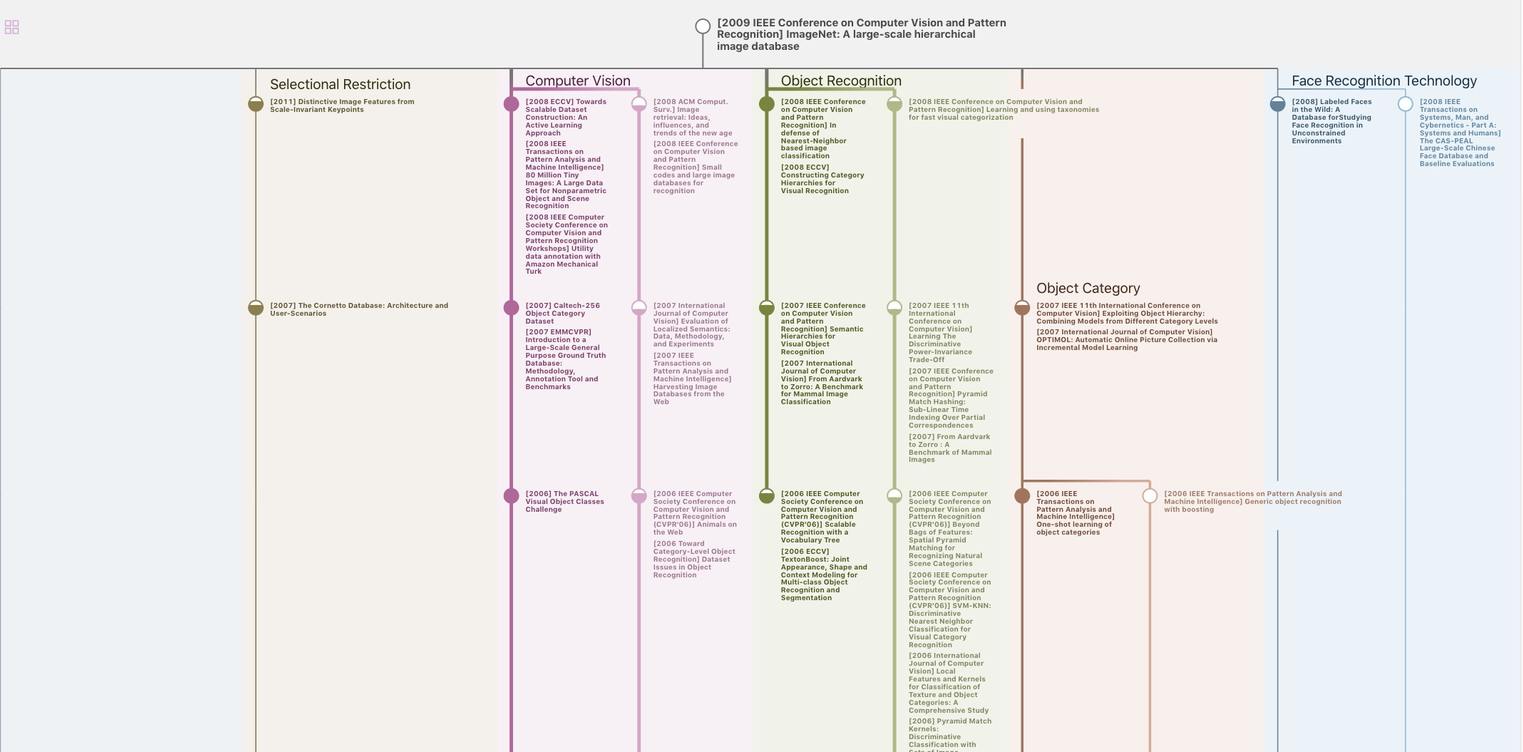
Generate MRT to find the research sequence of this paper
Chat Paper
Summary is being generated by the instructions you defined